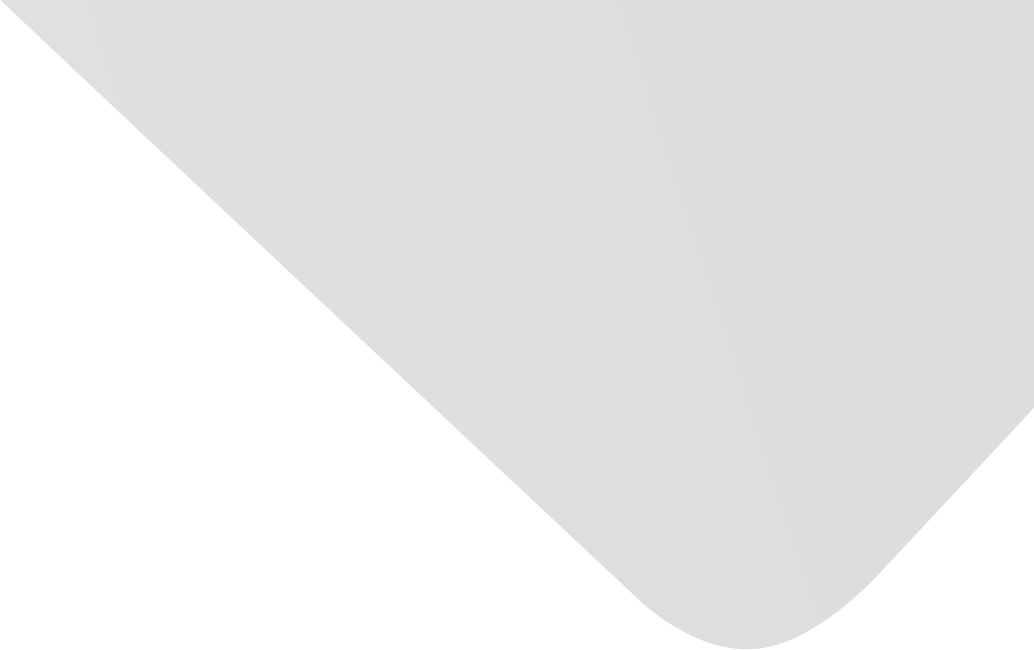
Comparison between features extraction techniques for impairments Arabic speech
العناوين الأخرى
مقارنة بين تقنيات استخلاص الميزات عند ضعف الكلام العربي
المؤلفون المشاركون
Muhammad, Yusra Faysal
Sharif, Sura Ramzi
المصدر
al-Rafidain Engineering Journal
العدد
المجلد 27، العدد 2 (30 سبتمبر/أيلول 2022)، ص ص. 190-197، 8ص.
الناشر
تاريخ النشر
2022-09-30
دولة النشر
العراق
عدد الصفحات
8
التخصصات الرئيسية
تكنولوجيا المعلومات وعلم الحاسوب
الموضوعات
الملخص AR
تعد عملية التعرف على الكلام التلقائي واحدة من المهام الصعبة، مع وجود ضوضاء مصاحبة في أغلب الأحيان للكلام وعدم القدرة على التنبؤ في الكلام المنطوق يؤدي إلى مشاكل حادة في عملية تحويل الكلمات المنطوقة إلى نص.
استخراج ميزات مقاومة للضوضاء لتعويض هذا التراجع في الأداء هو الأخر تحديا حتى السنوات القليلة الماضية.
هذا البحث يحقق في تأثير الميزات المختلفة المستخرجة من موجات الكلام.
ثم اختبرت هذه الميزات مع نوعين من تقنيات التعلم العميق هما الذاكرة طويلة المدى LSTM النموذج التقليدية ونموذج هجين يتضمن الشبكة العصبية التلافيفية ذات الذاكرة طويلة LSTM-CNN.
أظهرت نتائج هذا البحث أن ميزات MFCC أكثر مقاومة الضوضاء، حيث حققت أعلى دقة مع نموذج LSTM بنسبة 93٪ ومع الموديل الهجين LSTM-CNN كانت الدقة 91٪.
الملخص EN
Automatic speech recognition (ASR) is a tough task, with the existence of related noise and high unpredictability in a speech presenting the most severe problems.
especially with regard to the noise of speech impairments, whether due to disability or mispronunciation in children.
extraction of noise-resistant features to compensate for speech degradation due to noise impact has remained a difficult challenge in the last few years.
this research investigated the impact of different wavelet generations for extracting speech features, then test the produced dataset from each technique with two types of deep learning techniques: deep long short-term memory (LSTM) and hyper deep learning model convolutional neural network with long short-term memory (CNN-LSTM).
the result shows that the deep long short-term memory of MFCC has reached 93% as an accuracy while in the hyper deep learning model of CNN-LSTM the accuracy of MFCC was 91%, as the highest recorded accuracy which proves that MFCC would be the best feature extraction technique for our developed dataset.
نمط استشهاد جمعية علماء النفس الأمريكية (APA)
Sharif, Sura Ramzi& Muhammad, Yusra Faysal. 2022. Comparison between features extraction techniques for impairments Arabic speech. al-Rafidain Engineering Journal،Vol. 27, no. 2, pp.190-197.
https://search.emarefa.net/detail/BIM-1560152
نمط استشهاد الجمعية الأمريكية للغات الحديثة (MLA)
Sharif, Sura Ramzi& Muhammad, Yusra Faysal. Comparison between features extraction techniques for impairments Arabic speech. al-Rafidain Engineering Journal Vol. 27, no. 2 (Sep. 2022), pp.190-197.
https://search.emarefa.net/detail/BIM-1560152
نمط استشهاد الجمعية الطبية الأمريكية (AMA)
Sharif, Sura Ramzi& Muhammad, Yusra Faysal. Comparison between features extraction techniques for impairments Arabic speech. al-Rafidain Engineering Journal. 2022. Vol. 27, no. 2, pp.190-197.
https://search.emarefa.net/detail/BIM-1560152
نوع البيانات
مقالات
لغة النص
الإنجليزية
الملاحظات
Includes bibliographical references: p. 195-196
رقم السجل
BIM-1560152
قاعدة معامل التأثير والاستشهادات المرجعية العربي "ارسيف Arcif"
أضخم قاعدة بيانات عربية للاستشهادات المرجعية للمجلات العلمية المحكمة الصادرة في العالم العربي
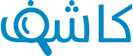
تقوم هذه الخدمة بالتحقق من التشابه أو الانتحال في الأبحاث والمقالات العلمية والأطروحات الجامعية والكتب والأبحاث باللغة العربية، وتحديد درجة التشابه أو أصالة الأعمال البحثية وحماية ملكيتها الفكرية. تعرف اكثر
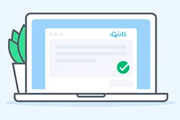