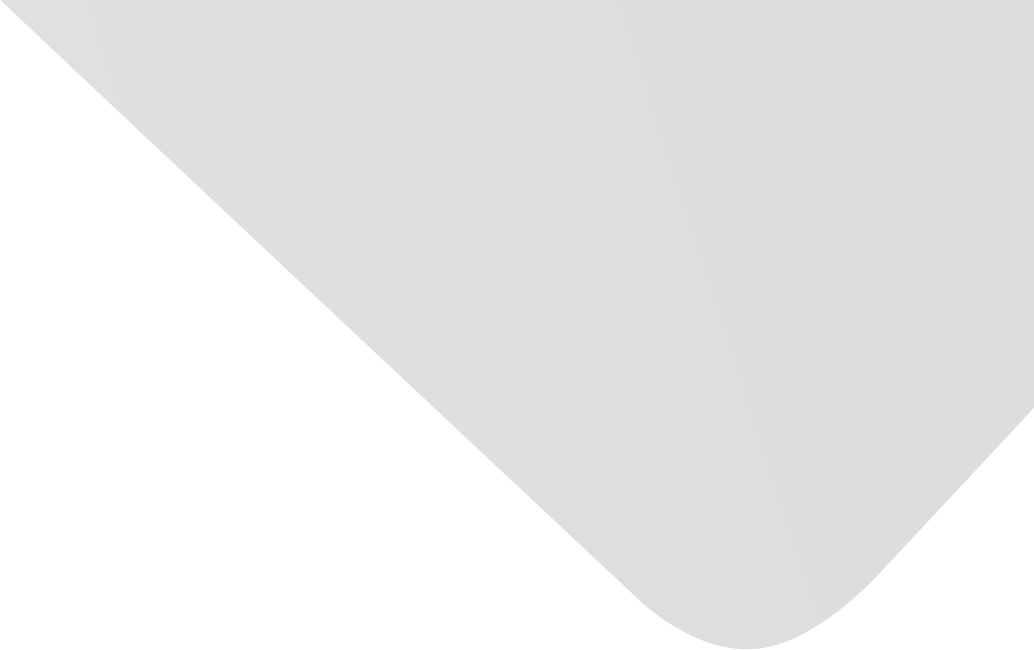
Combat Mobile Evasive Malware via Skip-Gram-Based Malware Detection
المؤلفون المشاركون
Egitmen, Alper
Bulut, Irfan
Aygun, R. Can
Gunduz, A. Bilge
Seyrekbasan, Omer
Yavuz, A. Gokhan
المصدر
Security and Communication Networks
العدد
المجلد 2020، العدد 2020 (31 ديسمبر/كانون الأول 2020)، ص ص. 1-10، 10ص.
الناشر
Hindawi Publishing Corporation
تاريخ النشر
2020-04-20
دولة النشر
مصر
عدد الصفحات
10
التخصصات الرئيسية
تكنولوجيا المعلومات وعلم الحاسوب
الملخص EN
Android malware detection is an important research topic in the security area.
There are a variety of existing malware detection models based on static and dynamic malware analysis.
However, most of these models are not very successful when it comes to evasive malware detection.
In this study, we aimed to create a malware detection model based on a natural language model called skip-gram to detect evasive malware with the highest accuracy rate possible.
In order to train and test our proposed model, we used an up-to-date malware dataset called Argus Android Malware Dataset (AMD) since the AMD contains various evasive malware families and detailed information about them.
Meanwhile, for the benign samples, we used Comodo Android Benign Dataset.
Our proposed model starts with extracting skip-gram-based features from instruction sequences of Android applications.
Then it applies several machine learning algorithms to classify samples as benign or malware.
We tested our proposed model with two different scenarios.
In the first scenario, the random forest-based classifier performed with 95.64% detection accuracy on the entire dataset and 95% detection accuracy against evasive only samples.
In the second scenario, we created a test dataset that contained zero-day malware samples only.
For the training set, we did not use any sample that belongs to the malware families in the test set.
The random forest-based model performed with 37.36% accuracy rate against zero-day malware.
In addition, we compared our proposed model’s malware detection performance against several commercial antimalware applications using VirusTotal API.
Our model outperformed 7 out of 10 antimalware applications and tied with one of them on the same test scenario.
نمط استشهاد جمعية علماء النفس الأمريكية (APA)
Egitmen, Alper& Bulut, Irfan& Aygun, R. Can& Gunduz, A. Bilge& Seyrekbasan, Omer& Yavuz, A. Gokhan. 2020. Combat Mobile Evasive Malware via Skip-Gram-Based Malware Detection. Security and Communication Networks،Vol. 2020, no. 2020, pp.1-10.
https://search.emarefa.net/detail/BIM-1208515
نمط استشهاد الجمعية الأمريكية للغات الحديثة (MLA)
Egitmen, Alper…[et al.]. Combat Mobile Evasive Malware via Skip-Gram-Based Malware Detection. Security and Communication Networks No. 2020 (2020), pp.1-10.
https://search.emarefa.net/detail/BIM-1208515
نمط استشهاد الجمعية الطبية الأمريكية (AMA)
Egitmen, Alper& Bulut, Irfan& Aygun, R. Can& Gunduz, A. Bilge& Seyrekbasan, Omer& Yavuz, A. Gokhan. Combat Mobile Evasive Malware via Skip-Gram-Based Malware Detection. Security and Communication Networks. 2020. Vol. 2020, no. 2020, pp.1-10.
https://search.emarefa.net/detail/BIM-1208515
نوع البيانات
مقالات
لغة النص
الإنجليزية
الملاحظات
Includes bibliographical references
رقم السجل
BIM-1208515
قاعدة معامل التأثير والاستشهادات المرجعية العربي "ارسيف Arcif"
أضخم قاعدة بيانات عربية للاستشهادات المرجعية للمجلات العلمية المحكمة الصادرة في العالم العربي
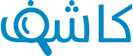
تقوم هذه الخدمة بالتحقق من التشابه أو الانتحال في الأبحاث والمقالات العلمية والأطروحات الجامعية والكتب والأبحاث باللغة العربية، وتحديد درجة التشابه أو أصالة الأعمال البحثية وحماية ملكيتها الفكرية. تعرف اكثر
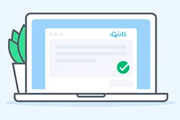