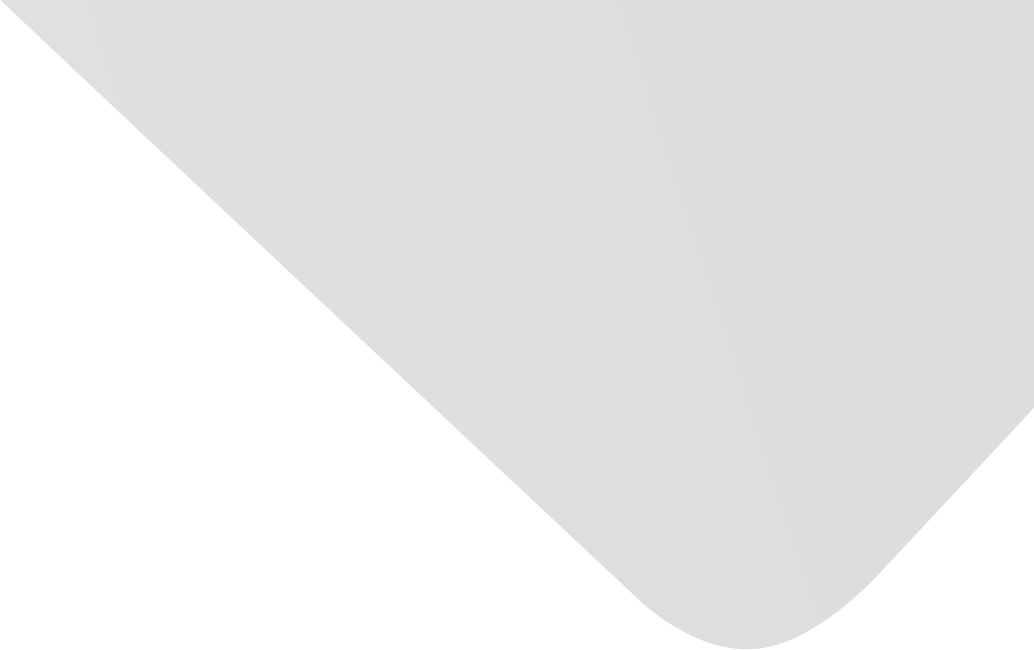
Intrusion detection using feed-forward neural networks
العناوين الأخرى
كشف الاختراق باستخدام الشبكات العصبونية ذات التغذية الأمامية
مقدم أطروحة جامعية
مشرف أطروحة جامعية
أعضاء اللجنة
al-Smadi, Adnan M.
Nhood, Jihad
al-Dawaid, Isam
الجامعة
جامعة آل البيت
الكلية
كلية الأمير الحسين بن عبد الله لتكنولوجيا المعلومات
القسم الأكاديمي
قسم علوم الحاسوب
دولة الجامعة
الأردن
الدرجة العلمية
ماجستير
تاريخ الدرجة العلمية
2008
الملخص الإنجليزي
Intrusion detection is an interesting approach that could be used to improve the security of network systems.
An Intrusion detection system (IDS) detects suspected patterns of network traffic on the remaining open parts through monitoring user activities (runtime gathering of data from system operations), and the subsequent analysis of these activities.
The main problem is the difficulty of distinguishing between natural behavior and abnormal behavior in computer networks due to the significant overlap in monitoring data.
This detection process generate (False Alarms) resulting from the use of intrusion detection based on the (Anomaly Intrusion Detection Systems).
The use of Fuzzy Set might reduce the amount of false alarm, where the degree of relationship to the use of any process for separation of this overlap could be used to define normal and abnormal behavior in computer networks.
For that data fuzzy fiction is needed before classification The purpose of this work is to contribute ideas of finding a solution to detect attacks (Intrusion) through building Intelligent detection system using feed-forward neural networks to detect attacks with low false negative rate (which is the most important point)and low false positive rate.
To do so, two feed-forward neural networks) architectures (one for non fuzzified data, the other for fuzzified data) are suggested, and their behaviors in detecting the attacks are studied.
To evaluate the performance of the proposed IDS, a standard set of data KDD (knowledge Discovery in Database) proposed by Massachusetts Institute of Technology’s (MIT) Lincoln Labs is used.
The dataset can be divided mainly into five categories Normal data, Probing attack, Dos attack, U2R attack, (R2L attack).
The suggested neural networks were trained with reduced feature set (12 out of 41 features), different neural network architectures were tested and the most proper one was used.
In this research, the suggested IDS not only has the ability to distinguish if the access is normal or attack, but also capable of distinguishing the attack type.
The suggested classifiers were tested over the entire dataset to evaluate real word performance.
The preliminary results are promising at which the accuracy percentage is about (95.9303 %) for Neural Network (NN) trained with non fuzzified dataset, while, the proportion of precision in the classification of the data after fuzzification is about (97.4890 %).
التخصصات الرئيسية
تكنولوجيا المعلومات وعلم الحاسوب
الموضوعات
عدد الصفحات
72
قائمة المحتويات
Table of contents.
Abstract.
Chapter one : Preface.
Chapter two : Theoretical background.
Chapter three : Design and implementation.
Chapter four : Assessment results.
Chapter five : Conclusion and future works.
References.
نمط استشهاد جمعية علماء النفس الأمريكية (APA)
al-Ubaydi, Khattab Mejeal Ali. (2008). Intrusion detection using feed-forward neural networks. (Master's theses Theses and Dissertations Master). Al albayt University, Jordan
https://search.emarefa.net/detail/BIM-302909
نمط استشهاد الجمعية الأمريكية للغات الحديثة (MLA)
al-Ubaydi, Khattab Mejeal Ali. Intrusion detection using feed-forward neural networks. (Master's theses Theses and Dissertations Master). Al albayt University. (2008).
https://search.emarefa.net/detail/BIM-302909
نمط استشهاد الجمعية الطبية الأمريكية (AMA)
al-Ubaydi, Khattab Mejeal Ali. (2008). Intrusion detection using feed-forward neural networks. (Master's theses Theses and Dissertations Master). Al albayt University, Jordan
https://search.emarefa.net/detail/BIM-302909
لغة النص
الإنجليزية
نوع البيانات
رسائل جامعية
رقم السجل
BIM-302909
قاعدة معامل التأثير والاستشهادات المرجعية العربي "ارسيف Arcif"
أضخم قاعدة بيانات عربية للاستشهادات المرجعية للمجلات العلمية المحكمة الصادرة في العالم العربي
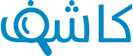
تقوم هذه الخدمة بالتحقق من التشابه أو الانتحال في الأبحاث والمقالات العلمية والأطروحات الجامعية والكتب والأبحاث باللغة العربية، وتحديد درجة التشابه أو أصالة الأعمال البحثية وحماية ملكيتها الفكرية. تعرف اكثر
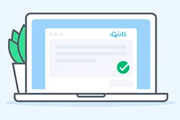