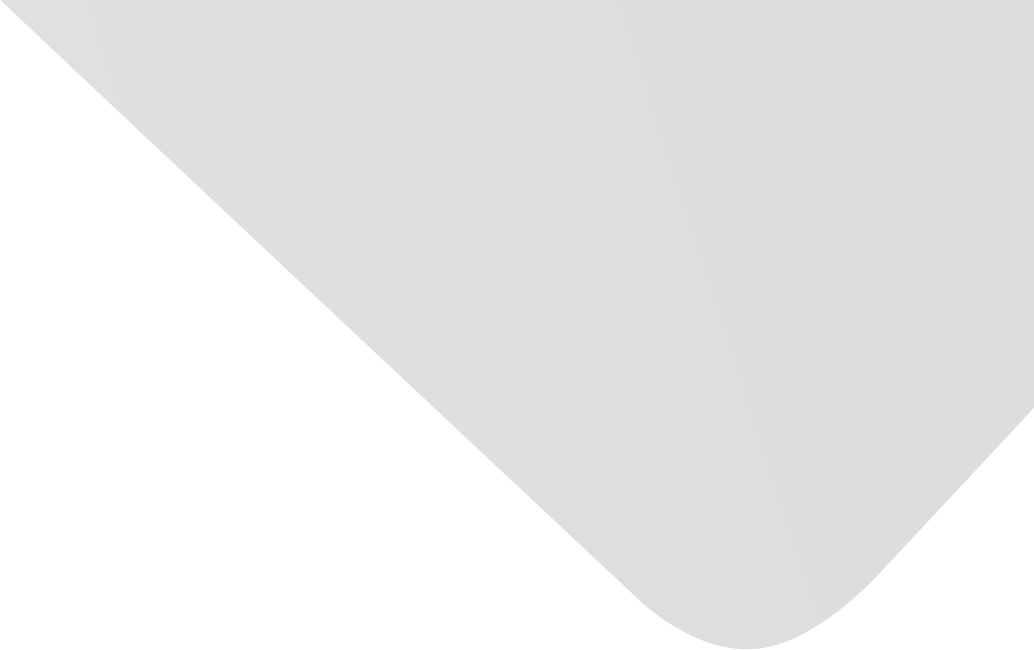
Imbalanced Fault Classification of Bearing via Wasserstein Generative Adversarial Networks with Gradient Penalty
المؤلفون المشاركون
Liu, Guifang
Han, Baokun
Jia, Sixiang
Wang, Jinrui
المصدر
العدد
المجلد 2020، العدد 2020 (31 ديسمبر/كانون الأول 2020)، ص ص. 1-14، 14ص.
الناشر
Hindawi Publishing Corporation
تاريخ النشر
2020-07-21
دولة النشر
مصر
عدد الصفحات
14
التخصصات الرئيسية
الملخص EN
Recently, generative adversarial networks (GANs) are widely applied to increase the amounts of imbalanced input samples in fault diagnosis.
However, the existing GAN-based methods have convergence difficulties and training instability, which affect the fault diagnosis efficiency.
This paper develops a novel framework for imbalanced fault classification based on Wasserstein generative adversarial networks with gradient penalty (WGAN-GP), which interpolates randomly between the true and generated samples to ensure that the transition region between the true and false samples satisfies the Lipschitz constraint.
The process of feature learning is visualized to show the feature extraction process of WGAN-GP.
To verify the availability of the generated samples, a stacked autoencoder (SAE) is set to classify the enhanced dataset composed of the generated samples and original samples.
Furthermore, the exhibition of the loss curve indicates that WGAN-GP has better convergence and faster training speed due to the introduction of the gradient penalty.
Three bearing datasets are employed to verify the effectiveness of the developed framework, and the results show that the proposed framework has an excellent performance in mechanical fault diagnosis under the imbalanced training dataset.
نمط استشهاد جمعية علماء النفس الأمريكية (APA)
Han, Baokun& Jia, Sixiang& Liu, Guifang& Wang, Jinrui. 2020. Imbalanced Fault Classification of Bearing via Wasserstein Generative Adversarial Networks with Gradient Penalty. Shock and Vibration،Vol. 2020, no. 2020, pp.1-14.
https://search.emarefa.net/detail/BIM-1212826
نمط استشهاد الجمعية الأمريكية للغات الحديثة (MLA)
Han, Baokun…[et al.]. Imbalanced Fault Classification of Bearing via Wasserstein Generative Adversarial Networks with Gradient Penalty. Shock and Vibration No. 2020 (2020), pp.1-14.
https://search.emarefa.net/detail/BIM-1212826
نمط استشهاد الجمعية الطبية الأمريكية (AMA)
Han, Baokun& Jia, Sixiang& Liu, Guifang& Wang, Jinrui. Imbalanced Fault Classification of Bearing via Wasserstein Generative Adversarial Networks with Gradient Penalty. Shock and Vibration. 2020. Vol. 2020, no. 2020, pp.1-14.
https://search.emarefa.net/detail/BIM-1212826
نوع البيانات
مقالات
لغة النص
الإنجليزية
الملاحظات
Includes bibliographical references
رقم السجل
BIM-1212826
قاعدة معامل التأثير والاستشهادات المرجعية العربي "ارسيف Arcif"
أضخم قاعدة بيانات عربية للاستشهادات المرجعية للمجلات العلمية المحكمة الصادرة في العالم العربي
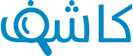
تقوم هذه الخدمة بالتحقق من التشابه أو الانتحال في الأبحاث والمقالات العلمية والأطروحات الجامعية والكتب والأبحاث باللغة العربية، وتحديد درجة التشابه أو أصالة الأعمال البحثية وحماية ملكيتها الفكرية. تعرف اكثر
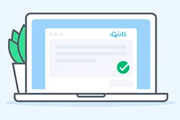