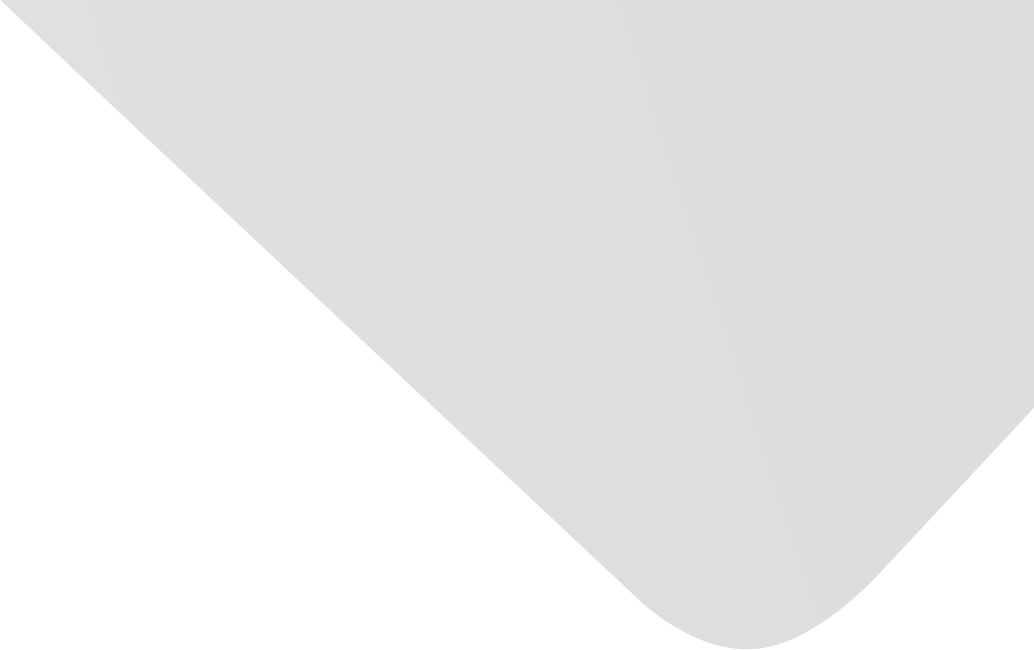
Complete Defense Framework to Protect Deep Neural Networks against Adversarial Examples
المؤلفون المشاركون
Sun, Guangling
Su, Yuying
Qin, Chuan
Xu, Wenbo
Lu, Xiaofeng
Ceglowski, Andrzej
المصدر
Mathematical Problems in Engineering
العدد
المجلد 2020، العدد 2020 (31 ديسمبر/كانون الأول 2020)، ص ص. 1-17، 17ص.
الناشر
Hindawi Publishing Corporation
تاريخ النشر
2020-05-11
دولة النشر
مصر
عدد الصفحات
17
التخصصات الرئيسية
الملخص EN
Although Deep Neural Networks (DNNs) have achieved great success on various applications, investigations have increasingly shown DNNs to be highly vulnerable when adversarial examples are used as input.
Here, we present a comprehensive defense framework to protect DNNs against adversarial examples.
First, we present statistical and minor alteration detectors to filter out adversarial examples contaminated by noticeable and unnoticeable perturbations, respectively.
Then, we ensemble the detectors, a deep Residual Generative Network (ResGN), and an adversarially trained targeted network, to construct a complete defense framework.
In this framework, the ResGN is our previously proposed network which is used to remove adversarial perturbations, and the adversarially trained targeted network is a network that is learned through adversarial training.
Specifically, once the detectors determine an input example to be adversarial, it is cleaned by ResGN and then classified by the adversarially trained targeted network; otherwise, it is directly classified by this network.
We empirically evaluate the proposed complete defense on ImageNet dataset.
The results confirm the robustness against current representative attacking methods including fast gradient sign method, randomized fast gradient sign method, basic iterative method, universal adversarial perturbations, DeepFool method, and Carlini & Wagner method.
نمط استشهاد جمعية علماء النفس الأمريكية (APA)
Sun, Guangling& Su, Yuying& Qin, Chuan& Xu, Wenbo& Lu, Xiaofeng& Ceglowski, Andrzej. 2020. Complete Defense Framework to Protect Deep Neural Networks against Adversarial Examples. Mathematical Problems in Engineering،Vol. 2020, no. 2020, pp.1-17.
https://search.emarefa.net/detail/BIM-1201037
نمط استشهاد الجمعية الأمريكية للغات الحديثة (MLA)
Sun, Guangling…[et al.]. Complete Defense Framework to Protect Deep Neural Networks against Adversarial Examples. Mathematical Problems in Engineering No. 2020 (2020), pp.1-17.
https://search.emarefa.net/detail/BIM-1201037
نمط استشهاد الجمعية الطبية الأمريكية (AMA)
Sun, Guangling& Su, Yuying& Qin, Chuan& Xu, Wenbo& Lu, Xiaofeng& Ceglowski, Andrzej. Complete Defense Framework to Protect Deep Neural Networks against Adversarial Examples. Mathematical Problems in Engineering. 2020. Vol. 2020, no. 2020, pp.1-17.
https://search.emarefa.net/detail/BIM-1201037
نوع البيانات
مقالات
لغة النص
الإنجليزية
الملاحظات
Includes bibliographical references
رقم السجل
BIM-1201037
قاعدة معامل التأثير والاستشهادات المرجعية العربي "ارسيف Arcif"
أضخم قاعدة بيانات عربية للاستشهادات المرجعية للمجلات العلمية المحكمة الصادرة في العالم العربي
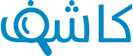
تقوم هذه الخدمة بالتحقق من التشابه أو الانتحال في الأبحاث والمقالات العلمية والأطروحات الجامعية والكتب والأبحاث باللغة العربية، وتحديد درجة التشابه أو أصالة الأعمال البحثية وحماية ملكيتها الفكرية. تعرف اكثر
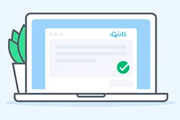