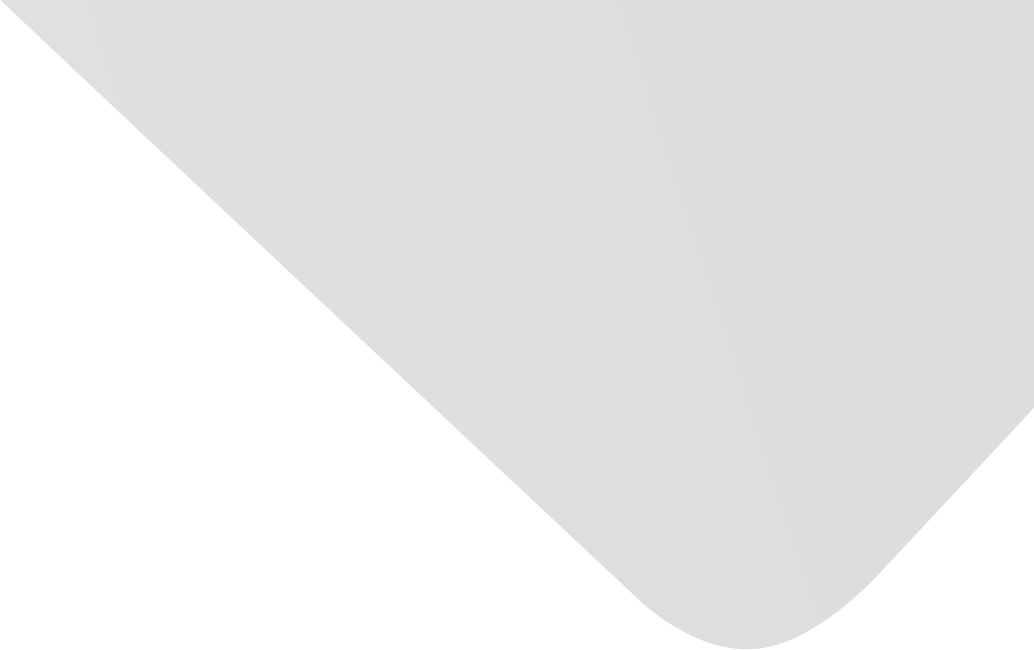
On the use of multiple instance learning for data classification
المؤلفون المشاركون
Salih, Nibras Z.
Khalaf, Wala Muhammad Hasan
المصدر
Journal of Engineering and Sustainable Development
العدد
المجلد 25، العدد (s) (31 ديسمبر/كانون الأول 2021)، ص ص. 127-137، 11ص.
الناشر
الجامعة المستنصرية كلية الهندسة
تاريخ النشر
2021-12-31
دولة النشر
العراق
عدد الصفحات
11
التخصصات الرئيسية
تكنولوجيا المعلومات وعلم الحاسوب
الموضوعات
الملخص EN
In the multiple instances learning framework, instances are arranged into bags, each bag contains several instances, the labels of each instance are not available but the label is available for each bag.
Whilst in a single instance learning each instance is connected with the label that contains a single feature vector.
This paper examines the distinction between these paradigms to see if it is appropriate, to cast the problem within a multiple instance framework.
In single-instance learning, two datasets are applied (students' dataset and iris dataset) using Naïve Bayes Classifier (NBC), Multilayer perceptron (MLP), Support Vector Machine (SVM), and Sequential Minimal Optimization (SMO), while SimpleMI, MIWrapper, and MIBoost in multiple instances learning.
Leave One Out Cross-Validation (LOOCV), five and ten folds Cross-Validation techniques (5-CV, 10-CV) are implemented to evaluate the classification results.
A comparison of the result of these techniques is made, several algorithms are found to be more effective for classification in the multiple instances learning.
The suitable algorithms for the students' dataset are MIBoost with MLP for LOOCV with an accuracy of 75% , whereas SimpleMI with SMO for the iris dataset is the suitable algorithm for 10-CV with an accuracy of In the multiple instances learning framework, instances are arranged into bags, each bag contains several instances, the labels of each instance are not available but the label is available for each bag.
Whilst in a single instance learning each instance is connected with the label that contains a single feature vector.
This paper examines the distinction between these paradigms to see if it is appropriate, to cast the problem within a multiple instance framework.
In single-instance learning, two datasets are applied (students' dataset and iris dataset) using Naïve Bayes Classifier (NBC), Multilayer perceptron (MLP), Support Vector Machine (SVM), and Sequential Minimal Optimization (SMO), while SimpleMI, MIWrapper, and MIBoost in multiple instances learning.
Leave One Out Cross-Validation (LOOCV), five and ten folds Cross-Validation techniques (5-CV, 10-CV) are implemented to evaluate the classification results.
A comparison of the result of these techniques is made, several algorithms are found to be more effective for classification in the multiple instances learning.
The suitable algorithms for the students' dataset are MIBoost with MLP for LOOCV with an accuracy of 75% , whereas SimpleMI with SMO for the iris dataset is the suitable algorithm for 10-CV with an accuracy of 99.33% .
نمط استشهاد جمعية علماء النفس الأمريكية (APA)
Salih, Nibras Z.& Khalaf, Wala Muhammad Hasan. 2021. On the use of multiple instance learning for data classification. Journal of Engineering and Sustainable Development،Vol. 25, no. (s), pp.127-137.
https://search.emarefa.net/detail/BIM-1273094
نمط استشهاد الجمعية الأمريكية للغات الحديثة (MLA)
Salih, Nibras Z.& Khalaf, Wala Muhammad Hasan. On the use of multiple instance learning for data classification. Journal of Engineering and Sustainable Development Vol. 25, Special issue (2021), pp.127-137.
https://search.emarefa.net/detail/BIM-1273094
نمط استشهاد الجمعية الطبية الأمريكية (AMA)
Salih, Nibras Z.& Khalaf, Wala Muhammad Hasan. On the use of multiple instance learning for data classification. Journal of Engineering and Sustainable Development. 2021. Vol. 25, no. (s), pp.127-137.
https://search.emarefa.net/detail/BIM-1273094
نوع البيانات
مقالات
لغة النص
الإنجليزية
الملاحظات
-
رقم السجل
BIM-1273094
قاعدة معامل التأثير والاستشهادات المرجعية العربي "ارسيف Arcif"
أضخم قاعدة بيانات عربية للاستشهادات المرجعية للمجلات العلمية المحكمة الصادرة في العالم العربي
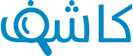
تقوم هذه الخدمة بالتحقق من التشابه أو الانتحال في الأبحاث والمقالات العلمية والأطروحات الجامعية والكتب والأبحاث باللغة العربية، وتحديد درجة التشابه أو أصالة الأعمال البحثية وحماية ملكيتها الفكرية. تعرف اكثر
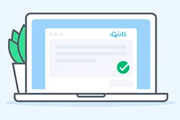