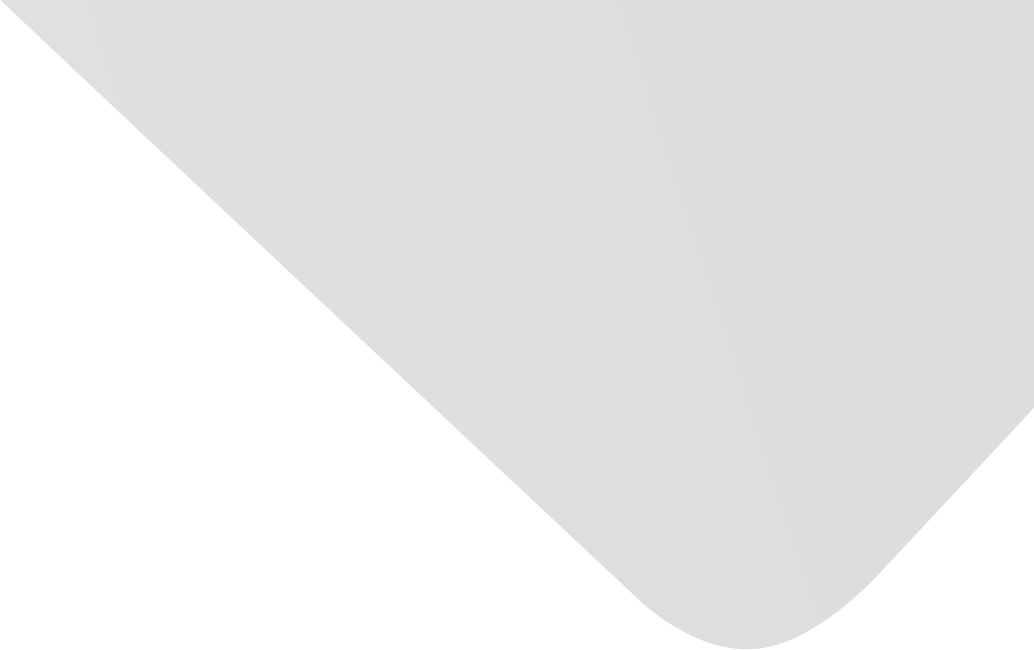
Exploring self-supervised pretraining datasets for complex scene understanding
المؤلفون المشاركون
Khattab, Dina
Kawashti, Yumna Ahmad
Arif, Mustafa M.
المصدر
International Journal of Intelligent Computing and Information Sciences
العدد
المجلد 23، العدد 2 (30 يونيو/حزيران 2023)، ص ص. 62-72، 11ص.
الناشر
جامعة عين شمس كلية الحاسبات و المعلومات
تاريخ النشر
2023-06-30
دولة النشر
مصر
عدد الصفحات
11
التخصصات الرئيسية
تكنولوجيا المعلومات وعلم الحاسوب
الموضوعات
الملخص EN
With the rapid advancements of deep learning research, there have been many milestones achieved in the field of computer vision.
however, most of these advances are only applicable in cases where hand-annotated datasets are available.
this is considered the current bottleneck of deep learning that self-supervised learning aims to overcome.
the self-supervised framework consists of proxy and target tasks.
the proxy task is a self-supervised task pretrained on unlabeled data, the weights of which are transferred to the target task.
The prevalent paradigm in self-supervised research is to pretrain using ImageNet which is a single-object centric dataset.
in this work, we investigate whether this is the best choice when the target task is multi-object centric.
we pretrain “SimSiam” which is a non-contrastive self-supervised algorithm using two different pretraining datasets : ImageNet100 (single-object centric) and COCO (multi-object centric).
the transfer performance of each pretrained model is evaluated on the target task of multi-label classification using PascalVOC.
furtherly, we evaluate the two pretrained models using CityScapes ; an autonomous driving dataset in order to study the implications of the chosen pretraining datasets in different domains.
our results showed that the SimSiam model pretrained using COCO consistently outperformed the ImageNet100 pretrained model by ~ + 1 percent (57.4 vs 58.3 mAP for CityScapes).
this is significant since COCO is smaller in size.
we conclude that using multi-object centric datasets for pretraining self-supervised learning algorithms is more efficient in cases where the target task is multi-object centric and in complex scene understanding tasks such as autonomous driving applications.
نمط استشهاد جمعية علماء النفس الأمريكية (APA)
Kawashti, Yumna Ahmad& Khattab, Dina& Arif, Mustafa M.. 2023. Exploring self-supervised pretraining datasets for complex scene understanding. International Journal of Intelligent Computing and Information Sciences،Vol. 23, no. 2, pp.62-72.
https://search.emarefa.net/detail/BIM-1495806
نمط استشهاد الجمعية الأمريكية للغات الحديثة (MLA)
Kawashti, Yumna Ahmad…[et al.]. Exploring self-supervised pretraining datasets for complex scene understanding. International Journal of Intelligent Computing and Information Sciences Vol. 23, no. 2 (Jun. 2023), pp.62-72.
https://search.emarefa.net/detail/BIM-1495806
نمط استشهاد الجمعية الطبية الأمريكية (AMA)
Kawashti, Yumna Ahmad& Khattab, Dina& Arif, Mustafa M.. Exploring self-supervised pretraining datasets for complex scene understanding. International Journal of Intelligent Computing and Information Sciences. 2023. Vol. 23, no. 2, pp.62-72.
https://search.emarefa.net/detail/BIM-1495806
نوع البيانات
مقالات
لغة النص
الإنجليزية
الملاحظات
Includes bibliographical references : p. 71-72
رقم السجل
BIM-1495806
قاعدة معامل التأثير والاستشهادات المرجعية العربي "ارسيف Arcif"
أضخم قاعدة بيانات عربية للاستشهادات المرجعية للمجلات العلمية المحكمة الصادرة في العالم العربي

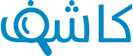
تقوم هذه الخدمة بالتحقق من التشابه أو الانتحال في الأبحاث والمقالات العلمية والأطروحات الجامعية والكتب والأبحاث باللغة العربية، وتحديد درجة التشابه أو أصالة الأعمال البحثية وحماية ملكيتها الفكرية. تعرف اكثر
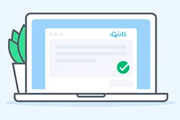