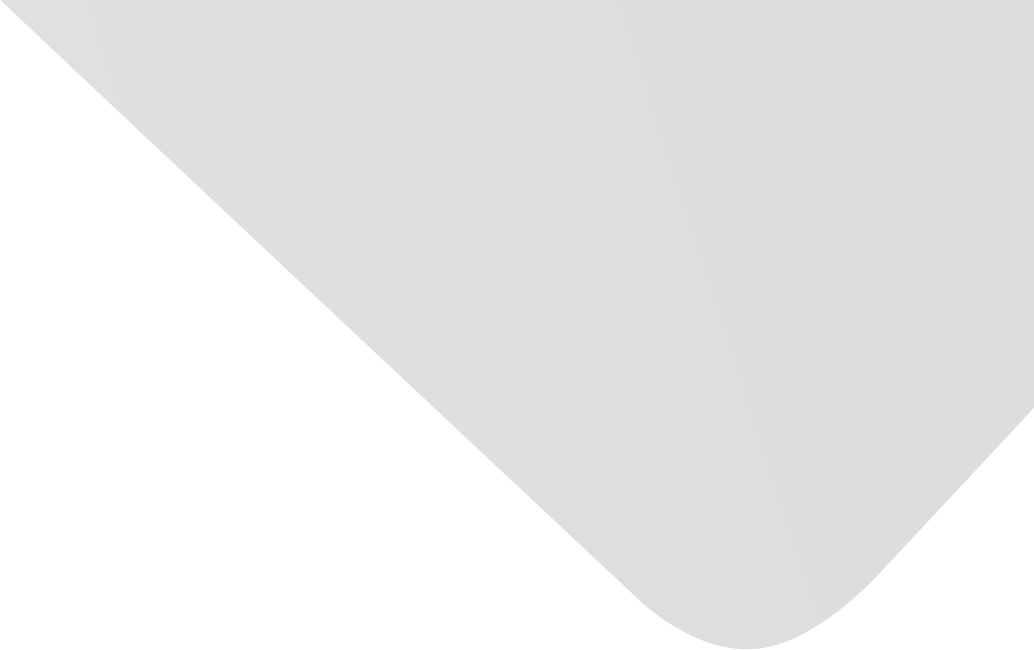
Optimal deep learning network for musculoskeletal X-Ray imaging classification for hospital medical record system
العناوين الأخرى
شبكة التعليم العميق المثلى لتصنيف التصوير بالأشعة السينية للعضلات الهيكلية لنظام السجلات الطبية بالمستشفى
المؤلفون المشاركون
al-Rashidi, Nayif Ilayyan
al-Rashidi, Muhammad Bin Umayr
al-Adibi, Muhammad Bin Ali
المصدر
Journal of Engineering Sciences and Information Technology
العدد
المجلد 6، العدد 7 (31 ديسمبر/كانون الأول 2022)، ص ص. 21-34، 14ص.
الناشر
تاريخ النشر
2022-12-31
دولة النشر
فلسطين (قطاع غزة)
عدد الصفحات
14
التخصصات الرئيسية
الملخص AR
أرشفة الصور الطبية هي أحد المكونات الأساسية لأي نظام للسجلات الطبية بالمستشفى (HMRS).
وهي تشمل، على سبيل المثال، التصوير بالرنين المغناطيسي، والأشعة المقطعية، والأشعة السينية، والموجات فوق الصوتية، والأشعة السينية للعضلات الهيكلية وما إلى ذلك.
تعد صور الأشعة السينية للعضلات الهيكلية ذات أهمية نسبية من حيث العدد بين الأنواع الأخرى من التصوير الطي.
تستخدم معظم نظام السجلات الطبية الموجودة بالمستشفى إما بالتعليق التوضيحي اليدوي للصور أو تستخدم البيانات الوصفية لكل صورة للأرشفة.
وجد هذا النهج على انه ناقصا بسبب العمل اليدوي المكثف، وفرص سوء التصنيف، والاعتماد التام على الخبرة البشرية.
علاوة على ذلك، من الصعب نسبيا معالجة أرشفة الصور وملفات التعريف الخاصة بها.
المنهجية: يمكن معالجة هذه المشكلة من خلال الحل المختلط لرؤية الكمبيوتر والتعلم العميق.
في الأدبيات الحديثة، اقترح الباحثون استخدام خوارزميات التعلم الآلي وخوارزميات التعلم العميق لتصنيف الصور الطبية الحيوية وأرشفتها.
