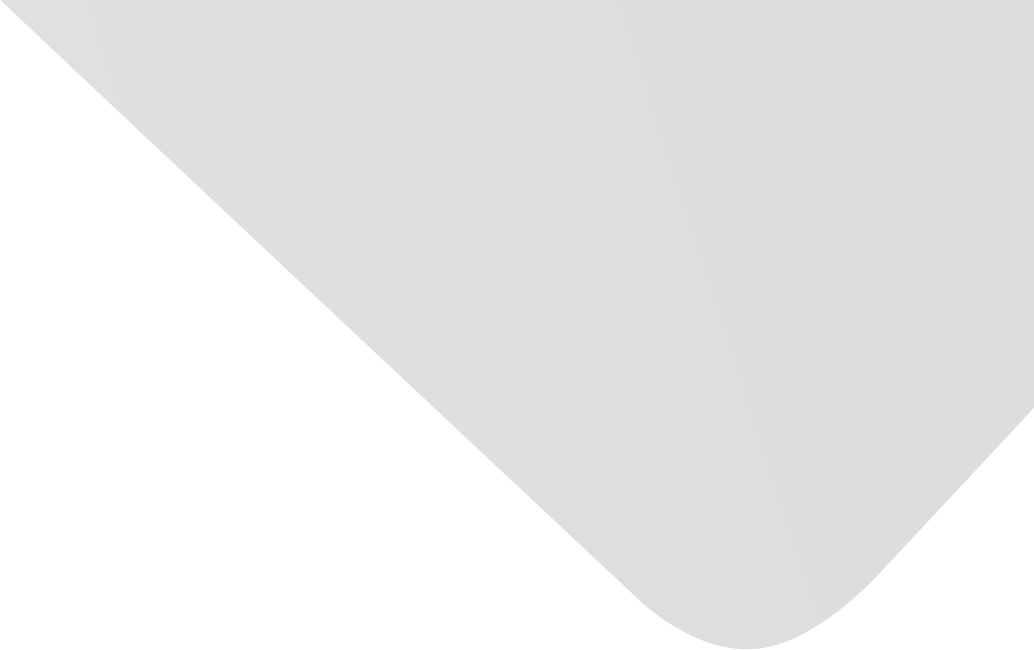
Data-Driven Decision-Support System for Speaker Identification Using E-Vector System
المؤلفون المشاركون
Chen, C. L. Philip
Li, Tieshan
Ma, He
Zuo, Yi
المصدر
العدد
المجلد 2020، العدد 2020 (31 ديسمبر/كانون الأول 2020)، ص ص. 1-13، 13ص.
الناشر
Hindawi Publishing Corporation
تاريخ النشر
2020-06-29
دولة النشر
مصر
عدد الصفحات
13
التخصصات الرئيسية
الملخص EN
Recently, biometric authorizations using fingerprint, voiceprint, and facial features have garnered considerable attention from the public with the development of recognition techniques and popularization of the smartphone.
Among such biometrics, voiceprint has a personal identity as high as that of fingerprint and also uses a noncontact mode to recognize similar faces.
Speech signal-processing is one of the keys to accuracy in voice recognition.
Most voice-identification systems still employ the mel-scale frequency cepstrum coefficient (MFCC) as the key vocal feature.
The quality and accuracy of the MFCC are dependent on the prepared phrase, which belongs to text-dependent speaker identification.
In contrast, several new features, such as d-vector, provide a black-box process in vocal feature learning.
To address these aspects, a novel data-driven approach for vocal feature extraction based on a decision-support system (DSS) is proposed in this study.
Each speech signal can be transformed into a vector representing the vocal features using this DSS.
The establishment of this DSS involves three steps: (i) voice data preprocessing, (ii) hierarchical cluster analysis for the inverse discrete cosine transform cepstrum coefficient, and (iii) learning the E-vector through minimization of the Euclidean metric.
We compare experiments to verify the E-vectors extracted by this DSS with other vocal features measures and apply them to both text-dependent and text-independent datasets.
In the experiments containing one utterance of each speaker, the average accuracy of the E-vector is improved by approximately 1.5% over the MFCC.
In the experiments containing multiple utterances of each speaker, the average micro-F1 score of the E-vector is also improved by approximately 2.1% over the MFCC.
The results of the E-vector show remarkable advantages when applied to both the Texas Instruments/Massachusetts Institute of Technology corpus and LibriSpeech corpus.
These improvements of the E-vector contribute to the capabilities of speaker identification and also enhance its usability for more real-world identification tasks.
نمط استشهاد جمعية علماء النفس الأمريكية (APA)
Ma, He& Zuo, Yi& Li, Tieshan& Chen, C. L. Philip. 2020. Data-Driven Decision-Support System for Speaker Identification Using E-Vector System. Scientific Programming،Vol. 2020, no. 2020, pp.1-13.
https://search.emarefa.net/detail/BIM-1209036
نمط استشهاد الجمعية الأمريكية للغات الحديثة (MLA)
Ma, He…[et al.]. Data-Driven Decision-Support System for Speaker Identification Using E-Vector System. Scientific Programming No. 2020 (2020), pp.1-13.
https://search.emarefa.net/detail/BIM-1209036
نمط استشهاد الجمعية الطبية الأمريكية (AMA)
Ma, He& Zuo, Yi& Li, Tieshan& Chen, C. L. Philip. Data-Driven Decision-Support System for Speaker Identification Using E-Vector System. Scientific Programming. 2020. Vol. 2020, no. 2020, pp.1-13.
https://search.emarefa.net/detail/BIM-1209036
نوع البيانات
مقالات
لغة النص
الإنجليزية
الملاحظات
Includes bibliographical references
رقم السجل
BIM-1209036
قاعدة معامل التأثير والاستشهادات المرجعية العربي "ارسيف Arcif"
أضخم قاعدة بيانات عربية للاستشهادات المرجعية للمجلات العلمية المحكمة الصادرة في العالم العربي
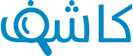
تقوم هذه الخدمة بالتحقق من التشابه أو الانتحال في الأبحاث والمقالات العلمية والأطروحات الجامعية والكتب والأبحاث باللغة العربية، وتحديد درجة التشابه أو أصالة الأعمال البحثية وحماية ملكيتها الفكرية. تعرف اكثر
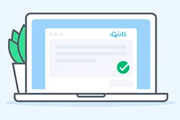