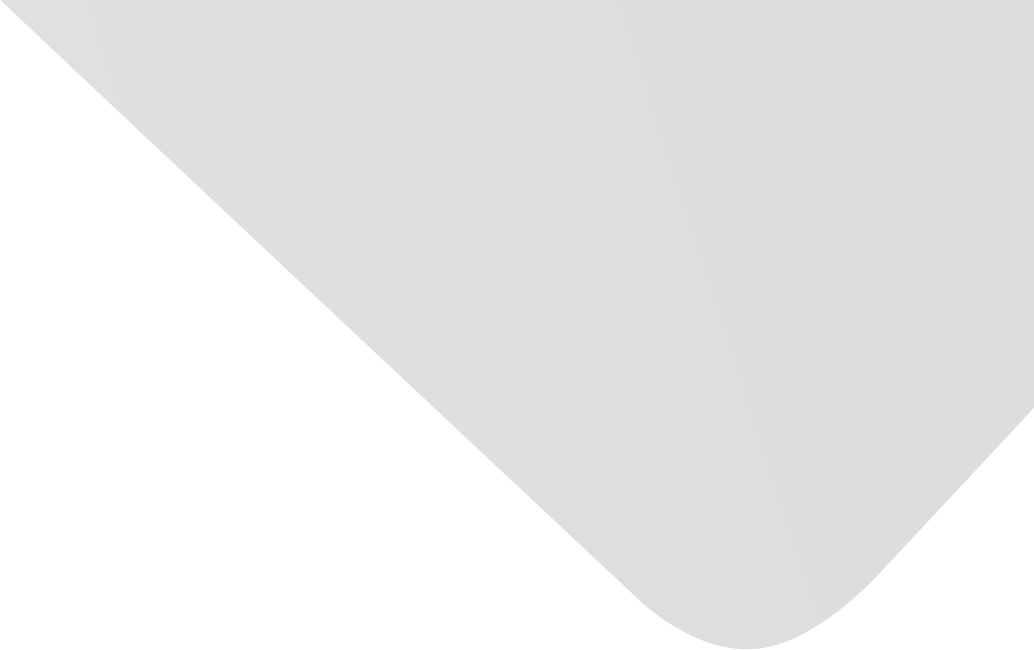
QuPiD Attack: Machine Learning-Based Privacy Quantification Mechanism for PIR Protocols in Health-Related Web Search
المؤلفون المشاركون
Ullah, Mohib
Khan, Rafiullah
Ahmad, Arshad
Binsawad, Muhammad
Alsayed, Alhuseen Omar
Islam, Muhammad Arshad
المصدر
العدد
المجلد 2020، العدد 2020 (31 ديسمبر/كانون الأول 2020)، ص ص. 1-11، 11ص.
الناشر
Hindawi Publishing Corporation
تاريخ النشر
2020-07-14
دولة النشر
مصر
عدد الصفحات
11
التخصصات الرئيسية
الملخص EN
With the advancement in ICT, web search engines have become a preferred source to find health-related information published over the Internet.
Google alone receives more than one billion health-related queries on a daily basis.
However, in order to provide the results most relevant to the user, WSEs maintain the users’ profiles.
These profiles may contain private and sensitive information such as the user’s health condition, disease status, and others.
Health-related queries contain privacy-sensitive information that may infringe user’s privacy, as the identity of a user is exposed and may be misused by the WSE and third parties.
This raises serious concerns since the identity of a user is exposed and may be misused by third parties.
One well-known solution to preserve privacy involves issuing the queries via peer-to-peer private information retrieval protocol, such as useless user profile (UUP), thereby hiding the user’s identity from the WSE.
This paper investigates the level of protection offered by UUP.
For this purpose, we present QuPiD (query profile distance) attack: a machine learning-based attack that evaluates the effectiveness of UUP in privacy protection.
QuPiD attack determines the distance between the user’s profile (web search history) and upcoming query using our proposed novel feature vector.
The experiments were conducted using ten classification algorithms belonging to the tree-based, rule-based, lazy learner, metaheuristic, and Bayesian families for the sake of comparison.
Furthermore, two subsets of an America Online dataset (noisy and clean datasets) were used for experimentation.
The results show that the proposed QuPiD attack associates more than 70% queries to the correct user with a precision of over 72% for the clean dataset, while for the noisy dataset, the proposed QuPiD attack associates more than 40% queries to the correct user with 70% precision.
نمط استشهاد جمعية علماء النفس الأمريكية (APA)
Khan, Rafiullah& Ahmad, Arshad& Alsayed, Alhuseen Omar& Binsawad, Muhammad& Islam, Muhammad Arshad& Ullah, Mohib. 2020. QuPiD Attack: Machine Learning-Based Privacy Quantification Mechanism for PIR Protocols in Health-Related Web Search. Scientific Programming،Vol. 2020, no. 2020, pp.1-11.
https://search.emarefa.net/detail/BIM-1209272
نمط استشهاد الجمعية الأمريكية للغات الحديثة (MLA)
Khan, Rafiullah…[et al.]. QuPiD Attack: Machine Learning-Based Privacy Quantification Mechanism for PIR Protocols in Health-Related Web Search. Scientific Programming No. 2020 (2020), pp.1-11.
https://search.emarefa.net/detail/BIM-1209272
نمط استشهاد الجمعية الطبية الأمريكية (AMA)
Khan, Rafiullah& Ahmad, Arshad& Alsayed, Alhuseen Omar& Binsawad, Muhammad& Islam, Muhammad Arshad& Ullah, Mohib. QuPiD Attack: Machine Learning-Based Privacy Quantification Mechanism for PIR Protocols in Health-Related Web Search. Scientific Programming. 2020. Vol. 2020, no. 2020, pp.1-11.
https://search.emarefa.net/detail/BIM-1209272
نوع البيانات
مقالات
لغة النص
الإنجليزية
الملاحظات
Includes bibliographical references
رقم السجل
BIM-1209272
قاعدة معامل التأثير والاستشهادات المرجعية العربي "ارسيف Arcif"
أضخم قاعدة بيانات عربية للاستشهادات المرجعية للمجلات العلمية المحكمة الصادرة في العالم العربي
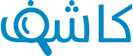
تقوم هذه الخدمة بالتحقق من التشابه أو الانتحال في الأبحاث والمقالات العلمية والأطروحات الجامعية والكتب والأبحاث باللغة العربية، وتحديد درجة التشابه أو أصالة الأعمال البحثية وحماية ملكيتها الفكرية. تعرف اكثر
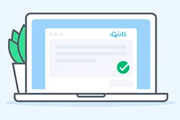