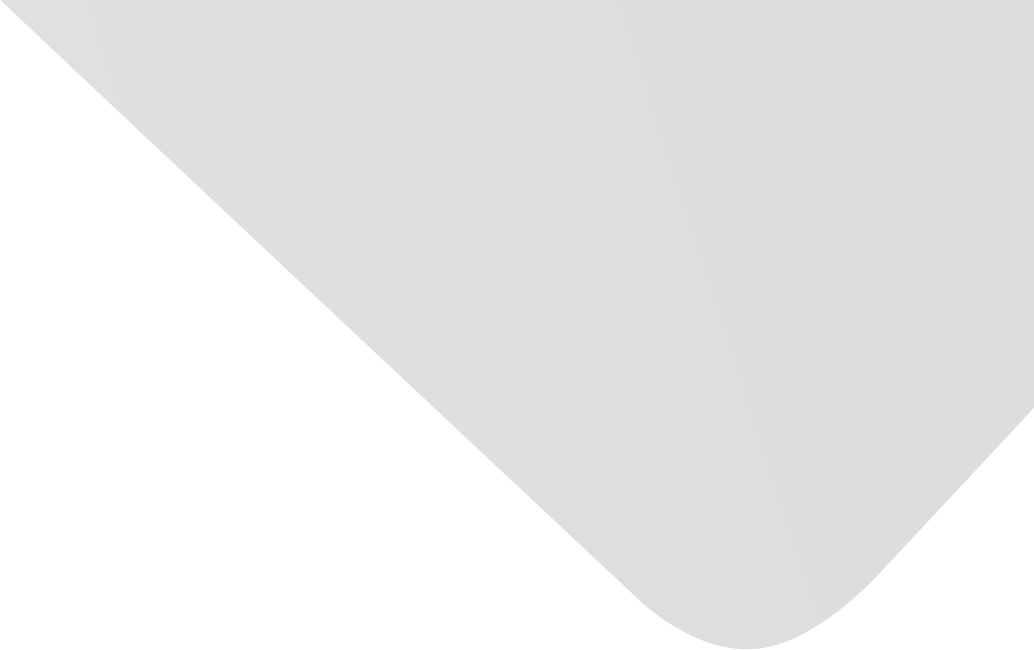
Design and Assessment of a Robust and Generalizable ANN-Based Classifier for the Prediction of Premature Birth by means of Multichannel Electrohysterographic Records
المؤلفون المشاركون
Mas-Cabo, J.
Alberola-Rubio, J.
Ye-Lin, Y.
Garcia-Casado, Javier
Prats-Boluda, Gema
Perales-Marín, Alfredo
المصدر
العدد
المجلد 2019، العدد 2019 (31 ديسمبر/كانون الأول 2019)، ص ص. 1-13، 13ص.
الناشر
Hindawi Publishing Corporation
تاريخ النشر
2019-11-25
دولة النشر
مصر
عدد الصفحات
13
التخصصات الرئيسية
الملخص EN
Preterm labor is one of the major causes of neonatal deaths and also the cause of significant health and development impairments in those who survive.
However, there are still no reliable and accurate tools for preterm labor prediction in clinical settings.
Electrohysterography (EHG) has been proven to provide relevant information on the labor time horizon.
Many studies focused on predicting preterm labor by using temporal, spectral, and nonlinear parameters extracted from single EHG recordings.
However, multichannel analysis, which includes information from the whole uterus and about coupling between the recording areas, may provide better results.
The cross validation method is often used to design classifiers and evaluate their performance.
However, when the validation dataset is used to tune the classifier hyperparameters, the performance metrics of this dataset may not properly assess its generalization capacity.
In this work, we developed and compared different classifiers, based on artificial neural networks, for predicting preterm labor using EHG features from single and multichannel recordings.
A set of temporal, spectral, nonlinear, and synchronization parameters computed from EHG recordings was used as the input features.
All the classifiers were evaluated on independent test datasets, which were never “seen” by the models, to determine their generalization capacity.
Classifiers’ performance was also evaluated when obstetrical data were included.
The experimental results show that the classifier performance metrics were significantly lower in the test dataset (AUC range 76-91%) than in the train and validation sets (AUC range 90-99%).
The multichannel classifiers outperformed the single-channel classifiers, especially when information was combined into mean efficiency indexes and included coupling information between channels.
Including obstetrical data slightly improved the classifier metrics and reached an AUC of 91.1±2.5% for the test dataset.
These results show promise for the transfer of the EHG technique to preterm labor prediction in clinical practice.
نمط استشهاد جمعية علماء النفس الأمريكية (APA)
Mas-Cabo, J.& Prats-Boluda, Gema& Garcia-Casado, Javier& Alberola-Rubio, J.& Perales-Marín, Alfredo& Ye-Lin, Y.. 2019. Design and Assessment of a Robust and Generalizable ANN-Based Classifier for the Prediction of Premature Birth by means of Multichannel Electrohysterographic Records. Journal of Sensors،Vol. 2019, no. 2019, pp.1-13.
https://search.emarefa.net/detail/BIM-1191288
نمط استشهاد الجمعية الأمريكية للغات الحديثة (MLA)
Mas-Cabo, J.…[et al.]. Design and Assessment of a Robust and Generalizable ANN-Based Classifier for the Prediction of Premature Birth by means of Multichannel Electrohysterographic Records. Journal of Sensors No. 2019 (2019), pp.1-13.
https://search.emarefa.net/detail/BIM-1191288
نمط استشهاد الجمعية الطبية الأمريكية (AMA)
Mas-Cabo, J.& Prats-Boluda, Gema& Garcia-Casado, Javier& Alberola-Rubio, J.& Perales-Marín, Alfredo& Ye-Lin, Y.. Design and Assessment of a Robust and Generalizable ANN-Based Classifier for the Prediction of Premature Birth by means of Multichannel Electrohysterographic Records. Journal of Sensors. 2019. Vol. 2019, no. 2019, pp.1-13.
https://search.emarefa.net/detail/BIM-1191288
نوع البيانات
مقالات
لغة النص
الإنجليزية
الملاحظات
Includes bibliographical references
رقم السجل
BIM-1191288
قاعدة معامل التأثير والاستشهادات المرجعية العربي "ارسيف Arcif"
أضخم قاعدة بيانات عربية للاستشهادات المرجعية للمجلات العلمية المحكمة الصادرة في العالم العربي
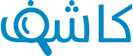
تقوم هذه الخدمة بالتحقق من التشابه أو الانتحال في الأبحاث والمقالات العلمية والأطروحات الجامعية والكتب والأبحاث باللغة العربية، وتحديد درجة التشابه أو أصالة الأعمال البحثية وحماية ملكيتها الفكرية. تعرف اكثر
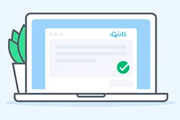