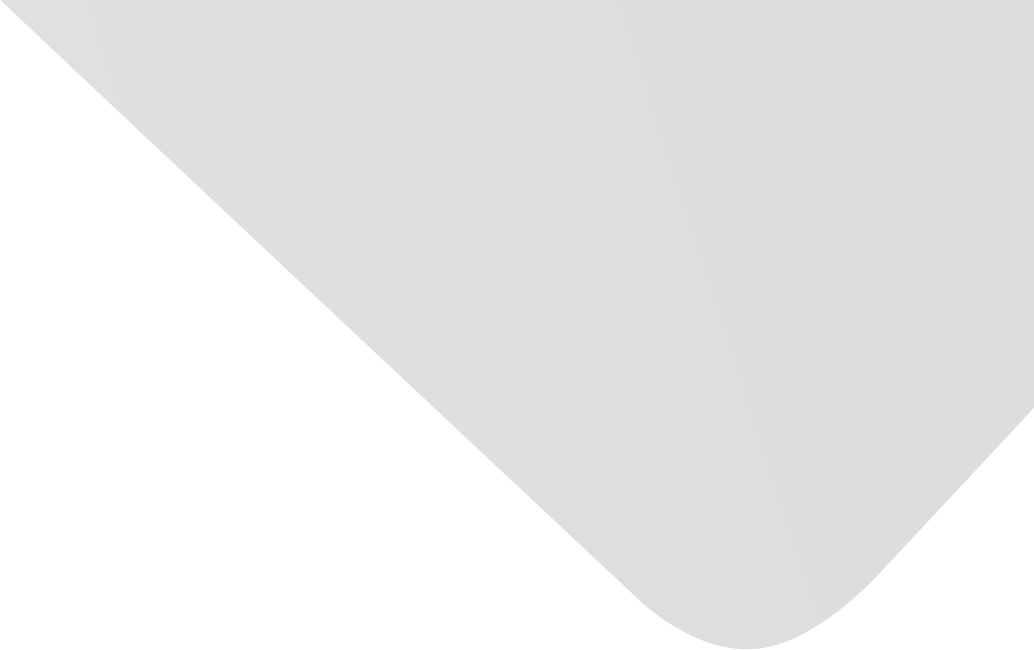
The impact of Arabic dialects on the performance of Arabic speech recognition systems
العناوين الأخرى
تأثير بعض اللهجات العربية على التعرف الآلي على الكلام باللغة العربية
مقدم أطروحة جامعية
مشرف أطروحة جامعية
الجامعة
جامعة بيرزيت
الكلية
كلية الهندسة و التكنولوجيا
القسم الأكاديمي
دائرة علم الحاسوب
دولة الجامعة
فلسطين (الضفة الغربية)
الدرجة العلمية
ماجستير
تاريخ الدرجة العلمية
2019
الملخص العربي
بعد التعرف التلقائي على الكلام ASR جوهر الاهتمام لعظم التطبيقات الحديثة، مثل البحث الصوتي، وإملاء الرسائل القصيرة وغيرها.
واحدة من التحديات التي تواجه عملية التعرف على الكلام هي الاختلافات في الكلام البشري، وهذا يرجع إلى العديد من العوامل مثل العمر والجنس والجنسية ومستوى التعليم.
عموما تعدد اللغات اللهجات وطريقة النطق لها تأثير كبير أيضا.
تضيف اللغة العربية تحديات أكبر من أي لغة أخرى، ويرجع ذلك إلى الفرق الكبير بين اللغة العربية الفصحى المعاصرة واللهجات الإقليمية في الدول العربية.
في هذا البحث، ندرس تأثير اللهجات العربية على أداء التعرف التلقائي للغة العربية.
يتم ذلك من خلال استخدام أساليب مختلفة للتكيف والتحسين في النموذج الصوتي.
لقد وجدنا أن استخدام أحدث تقنية للشبكة العصبية العميقة HMM DNN أدى إلى تحسين أداء التعرف التلقائي على نموذج المعياري التقليدي HMM-GMM.
