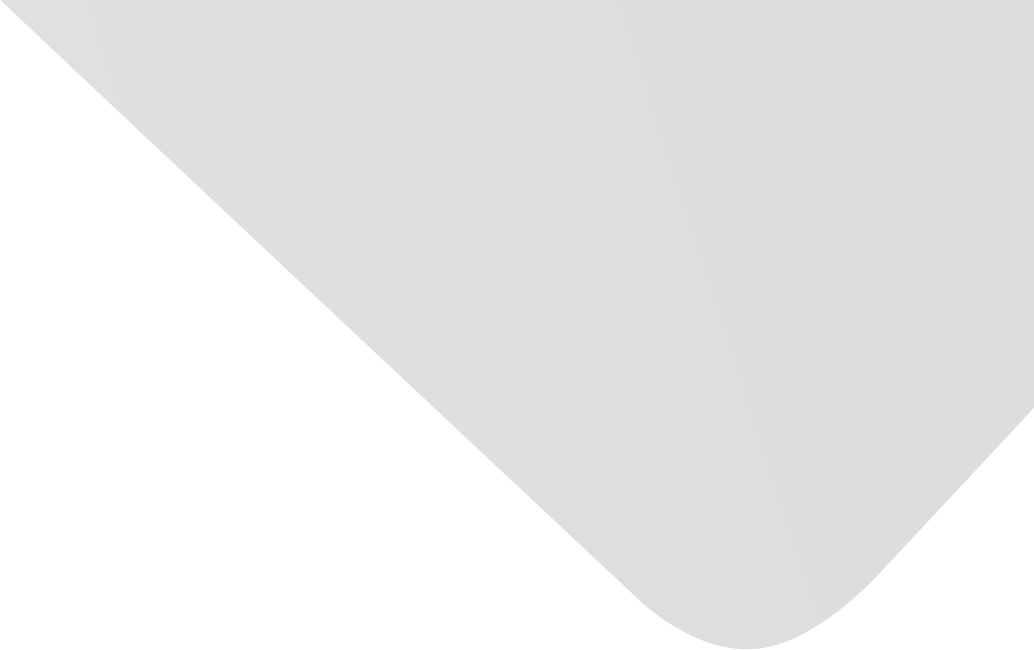
MFCFSiam: A Correlation-Filter-Guided Siamese Network with Multifeature for Visual Tracking
المؤلفون المشاركون
Li, Chenpu
Xing, Qianjian
Ma, Zhenguo
Zang, Ke
المصدر
Wireless Communications and Mobile Computing
العدد
المجلد 2020، العدد 2020 (31 ديسمبر/كانون الأول 2020)، ص ص. 1-19، 19ص.
الناشر
Hindawi Publishing Corporation
تاريخ النشر
2020-12-24
دولة النشر
مصر
عدد الصفحات
19
التخصصات الرئيسية
تكنولوجيا المعلومات وعلم الحاسوب
الملخص EN
With the development of deep learning, trackers based on convolutional neural networks (CNNs) have made significant achievements in visual tracking over the years.
The fully connected Siamese network (SiamFC) is a typical representation of those trackers.
SiamFC designs a two-branch architecture of a CNN and models’ visual tracking as a general similarity-learning problem.
However, the feature maps it uses for visual tracking are only from the last layer of the CNN.
Those features contain high-level semantic information but lack sufficiently detailed texture information.
This means that the SiamFC tracker tends to drift when there are other same-category objects or when the contrast between the target and the background is very low.
Focusing on addressing this problem, we design a novel tracking algorithm that combines a correlation filter tracker and the SiamFC tracker into one framework.
In this framework, the correlation filter tracker can use the Histograms of Oriented Gradients (HOG) and color name (CN) features to guide the SiamFC tracker.
This framework also contains an evaluation criterion which we design to evaluate the tracking result of the two trackers.
If this criterion finds the SiamFC tracker fails in some cases, our framework will use the tracking result from the correlation filter tracker to correct the SiamFC.
In this way, the defects of SiamFC’s high-level semantic features are remedied by the HOG and CN features.
So, our algorithm provides a framework which combines two trackers together and makes them complement each other in visual tracking.
And to the best of our knowledge, our algorithm is also the first one which designs an evaluation criterion using correlation filter and zero padding to evaluate the tracking result.
Comprehensive experiments are conducted on the Online Tracking Benchmark (OTB), Temple Color (TC128), Benchmark for UAV Tracking (UAV-123), and Visual Object Tracking (VOT) Benchmark.
The results show that our algorithm achieves quite a competitive performance when compared with the baseline tracker and several other state-of-the-art trackers.
نمط استشهاد جمعية علماء النفس الأمريكية (APA)
Li, Chenpu& Xing, Qianjian& Ma, Zhenguo& Zang, Ke. 2020. MFCFSiam: A Correlation-Filter-Guided Siamese Network with Multifeature for Visual Tracking. Wireless Communications and Mobile Computing،Vol. 2020, no. 2020, pp.1-19.
https://search.emarefa.net/detail/BIM-1214487
نمط استشهاد الجمعية الأمريكية للغات الحديثة (MLA)
Li, Chenpu…[et al.]. MFCFSiam: A Correlation-Filter-Guided Siamese Network with Multifeature for Visual Tracking. Wireless Communications and Mobile Computing No. 2020 (2020), pp.1-19.
https://search.emarefa.net/detail/BIM-1214487
نمط استشهاد الجمعية الطبية الأمريكية (AMA)
Li, Chenpu& Xing, Qianjian& Ma, Zhenguo& Zang, Ke. MFCFSiam: A Correlation-Filter-Guided Siamese Network with Multifeature for Visual Tracking. Wireless Communications and Mobile Computing. 2020. Vol. 2020, no. 2020, pp.1-19.
https://search.emarefa.net/detail/BIM-1214487
نوع البيانات
مقالات
لغة النص
الإنجليزية
الملاحظات
Includes bibliographical references
رقم السجل
BIM-1214487
قاعدة معامل التأثير والاستشهادات المرجعية العربي "ارسيف Arcif"
أضخم قاعدة بيانات عربية للاستشهادات المرجعية للمجلات العلمية المحكمة الصادرة في العالم العربي
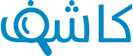
تقوم هذه الخدمة بالتحقق من التشابه أو الانتحال في الأبحاث والمقالات العلمية والأطروحات الجامعية والكتب والأبحاث باللغة العربية، وتحديد درجة التشابه أو أصالة الأعمال البحثية وحماية ملكيتها الفكرية. تعرف اكثر
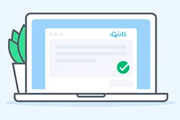