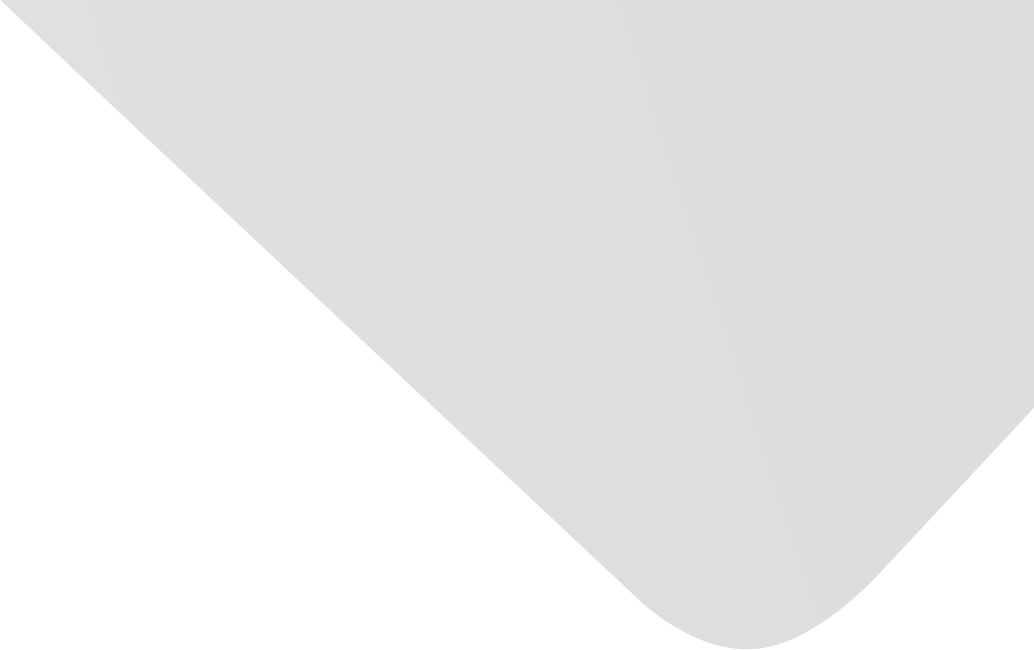
SS-SWT and SI-CNN: An Atrial Fibrillation Detection Framework for Time-Frequency ECG Signal
المؤلفون المشاركون
Dai, Honghua
Wang, Zongmin
He, Renke
Zhang, Hongpo
Xu, Mingliang
المصدر
Journal of Healthcare Engineering
العدد
المجلد 2020، العدد 2020 (31 ديسمبر/كانون الأول 2020)، ص ص. 1-11، 11ص.
الناشر
Hindawi Publishing Corporation
تاريخ النشر
2020-05-18
دولة النشر
مصر
عدد الصفحات
11
التخصصات الرئيسية
الملخص EN
Atrial fibrillation is the most common arrhythmia and is associated with high morbidity and mortality from stroke, heart failure, myocardial infarction, and cerebral thrombosis.
Effective and rapid detection of atrial fibrillation is critical to reducing morbidity and mortality in patients.
Screening atrial fibrillation quickly and efficiently remains a challenging task.
In this paper, we propose SS-SWT and SI-CNN: an atrial fibrillation detection framework for the time-frequency ECG signal.
First, specific-scale stationary wavelet transform (SS-SWT) is used to decompose a 5-s ECG signal into 8 scales.
We select specific scales of coefficients as valid time-frequency features and abandon the other coefficients.
The selected coefficients are fed to the scale-independent convolutional neural network (SI-CNN) as a two-dimensional (2D) matrix.
In SI-CNN, a convolution kernel specifically for the time-frequency characteristics of ECG signals is designed.
During the convolution process, the independence between each scale of coefficient is preserved, and the time domain and the frequency domain characteristics of the ECG signal are effectively extracted, and finally the atrial fibrillation signal is quickly and accurately identified.
In this study, experiments are performed using the MIT-BIH AFDB data in 5-s data segments.
We achieve 99.03% sensitivity, 99.35% specificity, and 99.23% overall accuracy.
The SS-SWT and SI-CNN we propose simplify the feature extraction step, effectively extracts the features of ECG, and reduces the feature redundancy that may be caused by wavelet transform.
The results shows that the method can effectively detect atrial fibrillation signals and has potential in clinical application.
نمط استشهاد جمعية علماء النفس الأمريكية (APA)
Zhang, Hongpo& He, Renke& Dai, Honghua& Xu, Mingliang& Wang, Zongmin. 2020. SS-SWT and SI-CNN: An Atrial Fibrillation Detection Framework for Time-Frequency ECG Signal. Journal of Healthcare Engineering،Vol. 2020, no. 2020, pp.1-11.
https://search.emarefa.net/detail/BIM-1186379
نمط استشهاد الجمعية الأمريكية للغات الحديثة (MLA)
Zhang, Hongpo…[et al.]. SS-SWT and SI-CNN: An Atrial Fibrillation Detection Framework for Time-Frequency ECG Signal. Journal of Healthcare Engineering No. 2020 (2020), pp.1-11.
https://search.emarefa.net/detail/BIM-1186379
نمط استشهاد الجمعية الطبية الأمريكية (AMA)
Zhang, Hongpo& He, Renke& Dai, Honghua& Xu, Mingliang& Wang, Zongmin. SS-SWT and SI-CNN: An Atrial Fibrillation Detection Framework for Time-Frequency ECG Signal. Journal of Healthcare Engineering. 2020. Vol. 2020, no. 2020, pp.1-11.
https://search.emarefa.net/detail/BIM-1186379
نوع البيانات
مقالات
لغة النص
الإنجليزية
الملاحظات
Includes bibliographical references
رقم السجل
BIM-1186379
قاعدة معامل التأثير والاستشهادات المرجعية العربي "ارسيف Arcif"
أضخم قاعدة بيانات عربية للاستشهادات المرجعية للمجلات العلمية المحكمة الصادرة في العالم العربي

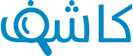
تقوم هذه الخدمة بالتحقق من التشابه أو الانتحال في الأبحاث والمقالات العلمية والأطروحات الجامعية والكتب والأبحاث باللغة العربية، وتحديد درجة التشابه أو أصالة الأعمال البحثية وحماية ملكيتها الفكرية. تعرف اكثر
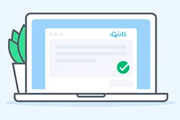