وتحصل على أفضل أداء عندما يكون لدينا HMM DNN بخمس طبقات مخفية، وأبعاد ٢٠٤٨ مخفية، وتحسين الحد الأدنى للخطأ الصوتي MPE، واستخدام MFCC متجه حامل خصائص المقطع الصوتي.
وعندما نضيف DNN إلى مرحلة استخراج الميزات للحصول على Bottleneck feature، تحصل على أداء أفضل من استخدام MFCC.
يجب أن نعرف أنه في مرحلة استخراج الميزات، قمنا بتقليل حجم الإطار إلى ٢٠ مللي ثانية وحافظنا على تحول التنقل الزمني مساويا ل ١٠ مللي ثانية.
هذا الإجراء يزيد من التداخل بين الإطارات ويقلل من فقدان البيانات.
أيضا في هذا البحث، بدأنا بتعرف تلقائي معتمد على لهجة معينة، وقد استخدمنا أربعة أحجام مختلفة من مجموعات البيانات تتراوح بين ۲۰۰۰ و٥٠٠٠.
وجدنا أن زيادة حجم بيانات التدريب يعزز أداء التعرف التلقائي.
والأهم من ذلك هو إضافة اللهجة العربية إلى مجموعة بيانات التدريب مما يعزز الأداء كذلك.
قارنا أداء مجموعتين من مجموعات البيانات: الأولى تحتوي على بيانات من اللغة العربية الفصحى وحجمها ٥٠٠٠٠ جملة والثانية عبارة عن مزيج من لهجة عربية واللغة العربية الفصحى وحجمها ٤٠٠٠٠ حملة.
وكانت النتيجة أننا حصلنا على أداء أفضل أثناء إضافة اللهجة إلى التدريب، وهذا يعني أن اختيار البيانات ونوعها البيانات التدريب مهم أيضا لتحسين أداء التعرف الآلي للغة العربية وليس فقط حجم مجموعة بيانات التدريب.
ثم فكرنا في مزج جميع اللهجات أثناء التدريب على التعرف الآلي للحصول على نموذج مستقل عن أبي لهجة عربية.
وكان أداء النموذج المستقل أفضل من النموذج المعتمد على لهجة معينة.
ويعزى ذلك الحجم بيانات التدريب للهجات العربية.
في هذا البحث أيضا حاولنا المقارنة بين أدوات المواءمة من خلال تجارب مختلفة، ووجدنا أن FMLLR وننغ باغ يتصرفان بنفس الكفاءة أثناء استخدام DD-ASR أي في حالة التدريب على لهجة واحدة فقط.
ولكن في حالة ID-ASR أي في حالة التدرب على أكثر من لهجة، كان أداء MAP أفضل من MLLR.
كما وتظهر نتائجنا أن استخدام تقنية الشبكات العميقة في أي مرحلة من مراحل التعرف الآلي على الأصوات يعزز أداء التعرف الآلي على الأصوات باللغة العربية نظرا لأننا استخدمنا هذه التقنية لإنشاء متجه حامل خصائص الصوت واستخدمناها في مرحلة النموذج الصوتي.
الملخص الإنجليزي
Automatic Speech Recognition (ASR) is the core of interest for most recent applications, like voice search (VS), short message dictation (SMD) and others.
One of the challenges of the Speech Recognition (SR) process is human speech variations, this is due to many factors like age, gender, nationality and level of education.
Generally different languages, different dialects and pronunciation have a big effect as well.
Arabic language adds more challenges to SR than any other languages, this is due to the large difference between Modern Standard Arabic (MSA) and regional dialects in Arab countries.
In this research, we study the impact of Arabic dialects on the performance of Arabic ASR.
This is through using different adaptation and optimization techniques in the Acoustic Model (AM).
We find that using the state-of-art of Deep Neural Network (DNN) improved the performance of ASR over the traditional Hidden Markov model-Gaussian Mixture Model (HMM-GMM).
