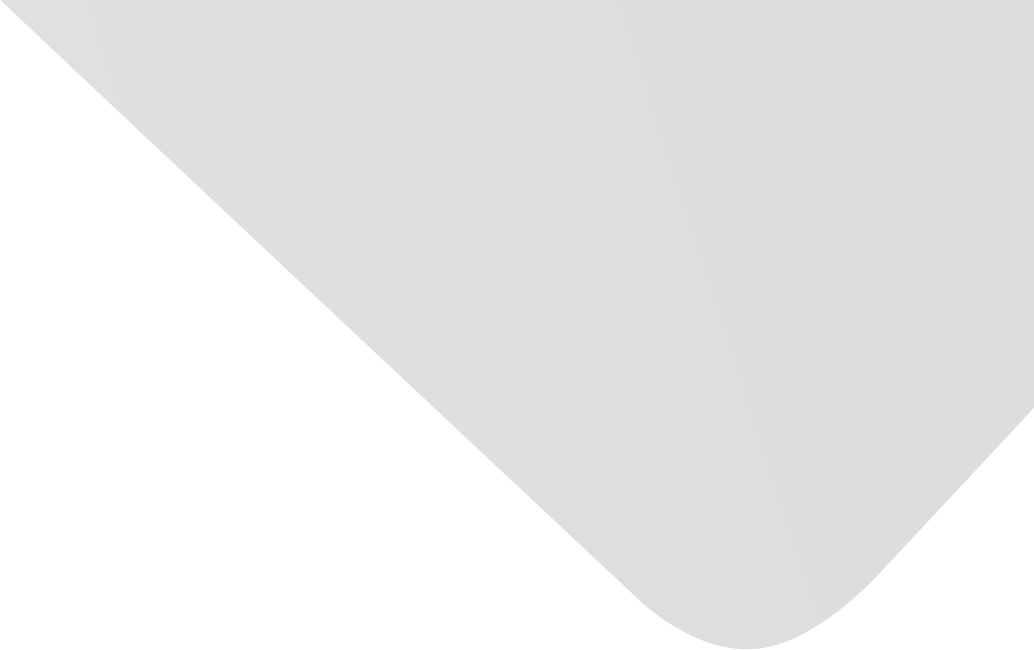
Speed Distribution Prediction of Freight Vehicles on Mountainous Freeway Using Deep Learning Methods
المؤلفون المشاركون
المصدر
Journal of Advanced Transportation
العدد
المجلد 2020، العدد 2020 (31 ديسمبر/كانون الأول 2020)، ص ص. 1-14، 14ص.
الناشر
Hindawi Publishing Corporation
تاريخ النشر
2020-01-10
دولة النشر
مصر
عدد الصفحات
14
التخصصات الرئيسية
الملخص EN
Driving speed is one of the most critical indicators in safety evaluation and network monitoring in freight transportation.
Speed prediction model serves as the most efficient method to obtain the data of driving speed.
Current speed prediction models mostly focus on operating speed, which is hard to reveal the overall condition of driving speed on the road section.
Meanwhile, the models were mostly developed based on the regression method, which is inconsistent with natural driving process.
Recurrent neural network (RNN) is a distinctive type of deep learning method to capture the temporary dependency in behavioral research.
The aim of this paper is to apply the deep learning method to predict the general condition of driving speed in consideration of the road geometry and the temporal evolutions.
3D mobile mapping was applied to obtain road geometry information with high precision, and driving simulation experiment was then conducted with the help of the road geometry data.
Driving speed was characterized by the bimodal Gauss mixture model.
RNN and its variants including long short-term memory (LSTM) and RNN and gated recurrent units (GRUs) were utilized to predict speed distribution in a spatial-temporal dimension with KL divergence being the loss function.
The result proved the applicability of the model in speed distribution prediction of freight vehicles, while LSTM holds the best performance with the length of input sequence being 400 m.
The result can be related to the threshold of drivers’ information processing on mountainous freeway.
Multiple linear regression models were constructed to be a contrast with the LSTM model, and the results showed that LSTM was superior to regression models in terms of the model accuracy and interpretability of the driving process and the formation of vehicle speed.
This study may help to understand speed change behavior of freight vehicles on mountainous freeways, while providing the feasible method for safety evaluation or network efficiency analysis.
نمط استشهاد جمعية علماء النفس الأمريكية (APA)
Chen, Yuren& Chen, Yu& Yu, Bo. 2020. Speed Distribution Prediction of Freight Vehicles on Mountainous Freeway Using Deep Learning Methods. Journal of Advanced Transportation،Vol. 2020, no. 2020, pp.1-14.
https://search.emarefa.net/detail/BIM-1181041
نمط استشهاد الجمعية الأمريكية للغات الحديثة (MLA)
Chen, Yuren…[et al.]. Speed Distribution Prediction of Freight Vehicles on Mountainous Freeway Using Deep Learning Methods. Journal of Advanced Transportation No. 2020 (2020), pp.1-14.
https://search.emarefa.net/detail/BIM-1181041
نمط استشهاد الجمعية الطبية الأمريكية (AMA)
Chen, Yuren& Chen, Yu& Yu, Bo. Speed Distribution Prediction of Freight Vehicles on Mountainous Freeway Using Deep Learning Methods. Journal of Advanced Transportation. 2020. Vol. 2020, no. 2020, pp.1-14.
https://search.emarefa.net/detail/BIM-1181041
نوع البيانات
مقالات
لغة النص
الإنجليزية
الملاحظات
Includes bibliographical references
رقم السجل
BIM-1181041
قاعدة معامل التأثير والاستشهادات المرجعية العربي "ارسيف Arcif"
أضخم قاعدة بيانات عربية للاستشهادات المرجعية للمجلات العلمية المحكمة الصادرة في العالم العربي
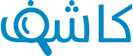
تقوم هذه الخدمة بالتحقق من التشابه أو الانتحال في الأبحاث والمقالات العلمية والأطروحات الجامعية والكتب والأبحاث باللغة العربية، وتحديد درجة التشابه أو أصالة الأعمال البحثية وحماية ملكيتها الفكرية. تعرف اكثر
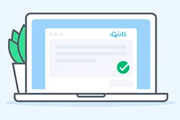