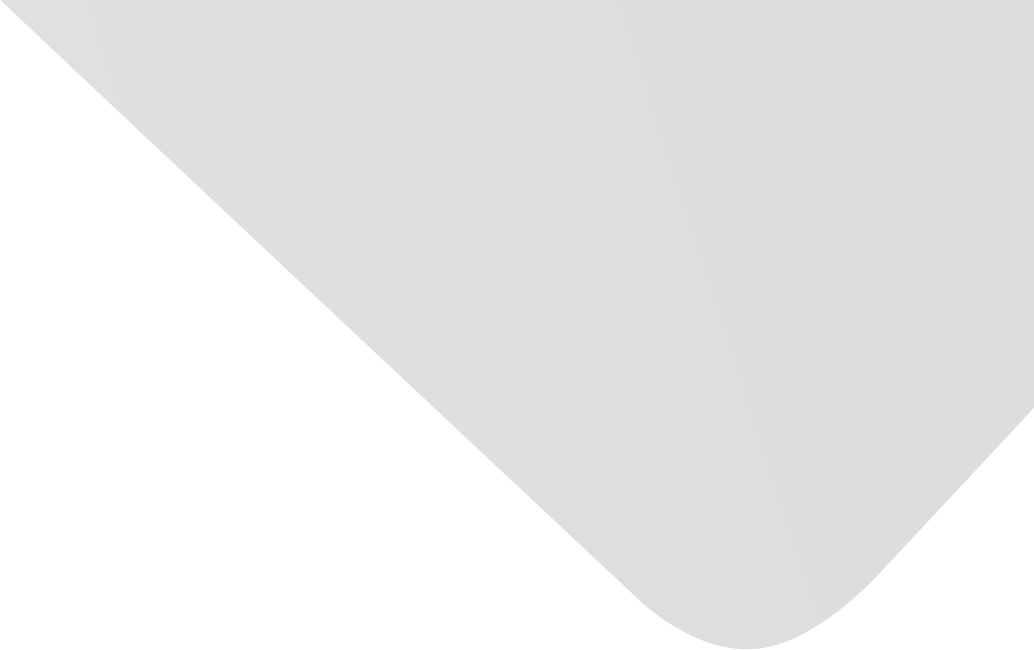
Machine learning for market volatility prediction
المؤلف
المصدر
Journal of Economics and Sustainable Development
العدد
المجلد 6، العدد 1 (31 مارس/آذار 2023)، ص ص. 680-701، 22ص.
الناشر
تاريخ النشر
2023-03-31
دولة النشر
الجزائر
عدد الصفحات
22
التخصصات الرئيسية
الموضوعات
الملخص AR
يعد توقع تقلبات السوق أحد أكثر المصطلحات شيوعا في سوق التداول اليوم.
يتم تمثيل تحركات الأسعار وتقلبات السوق ومخاطر التداول من خلال التقلبات المحققة.
يؤثر تغيير طفيف في التقلب على العائد المتوقع لجميع الأصول.
في هذا البحث للتنبؤ بالتقلبات، استخدمنا مجموعة البيانات التي توفرها منصة Kaggle Optiver، وهو صانع سوق إلكتروني عالمي رائد وملتزم بالتحسين المستمر للأسواق المالية وتحسين الوصول وأسعار الخيارات، والصناديق المتداولة في البورصة (ETFs)، في العديد من البورصات حول العالم، حيث يتم تداول الأسهم النقدية والسندات والعملات الأجنبية.
في دراستنا استخدمنا نماذج التنبؤ التالية: LightGBM،XGBoost ، CatBoost، Regression Linear، واستنتجنا بعض الأعمال ذات الصلة حول التنبؤ بالتقلب.
وبعد ذلك قمنا بتنفيذ النماذج.
تظهر النتائج أن نموذج LightGBM هو الأفضل من بين هذه النماذج، حيث حقق أدنى درجة جذر متوسط مربع النسبة المئوية لفقدان الخطأ (RMSPE) وهي: 286.0 وأعلى درجة في معامل التحديد R2 هي: 817.0، وأن RMSPE للنماذج الأخرى: XGBoost، CatBoost، Linear، 0.791: أيضا التوالي على هي لها r2 ودرجة، 0.347، 0.302، 0.303: التوالي على هي Regression 784.0،766.0.
نستخلص أن نموذج lightGBM هو النموذج الأفضل مقارنة بالنماذج XGBoost، CatBoost، Regression Linear في توقع تقلبات سوق الأسهم.
الملخص EN
Market volatility prediction is one of the most commonly used terms in the trading market today.
price movements, market volatility, and trading risks are all represented by realized volatility.
a small change in volatility affects the expected return on all assets.
in this research, to predict volatility, we will use the dataset provided by the Kaggle platform.
Optiver is a leading global electronic market maker and is committed to continuously improving financial markets.
improving access and prices for options.
exchange traded funds(ETFs), on numerous exchanges around the world, cash equities, bonds, and foreign currencies are traded.
the prediction models we used in our study are Lightgbm and XGboost and Catboost and linear regression, and we concluded some related works on forecasting volatility.
then we ran our models.
the results show that the Lightgbm model is the best among these models, as it achieved the lowest root mean square error percentage (RMSPE) score of: 0.286, and the highest score in the coefficient of determination R2 is: 0.817, and the RMSPE for other models: XGboost, Catboost and linear regression is, respectively: 0.303, 0.302 and 0.347, and the score of r2 is also: 0.791, 0.784, 0.766.
نمط استشهاد جمعية علماء النفس الأمريكية (APA)
Ata Allah, Ammur. 2023. Machine learning for market volatility prediction. Journal of Economics and Sustainable Development،Vol. 6, no. 1, pp.680-701.
https://search.emarefa.net/detail/BIM-1511234
نمط استشهاد الجمعية الأمريكية للغات الحديثة (MLA)
Ata Allah, Ammur. Machine learning for market volatility prediction. Journal of Economics and Sustainable Development Vol. 6, no. 1 (Mar. 2023), pp.680-701.
https://search.emarefa.net/detail/BIM-1511234
نمط استشهاد الجمعية الطبية الأمريكية (AMA)
Ata Allah, Ammur. Machine learning for market volatility prediction. Journal of Economics and Sustainable Development. 2023. Vol. 6, no. 1, pp.680-701.
https://search.emarefa.net/detail/BIM-1511234
نوع البيانات
مقالات
لغة النص
الإنجليزية
الملاحظات
Includes bibliographical references: p. 700-701
رقم السجل
BIM-1511234
قاعدة معامل التأثير والاستشهادات المرجعية العربي "ارسيف Arcif"
أضخم قاعدة بيانات عربية للاستشهادات المرجعية للمجلات العلمية المحكمة الصادرة في العالم العربي
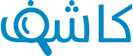
تقوم هذه الخدمة بالتحقق من التشابه أو الانتحال في الأبحاث والمقالات العلمية والأطروحات الجامعية والكتب والأبحاث باللغة العربية، وتحديد درجة التشابه أو أصالة الأعمال البحثية وحماية ملكيتها الفكرية. تعرف اكثر
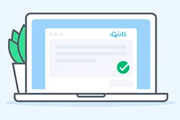