And we get the best performance when we have HMM-DNN with five hidden layers, 2048 hidden dimensions, Minimum Phone Error (MPE) optimization and Mel-Frequency Cepstral Coefficients (MFCC) as feature extraction.
But when we add DNN to the feature extraction stage to have Bottleneck features BNF, we get better performance than using MFCC.
We should know that in feature extraction phase, we reduced the frame size to 20 millisecond (ms) and kept the time shift equal to 10 ms.
This action increases the overlap between frames and reduces the data lose.
In our research, we start with Dependent Dialect ASR (DD-ASR), we used four different datasets sizes ranges between 2K to 50K utterances.
We found that increasing the size of training data enhances the performance of Arabic ASR.
And the most important is adding Arabic dialect to training dataset which enhances the performance as well.
We compare the 50K utterance dataset of Modern Spoken Arabic (MSA) only with the 40K utterance dataset of a mixture of Arabic dialect and MSA.
We get better performance while adding the dialect to training, this means that data selection of training data is also important to improve the performance of Arabic ASR despite the size of the training dataset size.
Then we thought of mixing all the dialects while training ASR to have Independent Dialect ASR (ID-ASR).
The performance of ID-ASR is better than DD-ASR.
For Language Model (LM) phase, We generate the LM using the text of the training dataset for each experiment.
So the size of training data affects the LM as well.
Also through our different experiments, we find that feature-space Maximum Likelihood Linear Regression (fMLLR) and Maximum A Posterior( MAP) act the same while using only one dialect trained ASR.
But when we train ASR on multiple dialects MAP has better performance than fMLLR.
Our results show that employing DNN technology in any phase of ASR enhances the performance of Arabic ASR.
As we used this technology to generate feature vector (Bottleneck features) and used it in the Acoustic Model phase.
التخصصات الرئيسية
تكنولوجيا المعلومات وعلم الحاسوب
الموضوعات
عدد الصفحات
100
قائمة المحتويات
Table of contents.
Abstract.
Abstract in Arabic.
Chapter one: Introduction.
Chapter two: Automatic speech recognition Asr-background.
Chapter three: Methodology and implementation.
Chapter four: Experiments and results.
Chapter five: Conclusions and future work.
References.
نمط استشهاد جمعية علماء النفس الأمريكية (APA)
Abu Gharibah, Amani. (2019). The impact of Arabic dialects on the performance of Arabic speech recognition systems. (Master's theses Theses and Dissertations Master). Birzeit University, Palestine (West Bank)
https://search.emarefa.net/detail/BIM-1520785
نمط استشهاد الجمعية الأمريكية للغات الحديثة (MLA)
Abu Gharibah, Amani. The impact of Arabic dialects on the performance of Arabic speech recognition systems. (Master's theses Theses and Dissertations Master). Birzeit University. (2019).
https://search.emarefa.net/detail/BIM-1520785
نمط استشهاد الجمعية الطبية الأمريكية (AMA)
Abu Gharibah, Amani. (2019). The impact of Arabic dialects on the performance of Arabic speech recognition systems. (Master's theses Theses and Dissertations Master). Birzeit University, Palestine (West Bank)
https://search.emarefa.net/detail/BIM-1520785
لغة النص
الإنجليزية
نوع البيانات
رسائل جامعية
رقم السجل
BIM-1520785
قاعدة معامل التأثير والاستشهادات المرجعية العربي "ارسيف Arcif"
أضخم قاعدة بيانات عربية للاستشهادات المرجعية للمجلات العلمية المحكمة الصادرة في العالم العربي
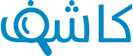
تقوم هذه الخدمة بالتحقق من التشابه أو الانتحال في الأبحاث والمقالات العلمية والأطروحات الجامعية والكتب والأبحاث باللغة العربية، وتحديد درجة التشابه أو أصالة الأعمال البحثية وحماية ملكيتها الفكرية. تعرف اكثر
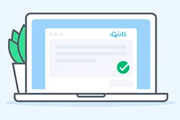