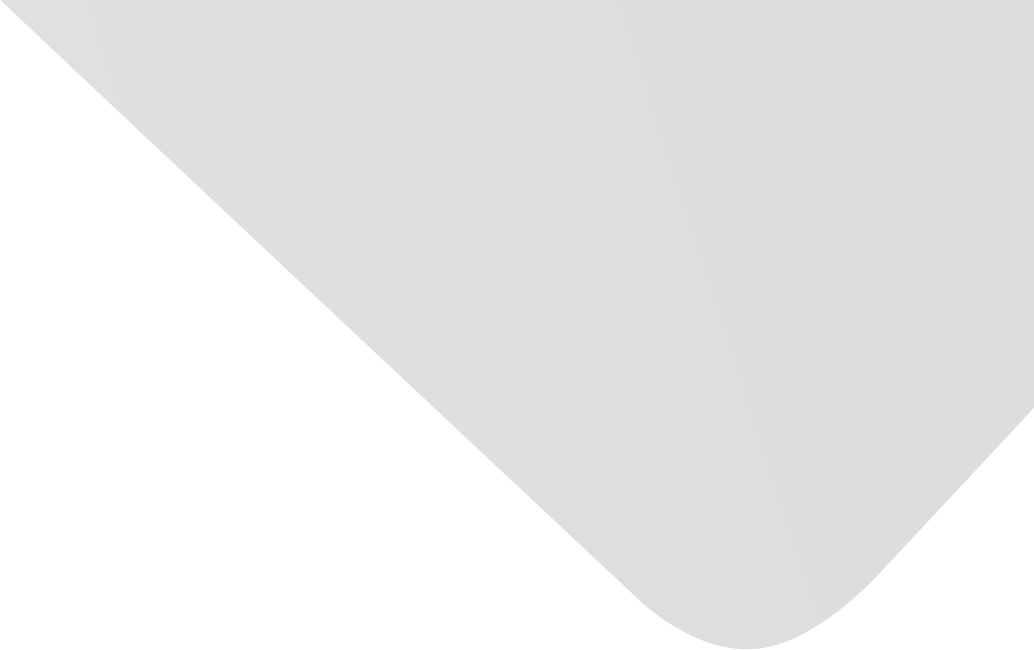
Rolling Bearing Fault Diagnosis Using a Deep Convolutional Autoencoding Network and Improved Gustafson–Kessel Clustering
المؤلفون المشاركون
Deng, Linfeng
Jin, Wuyin
Wu, Yaochun
Zhao, Rongzhen
He, Tianjing
Ma, Sencai
المصدر
العدد
المجلد 2020، العدد 2020 (31 ديسمبر/كانون الأول 2020)، ص ص. 1-17، 17ص.
الناشر
Hindawi Publishing Corporation
تاريخ النشر
2020-10-19
دولة النشر
مصر
عدد الصفحات
17
التخصصات الرئيسية
الملخص EN
Deep learning (DL) has been successfully used in fault diagnosis.
Training deep neural networks, such as convolutional neural networks (CNNs), require plenty of labeled samples.
However, in mechanical fault diagnosis, labeled data are costly and time-consuming to collect.
A novel method based on a deep convolutional autoencoding network (DCAEN) and adaptive nonparametric weighted-feature extraction Gustafson–Kessel (ANW-GK) clustering algorithm was developed for the fault diagnosis of bearings.
First, the DCAEN that is pretrained layer by layer by unlabeled samples and fine-tuned by a few labeled samples is applied to learn representative features from the vibration signals.
Then, the learned representative features are reduced by t-distributed stochastic neighbor embedding (t-SNE), and the low-dimensional main features are obtained.
Finally, the low-dimensional features are input ANW-GK clustering for fault identification.
Two datasets were used to validate the effectiveness of the proposed method.
The experimental results show that the proposed method can effectively diagnose different fault types with only a few labeled samples.
نمط استشهاد جمعية علماء النفس الأمريكية (APA)
Wu, Yaochun& Zhao, Rongzhen& Jin, Wuyin& Deng, Linfeng& He, Tianjing& Ma, Sencai. 2020. Rolling Bearing Fault Diagnosis Using a Deep Convolutional Autoencoding Network and Improved Gustafson–Kessel Clustering. Shock and Vibration،Vol. 2020, no. 2020, pp.1-17.
https://search.emarefa.net/detail/BIM-1212889
نمط استشهاد الجمعية الأمريكية للغات الحديثة (MLA)
Wu, Yaochun…[et al.]. Rolling Bearing Fault Diagnosis Using a Deep Convolutional Autoencoding Network and Improved Gustafson–Kessel Clustering. Shock and Vibration No. 2020 (2020), pp.1-17.
https://search.emarefa.net/detail/BIM-1212889
نمط استشهاد الجمعية الطبية الأمريكية (AMA)
Wu, Yaochun& Zhao, Rongzhen& Jin, Wuyin& Deng, Linfeng& He, Tianjing& Ma, Sencai. Rolling Bearing Fault Diagnosis Using a Deep Convolutional Autoencoding Network and Improved Gustafson–Kessel Clustering. Shock and Vibration. 2020. Vol. 2020, no. 2020, pp.1-17.
https://search.emarefa.net/detail/BIM-1212889
نوع البيانات
مقالات
لغة النص
الإنجليزية
الملاحظات
Includes bibliographical references
رقم السجل
BIM-1212889
قاعدة معامل التأثير والاستشهادات المرجعية العربي "ارسيف Arcif"
أضخم قاعدة بيانات عربية للاستشهادات المرجعية للمجلات العلمية المحكمة الصادرة في العالم العربي
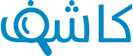
تقوم هذه الخدمة بالتحقق من التشابه أو الانتحال في الأبحاث والمقالات العلمية والأطروحات الجامعية والكتب والأبحاث باللغة العربية، وتحديد درجة التشابه أو أصالة الأعمال البحثية وحماية ملكيتها الفكرية. تعرف اكثر
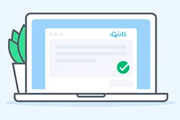