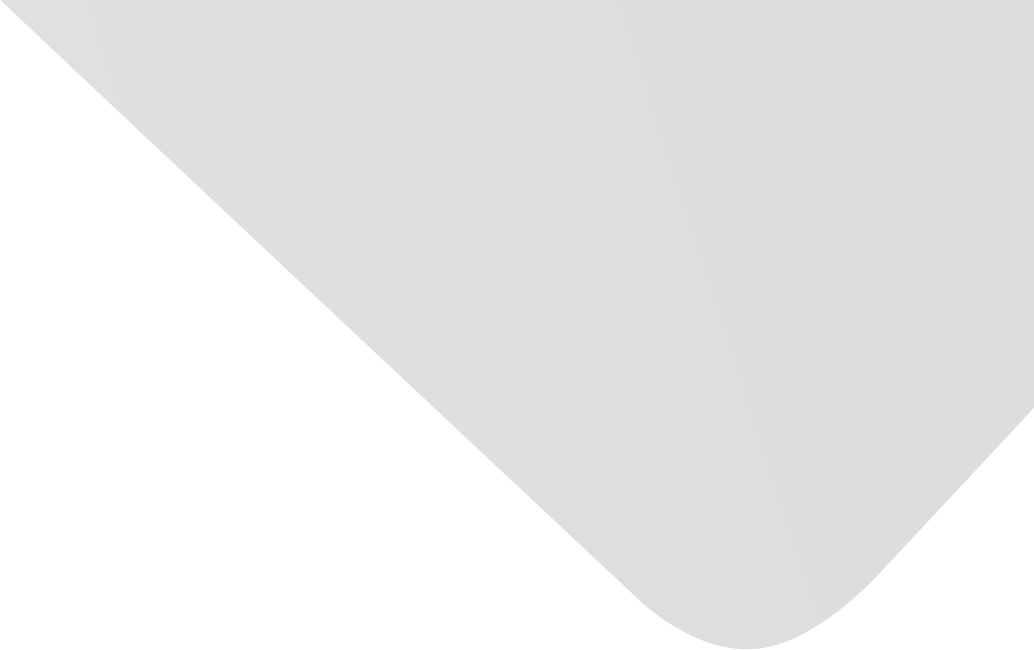
TR-IDS: Anomaly-Based Intrusion Detection through Text-Convolutional Neural Network and Random Forest
المؤلفون المشاركون
Liu, Qiang
Long, Jun
Min, Erxue
Cui, Jianjing
Chen, Wei
المصدر
Security and Communication Networks
العدد
المجلد 2018، العدد 2018 (31 ديسمبر/كانون الأول 2018)، ص ص. 1-9، 9ص.
الناشر
Hindawi Publishing Corporation
تاريخ النشر
2018-07-05
دولة النشر
مصر
عدد الصفحات
9
التخصصات الرئيسية
تكنولوجيا المعلومات وعلم الحاسوب
الملخص EN
As we head towards the IoT (Internet of Things) era, protecting network infrastructures and information security has become increasingly crucial.
In recent years, Anomaly-Based Network Intrusion Detection Systems (ANIDSs) have gained extensive attention for their capability of detecting novel attacks.
However, most ANIDSs focus on packet header information and omit the valuable information in payloads, despite the fact that payload-based attacks have become ubiquitous.
In this paper, we propose a novel intrusion detection system named TR-IDS, which takes advantage of both statistical features and payload features.
Word embedding and text-convolutional neural network (Text-CNN) are applied to extract effective information from payloads.
After that, the sophisticated random forest algorithm is performed on the combination of statistical features and payload features.
Extensive experimental evaluations demonstrate the effectiveness of the proposed methods.
نمط استشهاد جمعية علماء النفس الأمريكية (APA)
Min, Erxue& Long, Jun& Liu, Qiang& Cui, Jianjing& Chen, Wei. 2018. TR-IDS: Anomaly-Based Intrusion Detection through Text-Convolutional Neural Network and Random Forest. Security and Communication Networks،Vol. 2018, no. 2018, pp.1-9.
https://search.emarefa.net/detail/BIM-1214172
نمط استشهاد الجمعية الأمريكية للغات الحديثة (MLA)
Min, Erxue…[et al.]. TR-IDS: Anomaly-Based Intrusion Detection through Text-Convolutional Neural Network and Random Forest. Security and Communication Networks No. 2018 (2018), pp.1-9.
https://search.emarefa.net/detail/BIM-1214172
نمط استشهاد الجمعية الطبية الأمريكية (AMA)
Min, Erxue& Long, Jun& Liu, Qiang& Cui, Jianjing& Chen, Wei. TR-IDS: Anomaly-Based Intrusion Detection through Text-Convolutional Neural Network and Random Forest. Security and Communication Networks. 2018. Vol. 2018, no. 2018, pp.1-9.
https://search.emarefa.net/detail/BIM-1214172
نوع البيانات
مقالات
لغة النص
الإنجليزية
الملاحظات
Includes bibliographical references
رقم السجل
BIM-1214172
قاعدة معامل التأثير والاستشهادات المرجعية العربي "ارسيف Arcif"
أضخم قاعدة بيانات عربية للاستشهادات المرجعية للمجلات العلمية المحكمة الصادرة في العالم العربي
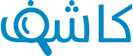
تقوم هذه الخدمة بالتحقق من التشابه أو الانتحال في الأبحاث والمقالات العلمية والأطروحات الجامعية والكتب والأبحاث باللغة العربية، وتحديد درجة التشابه أو أصالة الأعمال البحثية وحماية ملكيتها الفكرية. تعرف اكثر
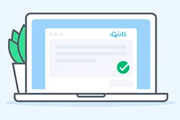