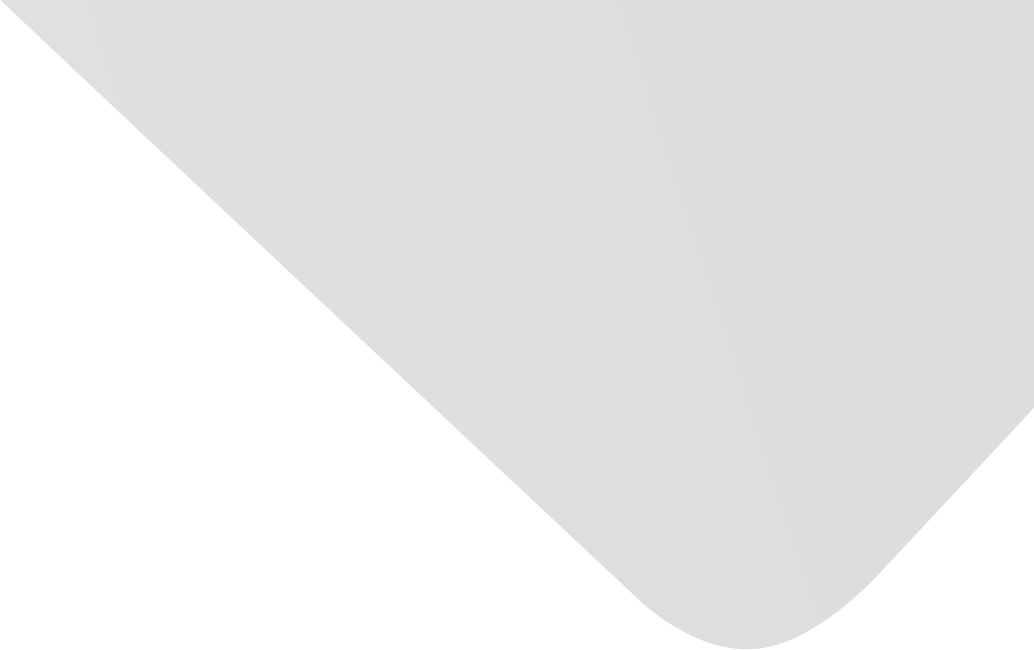
BE-FNet: 3D Bounding Box Estimation Feature Pyramid Network for Accurate and Efficient Maxillary Sinus Segmentation
المؤلفون المشاركون
Deng, Zhuofu
Zhu, Zhiliang
Wang, Binbin
المصدر
Mathematical Problems in Engineering
العدد
المجلد 2020، العدد 2020 (31 ديسمبر/كانون الأول 2020)، ص ص. 1-16، 16ص.
الناشر
Hindawi Publishing Corporation
تاريخ النشر
2020-01-28
دولة النشر
مصر
عدد الصفحات
16
التخصصات الرئيسية
الملخص EN
Maxillary sinus segmentation plays an important role in the choice of therapeutic strategies for nasal disease and treatment monitoring.
Difficulties in traditional approaches deal with extremely heterogeneous intensity caused by lesions, abnormal anatomy structures, and blurring boundaries of cavity.
2D and 3D deep convolutional neural networks have grown popular in medical image segmentation due to utilization of large labeled datasets to learn discriminative features.
However, for 3D segmentation in medical images, 2D networks are not competent in extracting more significant spacial features, and 3D ones suffer from unbearable burden of computation, which results in great challenges to maxillary sinus segmentation.
In this paper, we propose a deep neural network with an end-to-end manner to generalize a fully automatic 3D segmentation.
At first, our proposed model serves a symmetrical encoder-decoder architecture for multitask of bounding box estimation and in-region 3D segmentation, which cannot reduce excessive computation requirements but eliminate false positives remarkably, promoting 3D segmentation applied in 3D convolutional neural networks.
In addition, an overestimation strategy is presented to avoid overfitting phenomena in conventional multitask networks.
Meanwhile, we introduce residual dense blocks to increase the depth of the proposed network and attention excitation mechanism to improve the performance of bounding box estimation, both of which bring little influence to computation cost.
Especially, the structure of multilevel feature fusion in the pyramid network strengthens the ability of identification to global and local discriminative features in foreground and background achieving more advanced segmentation results.
At last, to address problems of blurring boundary and class imbalance in medical images, a hybrid loss function is designed for multiple tasks.
To illustrate the strength of our proposed model, we evaluated it against the state-of-the-art methods.
Our model performed better significantly with an average Dice 0.947±0.031, VOE 10.23±5.29, and ASD 2.86±2.11, respectively, which denotes a promising technique with strong robust in practice.
نمط استشهاد جمعية علماء النفس الأمريكية (APA)
Deng, Zhuofu& Wang, Binbin& Zhu, Zhiliang. 2020. BE-FNet: 3D Bounding Box Estimation Feature Pyramid Network for Accurate and Efficient Maxillary Sinus Segmentation. Mathematical Problems in Engineering،Vol. 2020, no. 2020, pp.1-16.
https://search.emarefa.net/detail/BIM-1196124
نمط استشهاد الجمعية الأمريكية للغات الحديثة (MLA)
Deng, Zhuofu…[et al.]. BE-FNet: 3D Bounding Box Estimation Feature Pyramid Network for Accurate and Efficient Maxillary Sinus Segmentation. Mathematical Problems in Engineering No. 2020 (2020), pp.1-16.
https://search.emarefa.net/detail/BIM-1196124
نمط استشهاد الجمعية الطبية الأمريكية (AMA)
Deng, Zhuofu& Wang, Binbin& Zhu, Zhiliang. BE-FNet: 3D Bounding Box Estimation Feature Pyramid Network for Accurate and Efficient Maxillary Sinus Segmentation. Mathematical Problems in Engineering. 2020. Vol. 2020, no. 2020, pp.1-16.
https://search.emarefa.net/detail/BIM-1196124
نوع البيانات
مقالات
لغة النص
الإنجليزية
الملاحظات
Includes bibliographical references
رقم السجل
BIM-1196124
قاعدة معامل التأثير والاستشهادات المرجعية العربي "ارسيف Arcif"
أضخم قاعدة بيانات عربية للاستشهادات المرجعية للمجلات العلمية المحكمة الصادرة في العالم العربي
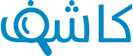
تقوم هذه الخدمة بالتحقق من التشابه أو الانتحال في الأبحاث والمقالات العلمية والأطروحات الجامعية والكتب والأبحاث باللغة العربية، وتحديد درجة التشابه أو أصالة الأعمال البحثية وحماية ملكيتها الفكرية. تعرف اكثر
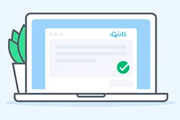