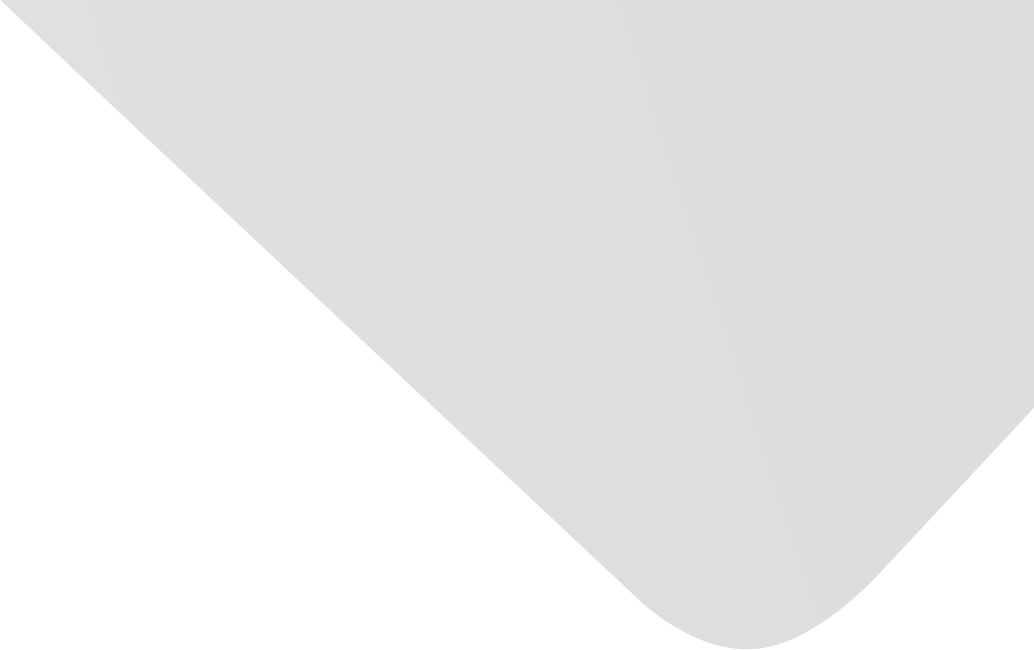
Bearing Fault Diagnosis with Kernel Sparse Representation Classification Based on Adaptive Local Iterative Filtering-Enhanced Multiscale Entropy Features
المؤلفون المشاركون
Liu, Ming
Zhang, Jinbao
Zhao, Yongqiang
Li, Xinglin
المصدر
Mathematical Problems in Engineering
العدد
المجلد 2019، العدد 2019 (31 ديسمبر/كانون الأول 2019)، ص ص. 1-17، 17ص.
الناشر
Hindawi Publishing Corporation
تاريخ النشر
2019-06-04
دولة النشر
مصر
عدد الصفحات
17
التخصصات الرئيسية
الملخص EN
To improve the bearings diagnosis accuracy considering multiple fault types with small samples, a new approach that combined adaptive local iterative filtering (ALIF), multiscale entropy features, and kernel sparse representation classification (KSRC) is put forward in this paper.
ALIF is used to adaptively decompose the nonlinear, nonstationary vibration signals into a sum of intrinsic mode functions (IMFs).
Multiple entropy features such as sample entropy, fuzzy entropy, and permutation entropy with multiscale are computed from the first three IMFs and a total of one hundred and eighty features are obtained.
After normalization, the features are employed to train and test the classifier KSRC, respectively.
Finally, the proposed approach is evaluated with two experimental tests.
One is concerned with different types of bearing faults from the centrifugal pump; and the other is from Case Western Reserve University (CWRU) considering 12 bearing fault states.
Experimental results have proved that the proposed approach is efficient for bearing fault diagnosis, and high accuracy will be obtained with high dimensional features through small samples.
نمط استشهاد جمعية علماء النفس الأمريكية (APA)
Zhang, Jinbao& Zhao, Yongqiang& Li, Xinglin& Liu, Ming. 2019. Bearing Fault Diagnosis with Kernel Sparse Representation Classification Based on Adaptive Local Iterative Filtering-Enhanced Multiscale Entropy Features. Mathematical Problems in Engineering،Vol. 2019, no. 2019, pp.1-17.
https://search.emarefa.net/detail/BIM-1197229
نمط استشهاد الجمعية الأمريكية للغات الحديثة (MLA)
Zhang, Jinbao…[et al.]. Bearing Fault Diagnosis with Kernel Sparse Representation Classification Based on Adaptive Local Iterative Filtering-Enhanced Multiscale Entropy Features. Mathematical Problems in Engineering No. 2019 (2019), pp.1-17.
https://search.emarefa.net/detail/BIM-1197229
نمط استشهاد الجمعية الطبية الأمريكية (AMA)
Zhang, Jinbao& Zhao, Yongqiang& Li, Xinglin& Liu, Ming. Bearing Fault Diagnosis with Kernel Sparse Representation Classification Based on Adaptive Local Iterative Filtering-Enhanced Multiscale Entropy Features. Mathematical Problems in Engineering. 2019. Vol. 2019, no. 2019, pp.1-17.
https://search.emarefa.net/detail/BIM-1197229
نوع البيانات
مقالات
لغة النص
الإنجليزية
الملاحظات
Includes bibliographical references
رقم السجل
BIM-1197229
قاعدة معامل التأثير والاستشهادات المرجعية العربي "ارسيف Arcif"
أضخم قاعدة بيانات عربية للاستشهادات المرجعية للمجلات العلمية المحكمة الصادرة في العالم العربي
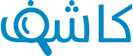
تقوم هذه الخدمة بالتحقق من التشابه أو الانتحال في الأبحاث والمقالات العلمية والأطروحات الجامعية والكتب والأبحاث باللغة العربية، وتحديد درجة التشابه أو أصالة الأعمال البحثية وحماية ملكيتها الفكرية. تعرف اكثر
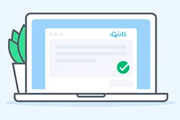