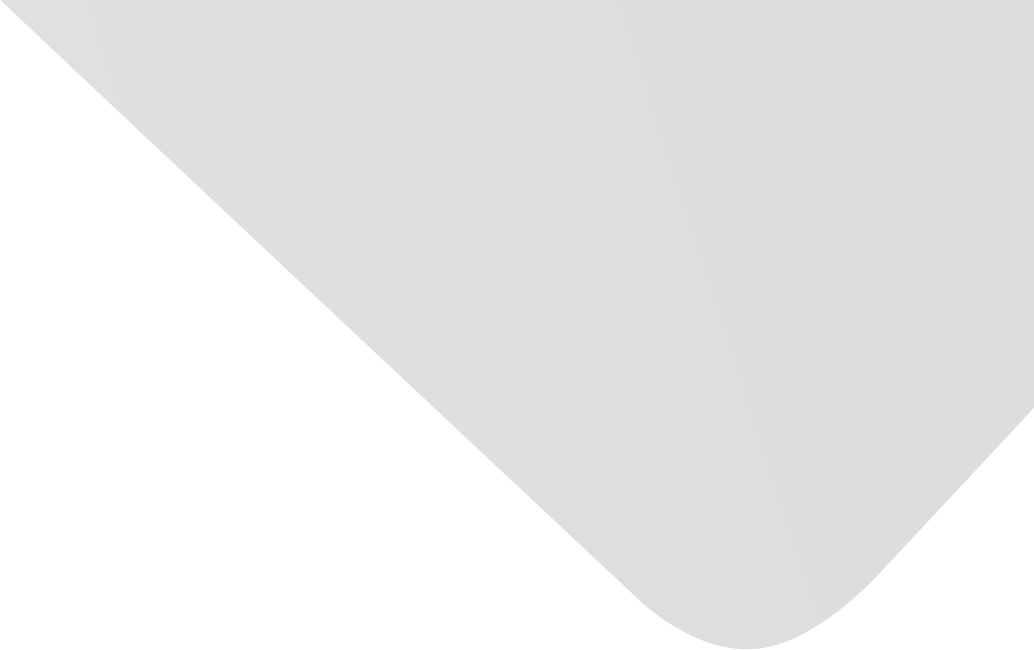
Off-line signature confirmation based on cluster representations of geometrical and statistical features through vector distance, neural network and support vector machine classifiers
المؤلفون المشاركون
Ventakaramu, Aravinda Chikmagalur
Devaraj, Suresha
Nanjundaiah, Prakash Hebbakavadi
Rajasekhar, Kyasambally
المصدر
The International Arab Journal of Information Technology
العدد
المجلد 19، العدد 4 (31 يوليو/تموز 2022)، ص ص. 667-673، 7ص.
الناشر
جامعة الزرقاء عمادة البحث العلمي
تاريخ النشر
2022-07-31
دولة النشر
الأردن
عدد الصفحات
7
التخصصات الرئيسية
تكنولوجيا المعلومات وعلم الحاسوب
الملخص EN
We exploited the geometrical and statistical properties of signature images for offline signature verification and identification in this paper, using signature clustering and classification based on extracted features.
The Offline-SVR has been tested on the 2004 Ministerio de Ciencia Tecnología e Innovación (MCTYTDB) OffLineSignSubCorpus dataset, the MCYT-330 online signature dataset, and the MCE-200 dataset, which together are referred to as the MCE-605 dataset.
Using a standard data set for experiments, the results of the Vector Distance (VD), Support Vector Machine (SVM) and Neural Network (NN) methods are significantly superior to those of other signature verification and recognition methods.
Moreover, the VD method performed better than The SVM and NN methods.
The purpose of the study is on clustering signature images using geometric and statistical features, as well as the utilization vector distance, neural networks, and support vector machines for signature image verification and identification.
It was decided to use the algorithm for developing geometric and statistical features.
The signature images are classified using generated features using k-means clustering, and Offline and Online- Support Vector Regression (SVR) is accomplished using VD, SVM, and NN training and classification with a different number of signatures each time, preceded by verification using recognition statistics.
Because of the minimal number of features, the designed mechanism seems to be much faster.
Experimenting on a standard dataset reveals that the results obtained from clustering signatures and categorization are effective and simple in comparison to other Offline signature confirmation systems.
In this research work, we address the problem of representing handwritten signatures (online/offline) suitable for effective verification and recognition.
We propose effective feature extraction for verification and recognition of signatures.
نمط استشهاد جمعية علماء النفس الأمريكية (APA)
Ventakaramu, Aravinda Chikmagalur& Devaraj, Suresha& Nanjundaiah, Prakash Hebbakavadi& Rajasekhar, Kyasambally. 2022. Off-line signature confirmation based on cluster representations of geometrical and statistical features through vector distance, neural network and support vector machine classifiers. The International Arab Journal of Information Technology،Vol. 19, no. 4, pp.667-673.
https://search.emarefa.net/detail/BIM-1437338
نمط استشهاد الجمعية الأمريكية للغات الحديثة (MLA)
Ventakaramu, Aravinda Chikmagalur…[et al.]. Off-line signature confirmation based on cluster representations of geometrical and statistical features through vector distance, neural network and support vector machine classifiers. The International Arab Journal of Information Technology Vol. 19, no. 4 (Jul. 2022), pp.667-673.
https://search.emarefa.net/detail/BIM-1437338
نمط استشهاد الجمعية الطبية الأمريكية (AMA)
Ventakaramu, Aravinda Chikmagalur& Devaraj, Suresha& Nanjundaiah, Prakash Hebbakavadi& Rajasekhar, Kyasambally. Off-line signature confirmation based on cluster representations of geometrical and statistical features through vector distance, neural network and support vector machine classifiers. The International Arab Journal of Information Technology. 2022. Vol. 19, no. 4, pp.667-673.
https://search.emarefa.net/detail/BIM-1437338
نوع البيانات
مقالات
لغة النص
الإنجليزية
الملاحظات
Includes bibliographical references : p. 671-672
رقم السجل
BIM-1437338
قاعدة معامل التأثير والاستشهادات المرجعية العربي "ارسيف Arcif"
أضخم قاعدة بيانات عربية للاستشهادات المرجعية للمجلات العلمية المحكمة الصادرة في العالم العربي
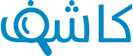
تقوم هذه الخدمة بالتحقق من التشابه أو الانتحال في الأبحاث والمقالات العلمية والأطروحات الجامعية والكتب والأبحاث باللغة العربية، وتحديد درجة التشابه أو أصالة الأعمال البحثية وحماية ملكيتها الفكرية. تعرف اكثر
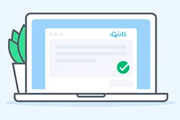