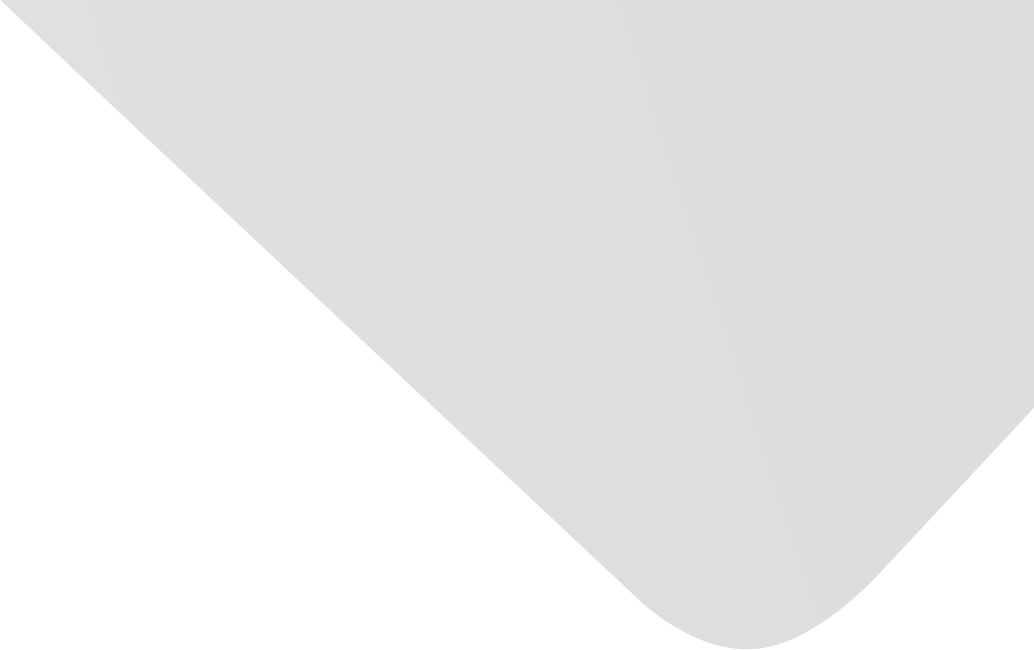
Evaluation of Short-Term Freeway Speed Prediction Based on Periodic Analysis Using Statistical Models and Machine Learning Models
المؤلفون المشاركون
Zou, Yajie
Tang, Jinjun
Yang, Xiaoxue
Ijaz, Muhammad
Liang, Jian
المصدر
Journal of Advanced Transportation
العدد
المجلد 2020، العدد 2020 (31 ديسمبر/كانون الأول 2020)، ص ص. 1-16، 16ص.
الناشر
Hindawi Publishing Corporation
تاريخ النشر
2020-01-20
دولة النشر
مصر
عدد الصفحات
16
التخصصات الرئيسية
الملخص EN
Accurate prediction of traffic information (i.e., traffic flow, travel time, traffic speed, etc.) is a key component of Intelligent Transportation System (ITS).
Traffic speed is an important indicator to evaluate traffic efficiency.
Up to date, although a few studies have considered the periodic feature in traffic prediction, very few studies comprehensively evaluate the impact of periodic component on statistical and machine learning prediction models.
This paper selects several representative statistical models and machine learning models to analyze the influence of periodic component on short-term speed prediction under different scenarios: (1) multi-horizon ahead prediction (5, 15, 30, 60 minutes ahead predictions), (2) with and without periodic component, (3) two data aggregation levels (5-minute and 15-minute), (4) peak hours and off-peak hours.
Specifically, three statistical models (i.e., space time (ST) model, vector autoregressive (VAR) model, autoregressive integrated moving average (ARIMA) model) and three machine learning approaches (i.e., support vector machines (SVM) model, multi-layer perceptron (MLP) model, recurrent neural network (RNN) model) are developed and examined.
Furthermore, the periodic features of the speed data are considered via a hybrid prediction method, which assumes that the data consist of two components: a periodic component and a residual component.
The periodic component is described by a trigonometric regression function, and the residual component is modeled by the statistical models or the machine learning approaches.
The important conclusions can be summarized as follows: (1) the multi-step ahead prediction accuracy improves when considering the periodic component of speed data for both three statistical models and three machine learning models, especially in the peak hours; (2) considering the impact of periodic component for all models, the prediction performance improvement gradually becomes larger as the time step increases; (3) under the same prediction horizon, the prediction performance of all models for 15-minute speed data is generally better than that for 5-minute speed data.
Overall, the findings in this paper suggest that the proposed hybrid prediction approach is effective for both statistical and machine learning models in short-term speed prediction.
نمط استشهاد جمعية علماء النفس الأمريكية (APA)
Yang, Xiaoxue& Zou, Yajie& Tang, Jinjun& Liang, Jian& Ijaz, Muhammad. 2020. Evaluation of Short-Term Freeway Speed Prediction Based on Periodic Analysis Using Statistical Models and Machine Learning Models. Journal of Advanced Transportation،Vol. 2020, no. 2020, pp.1-16.
https://search.emarefa.net/detail/BIM-1181111
نمط استشهاد الجمعية الأمريكية للغات الحديثة (MLA)
Yang, Xiaoxue…[et al.]. Evaluation of Short-Term Freeway Speed Prediction Based on Periodic Analysis Using Statistical Models and Machine Learning Models. Journal of Advanced Transportation No. 2020 (2020), pp.1-16.
https://search.emarefa.net/detail/BIM-1181111
نمط استشهاد الجمعية الطبية الأمريكية (AMA)
Yang, Xiaoxue& Zou, Yajie& Tang, Jinjun& Liang, Jian& Ijaz, Muhammad. Evaluation of Short-Term Freeway Speed Prediction Based on Periodic Analysis Using Statistical Models and Machine Learning Models. Journal of Advanced Transportation. 2020. Vol. 2020, no. 2020, pp.1-16.
https://search.emarefa.net/detail/BIM-1181111
نوع البيانات
مقالات
لغة النص
الإنجليزية
الملاحظات
Includes bibliographical references
رقم السجل
BIM-1181111
قاعدة معامل التأثير والاستشهادات المرجعية العربي "ارسيف Arcif"
أضخم قاعدة بيانات عربية للاستشهادات المرجعية للمجلات العلمية المحكمة الصادرة في العالم العربي
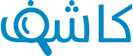
تقوم هذه الخدمة بالتحقق من التشابه أو الانتحال في الأبحاث والمقالات العلمية والأطروحات الجامعية والكتب والأبحاث باللغة العربية، وتحديد درجة التشابه أو أصالة الأعمال البحثية وحماية ملكيتها الفكرية. تعرف اكثر
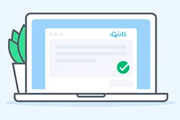