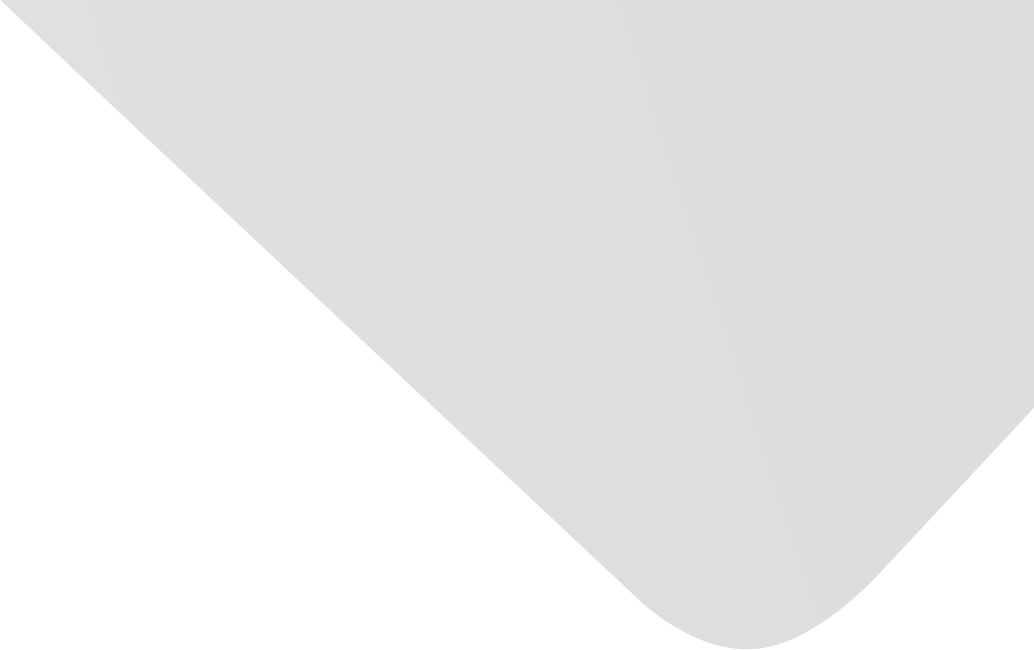
Rolling Bearing Fault Diagnosis Based on Sensitive Feature Transfer Learning and Local Maximum Margin Criterion under Variable Working Condition
المؤلفون المشاركون
Liu, Shiyuan
Dong, Fei
Yu, Xiao
Qian, Xu
المصدر
العدد
المجلد 2020، العدد 2020 (31 ديسمبر/كانون الأول 2020)، ص ص. 1-34، 34ص.
الناشر
Hindawi Publishing Corporation
تاريخ النشر
2020-09-01
دولة النشر
مصر
عدد الصفحات
34
التخصصات الرئيسية
الملخص EN
In real industrial scenarios, the working conditions of bearings are variable, and it is therefore difficult for data-driven diagnosis methods based on conventional machine-learning techniques to guarantee the desirable performance of diagnosis models, as the models assume that the distributions of both the training and testing data are the same.
To enhance the performance of the fault diagnosis of bearings under different working conditions, a novel diagnosis framework inspired by feature extraction, transfer learning (TL), and feature dimensionality reduction is proposed in this work, and dual-tree complex wavelet packet transform (DTCWPT) is used for signal processing.
Additionally, transferable sensitive feature selection by ReliefF and the sum of mean deviation (TSFSR) is proposed to reduce the redundant information of the original feature set, to select sensitive features for fault diagnosis, and to reduce the difference between the marginal distributions of the training and testing feature sets.
Furthermore, a modified feature reduction method, the local maximum margin criterion (LMMC), is proposed to acquire low-dimensional mapping for high-dimensional feature spaces.
Finally, bearing vibration signals collected from two test rigs are analyzed to demonstrate the adaptability, effectiveness, and practicability of the proposed diagnosis framework.
The experimental results show that the proposed method can achieve high diagnosis accuracy and has significant potential benefits in industrial applications.
نمط استشهاد جمعية علماء النفس الأمريكية (APA)
Liu, Shiyuan& Yu, Xiao& Qian, Xu& Dong, Fei. 2020. Rolling Bearing Fault Diagnosis Based on Sensitive Feature Transfer Learning and Local Maximum Margin Criterion under Variable Working Condition. Shock and Vibration،Vol. 2020, no. 2020, pp.1-34.
https://search.emarefa.net/detail/BIM-1210455
نمط استشهاد الجمعية الأمريكية للغات الحديثة (MLA)
Liu, Shiyuan…[et al.]. Rolling Bearing Fault Diagnosis Based on Sensitive Feature Transfer Learning and Local Maximum Margin Criterion under Variable Working Condition. Shock and Vibration No. 2020 (2020), pp.1-34.
https://search.emarefa.net/detail/BIM-1210455
نمط استشهاد الجمعية الطبية الأمريكية (AMA)
Liu, Shiyuan& Yu, Xiao& Qian, Xu& Dong, Fei. Rolling Bearing Fault Diagnosis Based on Sensitive Feature Transfer Learning and Local Maximum Margin Criterion under Variable Working Condition. Shock and Vibration. 2020. Vol. 2020, no. 2020, pp.1-34.
https://search.emarefa.net/detail/BIM-1210455
نوع البيانات
مقالات
لغة النص
الإنجليزية
الملاحظات
Includes bibliographical references
رقم السجل
BIM-1210455
قاعدة معامل التأثير والاستشهادات المرجعية العربي "ارسيف Arcif"
أضخم قاعدة بيانات عربية للاستشهادات المرجعية للمجلات العلمية المحكمة الصادرة في العالم العربي
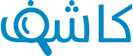
تقوم هذه الخدمة بالتحقق من التشابه أو الانتحال في الأبحاث والمقالات العلمية والأطروحات الجامعية والكتب والأبحاث باللغة العربية، وتحديد درجة التشابه أو أصالة الأعمال البحثية وحماية ملكيتها الفكرية. تعرف اكثر
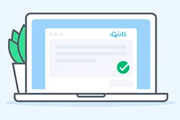