ومع ذلك، وجد أن الأدبيات ناقصة للتوصية بشبكة تعلم عميق موحدة لتصنيف صور السينية للعضلات الهيكلية بدقة وكفاءة أكبر.
تعتبر مجموعة بيانات LERA واحدة من مجموعة بيانات صور الأشعة السينية للعضلات الهيكلية المعيارية.
النتائج على حد علمي، لا يزال البحث عن أفضل مرشح للشبكة العصبية العميقة مفقودا في الأدبيات، وستقدم هذه الدراسة الأساس المنطقي والتجريبي للتوصية بشبكة التعلم العميق المثلى لتصنيف التصوير بالأشعة السينية لنظام السجلات الطبية بالمستشفى باستخدام مجموعة بيانات 1ERA(الصور الإشعاعية للعضلات الهيكلية).
تم الاستنتاج أن المتغيرات من Resent وNet Google و Dark Net$ المرشح المقترح لتصنيف صور الأشعة السينية LEAR.
الملخص EN
Background: medical image archiving is one of the integral components of any hospital medical record system (HMRS).
It includes, but is not limited to, MRI, CT-Scan, X-ray, Ultrasound, Musculoskeletal X-rays etc.
the musculoskeletal X-ray images are relatively significant in number among the other types of medical imaging.
most of the existing HMRS use either the manual annotation of the images or use metadata for every image for archiving.
this approach is found to be deficient because of intensive manual work, chances of misclassification, and reliance on human expertise.
moreover, archiving the images and their metafiles is relatively difficult to handle.
methodology: this issue can be handled by a hybrid solution of computer vision and deep learning.
in the recent literature, researchers have proposed using machine learning and deep learning algorithms for biomedical image classification and archiving.
however, the literature is found to be insufficient to recommend a unified deep learning network for Musculoskeletal X-ray Image classification with greater accuracy and efficiency.
the LERA dataset is considered one of the benchmark Musculoskeletal X-rays image datasets.
results: to the best of knowledge the investigation of the best candidate of deep neural network is still missing in the literature.
this study will present the logical and empirical rationale for the recommendation of the optimal deep learning network for X-ray imaging classification for hospital medical record system using LERA (musculoskeletal radiographs) dataset.
it has been concluded that the variants of resnet, Google Net, and DarkNet are the suggested candidates for LERA x-ray image classification.
نمط استشهاد جمعية علماء النفس الأمريكية (APA)
al-Rashidi, Nayif Ilayyan& al-Rashidi, Muhammad Bin Umayr& al-Adibi, Muhammad Bin Ali. 2022. Optimal deep learning network for musculoskeletal X-Ray imaging classification for hospital medical record system. Journal of Engineering Sciences and Information Technology،Vol. 6, no. 7, pp.21-34.
https://search.emarefa.net/detail/BIM-1582537
نمط استشهاد الجمعية الأمريكية للغات الحديثة (MLA)
al-Rashidi, Nayif Ilayyan…[et al.]. Optimal deep learning network for musculoskeletal X-Ray imaging classification for hospital medical record system. Journal of Engineering Sciences and Information Technology Vol. 6, no. 7 (Dec. 2022), pp.21-34.
https://search.emarefa.net/detail/BIM-1582537
نمط استشهاد الجمعية الطبية الأمريكية (AMA)
al-Rashidi, Nayif Ilayyan& al-Rashidi, Muhammad Bin Umayr& al-Adibi, Muhammad Bin Ali. Optimal deep learning network for musculoskeletal X-Ray imaging classification for hospital medical record system. Journal of Engineering Sciences and Information Technology. 2022. Vol. 6, no. 7, pp.21-34.
https://search.emarefa.net/detail/BIM-1582537
نوع البيانات
مقالات
لغة النص
الإنجليزية
الملاحظات
Includes bibliographical references : p. 32-34
رقم السجل
BIM-1582537
قاعدة معامل التأثير والاستشهادات المرجعية العربي "ارسيف Arcif"
أضخم قاعدة بيانات عربية للاستشهادات المرجعية للمجلات العلمية المحكمة الصادرة في العالم العربي
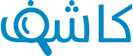
تقوم هذه الخدمة بالتحقق من التشابه أو الانتحال في الأبحاث والمقالات العلمية والأطروحات الجامعية والكتب والأبحاث باللغة العربية، وتحديد درجة التشابه أو أصالة الأعمال البحثية وحماية ملكيتها الفكرية. تعرف اكثر
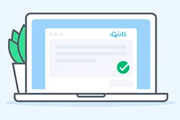