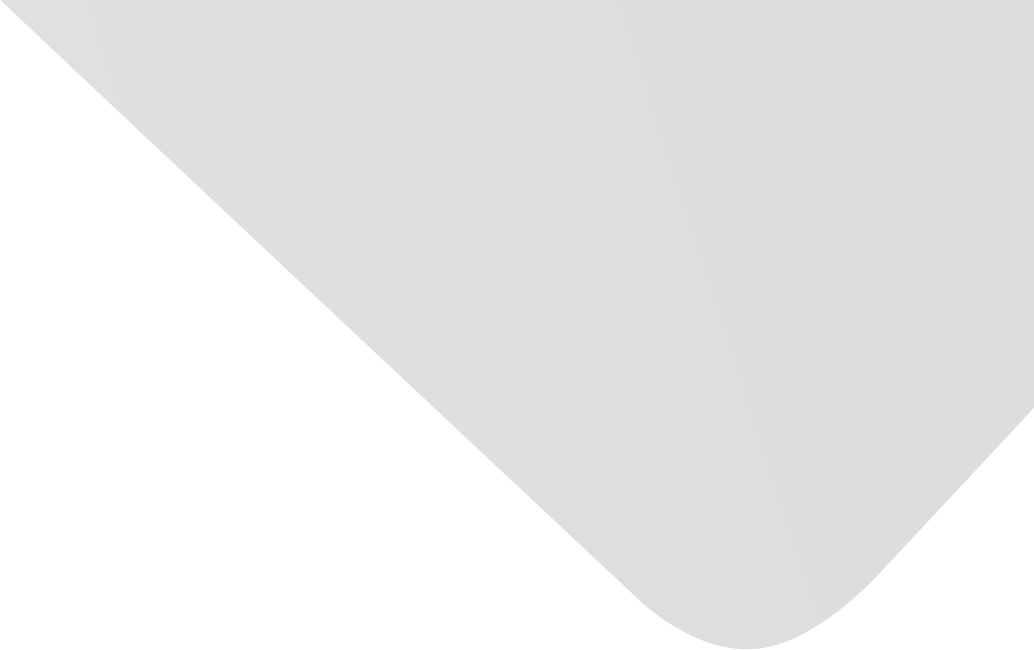
Fault Diagnosis under Variable Working Conditions Based on STFT and Transfer Deep Residual Network
المؤلفون المشاركون
Wang, Aiming
Du, Yan
He, Baomei
Meng, Guoying
Wang, Shuai
المصدر
العدد
المجلد 2020، العدد 2020 (31 ديسمبر/كانون الأول 2020)، ص ص. 1-18، 18ص.
الناشر
Hindawi Publishing Corporation
تاريخ النشر
2020-05-04
دولة النشر
مصر
عدد الصفحات
18
التخصصات الرئيسية
الملخص EN
Fault diagnosis plays a very important role in ensuring the safe and reliable operations of machines.
Currently, the deep learning-based fault diagnosis is attracting increasing attention.
However, fault diagnosis under variable working conditions has been a significant challenge due to the domain discrepancy problem.
This problem is also unavoidable in deep learning-based fault diagnosis methods.
This paper contributes to the ongoing investigation by proposing a new approach for the fault diagnosis under variable working conditions based on STFT and transfer deep residual network (TDRN).
The STFT was employed to convert vibration signal to time-frequency image as the input of the TDRN.
To address the domain discrepancy problem, the TDRN was developed in this paper.
Unlike traditional deep convolutional neural network (DCNN) methods, by combining with transfer learning, the TDRN can make a bridge between two different working conditions, thereby using the knowledge learned from a working condition to achieve a high classification accuracy in another working condition.
Moreover, since the residual learning is introducing, the TDRN can overcome the problems of training difficulty and performance degradation existing in traditional DCNN methods, thus further improving the classification accuracy.
Experiments are conducted on the popular CWRU bearing dataset to validate the effectiveness and superiority of the proposed approach.
The results show that the developed TDRN outperforms those methods without transfer learning and/or residual learning in terms of the accuracy and feature learning ability for the fault diagnosis under variable working conditions.
نمط استشهاد جمعية علماء النفس الأمريكية (APA)
Du, Yan& Wang, Aiming& Wang, Shuai& He, Baomei& Meng, Guoying. 2020. Fault Diagnosis under Variable Working Conditions Based on STFT and Transfer Deep Residual Network. Shock and Vibration،Vol. 2020, no. 2020, pp.1-18.
https://search.emarefa.net/detail/BIM-1209638
نمط استشهاد الجمعية الأمريكية للغات الحديثة (MLA)
Du, Yan…[et al.]. Fault Diagnosis under Variable Working Conditions Based on STFT and Transfer Deep Residual Network. Shock and Vibration No. 2020 (2020), pp.1-18.
https://search.emarefa.net/detail/BIM-1209638
نمط استشهاد الجمعية الطبية الأمريكية (AMA)
Du, Yan& Wang, Aiming& Wang, Shuai& He, Baomei& Meng, Guoying. Fault Diagnosis under Variable Working Conditions Based on STFT and Transfer Deep Residual Network. Shock and Vibration. 2020. Vol. 2020, no. 2020, pp.1-18.
https://search.emarefa.net/detail/BIM-1209638
نوع البيانات
مقالات
لغة النص
الإنجليزية
الملاحظات
Includes bibliographical references
رقم السجل
BIM-1209638
قاعدة معامل التأثير والاستشهادات المرجعية العربي "ارسيف Arcif"
أضخم قاعدة بيانات عربية للاستشهادات المرجعية للمجلات العلمية المحكمة الصادرة في العالم العربي
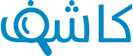
تقوم هذه الخدمة بالتحقق من التشابه أو الانتحال في الأبحاث والمقالات العلمية والأطروحات الجامعية والكتب والأبحاث باللغة العربية، وتحديد درجة التشابه أو أصالة الأعمال البحثية وحماية ملكيتها الفكرية. تعرف اكثر
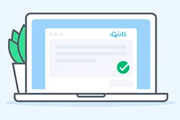