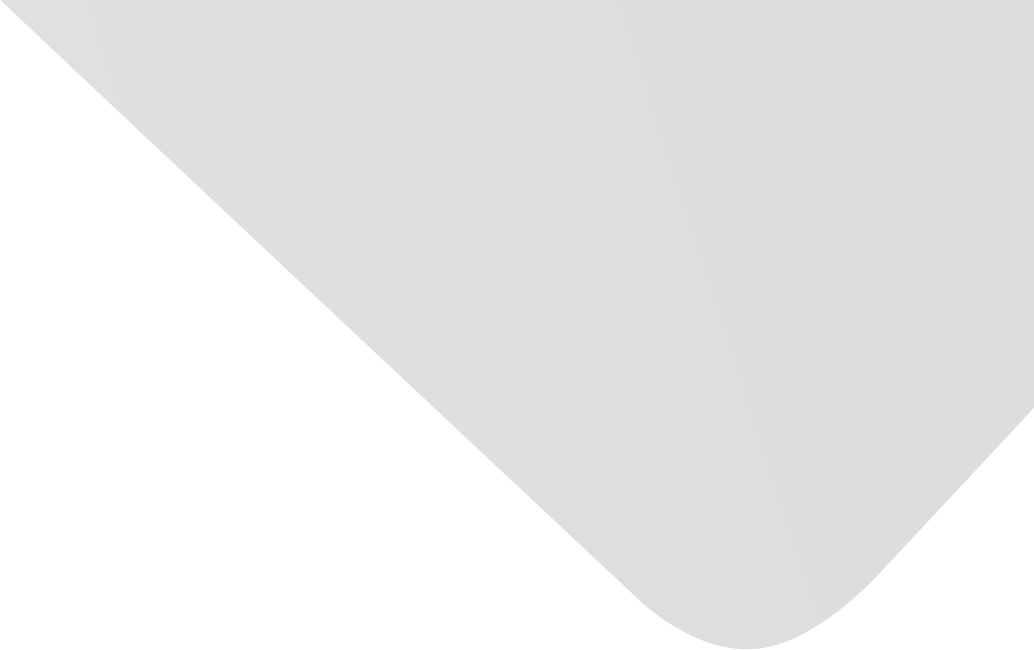
Reconstruct the Support Vectors to Improve LSSVM Sparseness for Mill Load Prediction
المؤلفون المشاركون
Si, Gangquan
Zhang, Yanbin
Shi, Jianquan
Guo, Zhang
Jia, Lixin
المصدر
Mathematical Problems in Engineering
العدد
المجلد 2017، العدد 2017 (31 ديسمبر/كانون الأول 2017)، ص ص. 1-12، 12ص.
الناشر
Hindawi Publishing Corporation
تاريخ النشر
2017-07-05
دولة النشر
مصر
عدد الصفحات
12
التخصصات الرئيسية
الملخص EN
The sparse strategy plays a significant role in the application of the least square support vector machine (LSSVM), to alleviate the condition that the solution of LSSVM is lacking sparseness and robustness.
In this paper, a sparse method using reconstructed support vectors is proposed, which has also been successfully applied to mill load prediction.
Different from other sparse algorithms, it no longer selects the support vectors from training data set according to the ranked contributions for optimization of LSSVM.
Instead, the reconstructed data is obtained first based on the initial model with all training data.
Then, select support vectors from reconstructed data set according to the location information of density clustering in training data set, and the process of selecting is terminated after traversing the total training data set.
Finally, the training model could be built based on the optimal reconstructed support vectors and the hyperparameter tuned subsequently.
What is more, the paper puts forward a supplemental algorithm to subtract the redundancy support vectors of previous model.
Lots of experiments on synthetic data sets, benchmark data sets, and mill load data sets are carried out, and the results illustrate the effectiveness of the proposed sparse method for LSSVM.
نمط استشهاد جمعية علماء النفس الأمريكية (APA)
Si, Gangquan& Shi, Jianquan& Guo, Zhang& Jia, Lixin& Zhang, Yanbin. 2017. Reconstruct the Support Vectors to Improve LSSVM Sparseness for Mill Load Prediction. Mathematical Problems in Engineering،Vol. 2017, no. 2017, pp.1-12.
https://search.emarefa.net/detail/BIM-1190359
نمط استشهاد الجمعية الأمريكية للغات الحديثة (MLA)
Si, Gangquan…[et al.]. Reconstruct the Support Vectors to Improve LSSVM Sparseness for Mill Load Prediction. Mathematical Problems in Engineering No. 2017 (2017), pp.1-12.
https://search.emarefa.net/detail/BIM-1190359
نمط استشهاد الجمعية الطبية الأمريكية (AMA)
Si, Gangquan& Shi, Jianquan& Guo, Zhang& Jia, Lixin& Zhang, Yanbin. Reconstruct the Support Vectors to Improve LSSVM Sparseness for Mill Load Prediction. Mathematical Problems in Engineering. 2017. Vol. 2017, no. 2017, pp.1-12.
https://search.emarefa.net/detail/BIM-1190359
نوع البيانات
مقالات
لغة النص
الإنجليزية
الملاحظات
Includes bibliographical references
رقم السجل
BIM-1190359
قاعدة معامل التأثير والاستشهادات المرجعية العربي "ارسيف Arcif"
أضخم قاعدة بيانات عربية للاستشهادات المرجعية للمجلات العلمية المحكمة الصادرة في العالم العربي
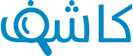
تقوم هذه الخدمة بالتحقق من التشابه أو الانتحال في الأبحاث والمقالات العلمية والأطروحات الجامعية والكتب والأبحاث باللغة العربية، وتحديد درجة التشابه أو أصالة الأعمال البحثية وحماية ملكيتها الفكرية. تعرف اكثر
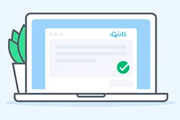