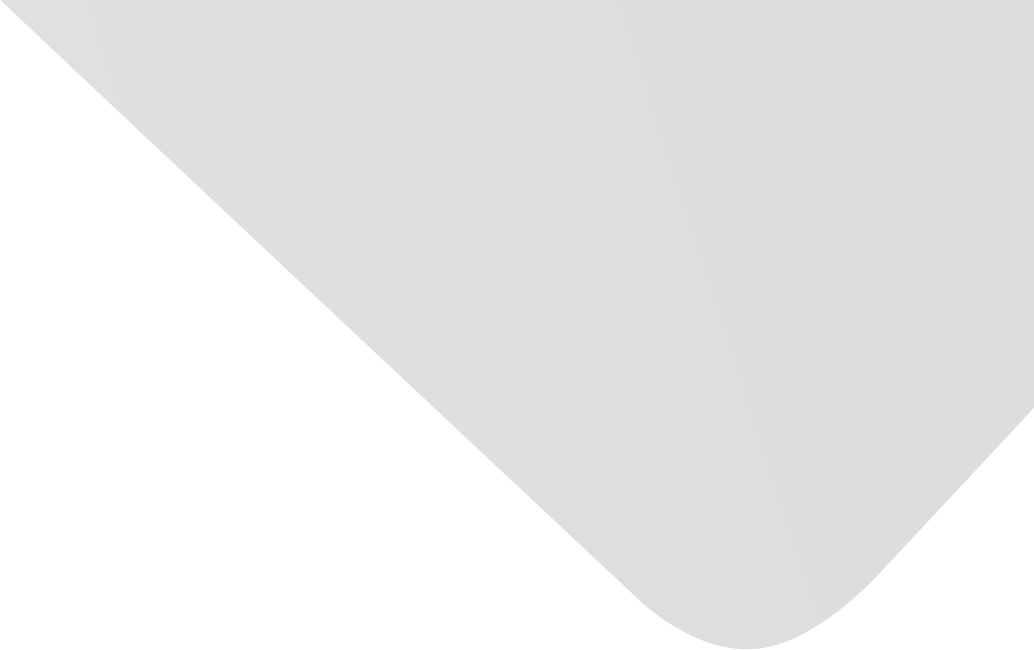
Fault Diagnosis of Induction Motors Using Recurrence Quantification Analysis and LSTM with Weighted BN
المؤلفون المشاركون
Qin, Chengjin
Xiao, Dengyu
Huang, Yixiang
Shi, Haotian
Li, Yanming
المصدر
العدد
المجلد 2019، العدد 2019 (31 ديسمبر/كانون الأول 2019)، ص ص. 1-14، 14ص.
الناشر
Hindawi Publishing Corporation
تاريخ النشر
2019-01-27
دولة النشر
مصر
عدد الصفحات
14
التخصصات الرئيسية
الملخص EN
Motor fault diagnosis has gained much attention from academic research and industry to guarantee motor reliability.
Generally, there exist two major approaches in the feature engineering for motor fault diagnosis: (1) traditional feature learning, which heavily depends on manual feature extraction, is often unable to discover the important underlying representations of faulty motors; (2) state-of-the-art deep learning techniques, which have somewhat improved diagnostic performance, while the intrinsic characteristics of black box and the lack of domain expertise have limited the further improvement.
To cover those shortcomings, in this paper, two manual feature learning approaches are embedded into a deep learning algorithm, and thus, a novel fault diagnosis framework is proposed for three-phase induction motors with a hybrid feature learning method, which combines empirical statistical parameters, recurrence quantification analysis (RQA) and long short-term memory (LSTM) neural network.
In addition, weighted batch normalization (BN), a modification of BN, is designed to evaluate the contributions of the three feature learning approaches.
The proposed method was experimentally demonstrated by carrying out the tests of 8 induction motors with 8 different faulty types.
Results show that compared with other popular intelligent diagnosis methods, the proposed method achieves the highest diagnostic accuracy in both the original dataset and the noised dataset.
It also verifies that RQA can play a bigger role in real-world applications for its excellent performance in dealing with the noised signals.
نمط استشهاد جمعية علماء النفس الأمريكية (APA)
Xiao, Dengyu& Huang, Yixiang& Qin, Chengjin& Shi, Haotian& Li, Yanming. 2019. Fault Diagnosis of Induction Motors Using Recurrence Quantification Analysis and LSTM with Weighted BN. Shock and Vibration،Vol. 2019, no. 2019, pp.1-14.
https://search.emarefa.net/detail/BIM-1211599
نمط استشهاد الجمعية الأمريكية للغات الحديثة (MLA)
Xiao, Dengyu…[et al.]. Fault Diagnosis of Induction Motors Using Recurrence Quantification Analysis and LSTM with Weighted BN. Shock and Vibration No. 2019 (2019), pp.1-14.
https://search.emarefa.net/detail/BIM-1211599
نمط استشهاد الجمعية الطبية الأمريكية (AMA)
Xiao, Dengyu& Huang, Yixiang& Qin, Chengjin& Shi, Haotian& Li, Yanming. Fault Diagnosis of Induction Motors Using Recurrence Quantification Analysis and LSTM with Weighted BN. Shock and Vibration. 2019. Vol. 2019, no. 2019, pp.1-14.
https://search.emarefa.net/detail/BIM-1211599
نوع البيانات
مقالات
لغة النص
الإنجليزية
الملاحظات
Includes bibliographical references
رقم السجل
BIM-1211599
قاعدة معامل التأثير والاستشهادات المرجعية العربي "ارسيف Arcif"
أضخم قاعدة بيانات عربية للاستشهادات المرجعية للمجلات العلمية المحكمة الصادرة في العالم العربي
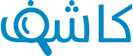
تقوم هذه الخدمة بالتحقق من التشابه أو الانتحال في الأبحاث والمقالات العلمية والأطروحات الجامعية والكتب والأبحاث باللغة العربية، وتحديد درجة التشابه أو أصالة الأعمال البحثية وحماية ملكيتها الفكرية. تعرف اكثر
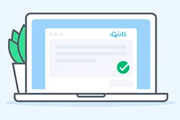