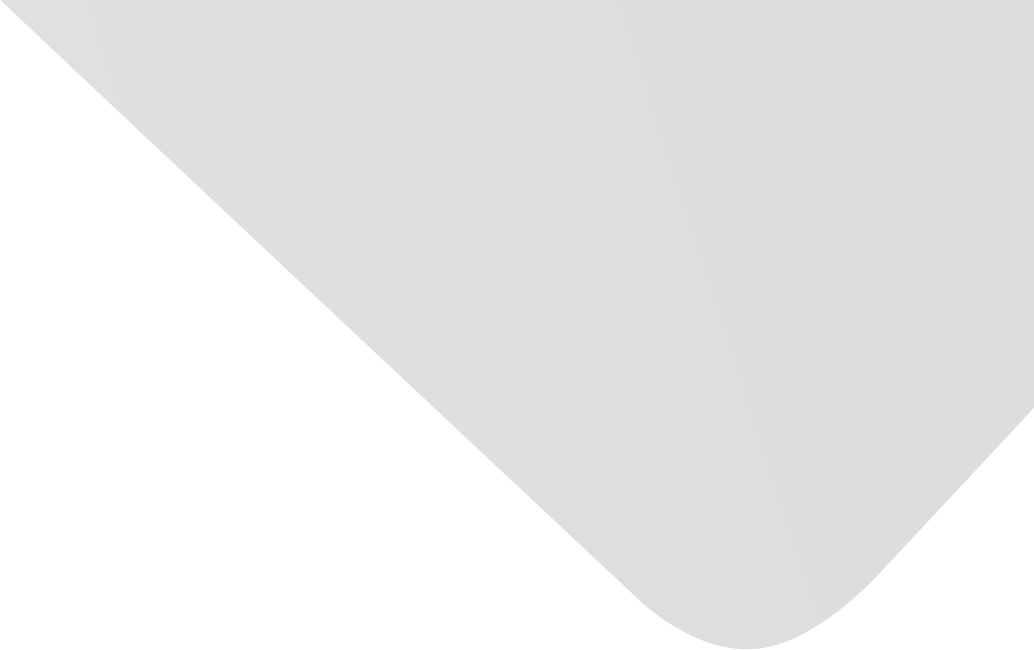
An Accurate PSO-GA Based Neural Network to Model Growth of Carbon Nanotubes
المؤلفون المشاركون
Asadnia, Mohsen
Khorasani, Amir Mahyar
Warkiani, Majid Ebrahimi
المصدر
العدد
المجلد 2017، العدد 2017 (31 ديسمبر/كانون الأول 2017)، ص ص. 1-6، 6ص.
الناشر
Hindawi Publishing Corporation
تاريخ النشر
2017-09-06
دولة النشر
مصر
عدد الصفحات
6
التخصصات الرئيسية
الملخص EN
By combining particle swarm optimization (PSO) and genetic algorithms (GA) this paper offers an innovative algorithm to train artificial neural networks (ANNs) for the purpose of calculating the experimental growth parameters of CNTs.
The paper explores experimentally obtaining data to train ANNs, as a method to reduce simulation time while ensuring the precision of formal physics models.
The results are compared with conventional particle swarm optimization based neural network (CPSONN) and Levenberg–Marquardt (LM) techniques.
The results show that PSOGANN can be successfully utilized for modeling the experimental parameters that are critical for the growth of CNTs.
نمط استشهاد جمعية علماء النفس الأمريكية (APA)
Asadnia, Mohsen& Khorasani, Amir Mahyar& Warkiani, Majid Ebrahimi. 2017. An Accurate PSO-GA Based Neural Network to Model Growth of Carbon Nanotubes. Journal of Nanomaterials،Vol. 2017, no. 2017, pp.1-6.
https://search.emarefa.net/detail/BIM-1183866
نمط استشهاد الجمعية الأمريكية للغات الحديثة (MLA)
Asadnia, Mohsen…[et al.]. An Accurate PSO-GA Based Neural Network to Model Growth of Carbon Nanotubes. Journal of Nanomaterials No. 2017 (2017), pp.1-6.
https://search.emarefa.net/detail/BIM-1183866
نمط استشهاد الجمعية الطبية الأمريكية (AMA)
Asadnia, Mohsen& Khorasani, Amir Mahyar& Warkiani, Majid Ebrahimi. An Accurate PSO-GA Based Neural Network to Model Growth of Carbon Nanotubes. Journal of Nanomaterials. 2017. Vol. 2017, no. 2017, pp.1-6.
https://search.emarefa.net/detail/BIM-1183866
نوع البيانات
مقالات
لغة النص
الإنجليزية
الملاحظات
Includes bibliographical references
رقم السجل
BIM-1183866
قاعدة معامل التأثير والاستشهادات المرجعية العربي "ارسيف Arcif"
أضخم قاعدة بيانات عربية للاستشهادات المرجعية للمجلات العلمية المحكمة الصادرة في العالم العربي
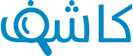
تقوم هذه الخدمة بالتحقق من التشابه أو الانتحال في الأبحاث والمقالات العلمية والأطروحات الجامعية والكتب والأبحاث باللغة العربية، وتحديد درجة التشابه أو أصالة الأعمال البحثية وحماية ملكيتها الفكرية. تعرف اكثر
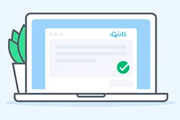