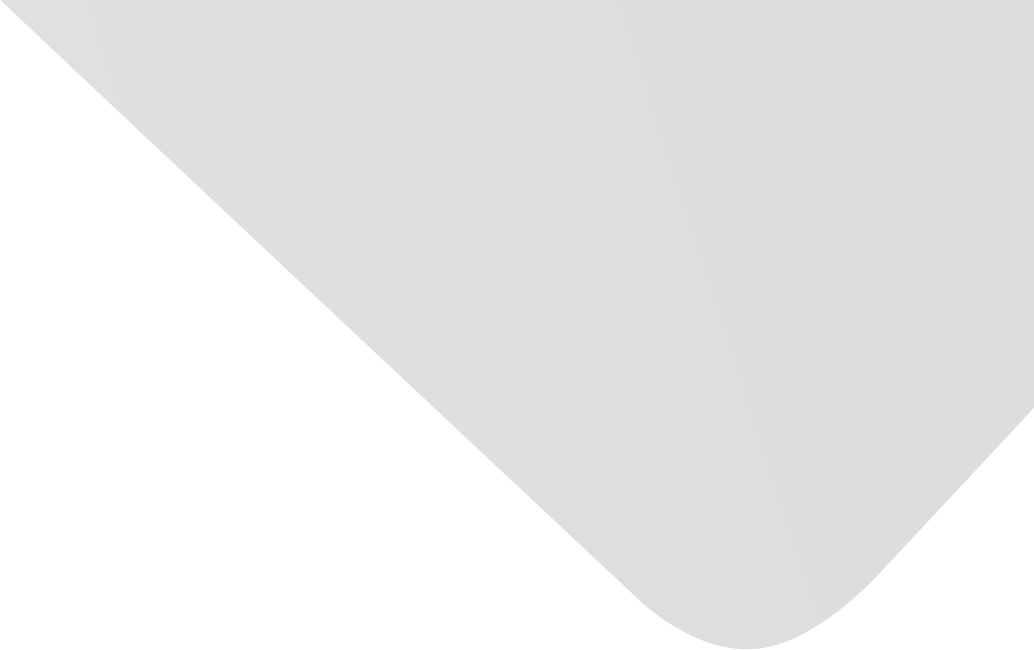
Improved Adaptive Holonic Particle Swarm Optimization
المؤلفون المشاركون
Li, Hao
Jin, Hongbin
Wang, Hanzhong
Ma, Yanyan
المصدر
Mathematical Problems in Engineering
العدد
المجلد 2019، العدد 2019 (31 ديسمبر/كانون الأول 2019)، ص ص. 1-22، 22ص.
الناشر
Hindawi Publishing Corporation
تاريخ النشر
2019-12-12
دولة النشر
مصر
عدد الصفحات
22
التخصصات الرئيسية
الملخص EN
For the first time , the Holonic Particle Swarm Optimization (HPSO ) algorithm applies multiagent theory about the improvement in the PSO algorithm and achieved good results.
In order to further improve the performance of the algorithm, this paper proposes an improved Adaptive Holonic Particle Swarm Optimization (AHPSO) algorithm.
Firstly, a brief review of the HPSO algorithm is carried out, and the HPSO algorithm can be further studied in three aspects: grouping strategy, iteration number setting, and state switching discrimination.
The HPSO algorithm uses an approximately uniform grouping strategy that is the simplest but does not consider the connections between particles.
And if the particles with larger or smaller differences are grouped together in different search stages, the search efficiency will be improved.
Therefore, this paper proposes a grouping strategy based on information entropy and system clustering and combines two grouping strategies with corresponding search methods.
The performance of the HPSO algorithm depends on the setting of the number of iterations.
If it is too small, it is difficult to search for the optimal and it wastes so many computing resources.
Therefore, this paper constructs an adaptive termination condition that causes the particles to terminate spontaneously after convergence.
The HPSO algorithm only performs a conversion from extensive search to exact search and still has the potential to fall into local optimum.
This paper proposes a state switching condition to improve the probability that the algorithm jumps out of the local optimum.
Finally, AHPSO and HPSO are compared by using 22 groups of standard test functions.
AHPSO is faster in convergence than HPSO, and the number of iterations of AHPSO convergence is employed in HPSO.
At this point, there exists a large gap between HPSO and the optimal solution, i.e., AHPSO can have better algorithm efficiency without setting the number of iterations.
نمط استشهاد جمعية علماء النفس الأمريكية (APA)
Li, Hao& Jin, Hongbin& Wang, Hanzhong& Ma, Yanyan. 2019. Improved Adaptive Holonic Particle Swarm Optimization. Mathematical Problems in Engineering،Vol. 2019, no. 2019, pp.1-22.
https://search.emarefa.net/detail/BIM-1197398
نمط استشهاد الجمعية الأمريكية للغات الحديثة (MLA)
Li, Hao…[et al.]. Improved Adaptive Holonic Particle Swarm Optimization. Mathematical Problems in Engineering No. 2019 (2019), pp.1-22.
https://search.emarefa.net/detail/BIM-1197398
نمط استشهاد الجمعية الطبية الأمريكية (AMA)
Li, Hao& Jin, Hongbin& Wang, Hanzhong& Ma, Yanyan. Improved Adaptive Holonic Particle Swarm Optimization. Mathematical Problems in Engineering. 2019. Vol. 2019, no. 2019, pp.1-22.
https://search.emarefa.net/detail/BIM-1197398
نوع البيانات
مقالات
لغة النص
الإنجليزية
الملاحظات
Includes bibliographical references
رقم السجل
BIM-1197398
قاعدة معامل التأثير والاستشهادات المرجعية العربي "ارسيف Arcif"
أضخم قاعدة بيانات عربية للاستشهادات المرجعية للمجلات العلمية المحكمة الصادرة في العالم العربي
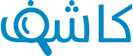
تقوم هذه الخدمة بالتحقق من التشابه أو الانتحال في الأبحاث والمقالات العلمية والأطروحات الجامعية والكتب والأبحاث باللغة العربية، وتحديد درجة التشابه أو أصالة الأعمال البحثية وحماية ملكيتها الفكرية. تعرف اكثر
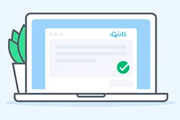