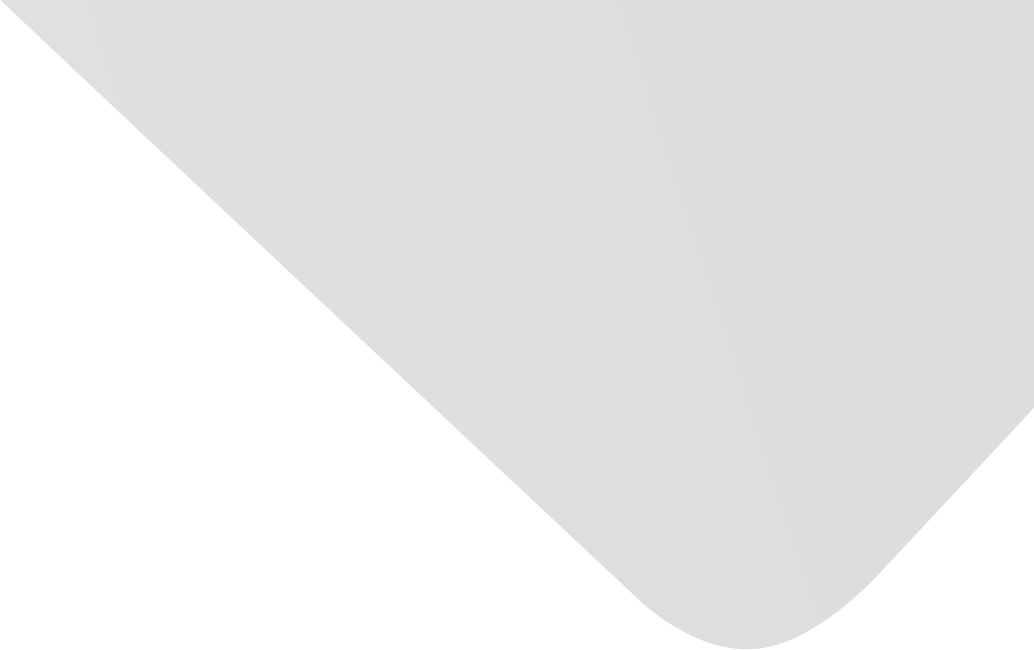
A hybrid strategy for minimizing association rules
مقدم أطروحة جامعية
مشرف أطروحة جامعية
al-Rababiah, Mamun S.
al-Hammami, Ala Husayn
أعضاء اللجنة
Shatnawi, Umar Ali
al-Batayinah, Khalid
Hanandeh, Isam Said S.
الجامعة
جامعة آل البيت
الكلية
كلية الأمير الحسين بن عبد الله لتكنولوجيا المعلومات
القسم الأكاديمي
قسم علوم الحاسوب
دولة الجامعة
الأردن
الدرجة العلمية
ماجستير
تاريخ الدرجة العلمية
2010
الملخص الإنجليزي
Associative classification is an integrating association rules mining and classification.
Associative Classification discovered patterns for useful information are important and in great demand in science, engineering and business.
Today, effective associative classification methods have been developed and widely used in various challenging industrial and business applications.
These methods attempt to provide significant and useful information for decision makers.
Paradoxically, associative classification itself can produce such huge amounts of rules that poses a knowledge management problem: to tackle thousands or even more rules discovered in a dataset.
In order to solve the rules number problem, in this study associative classification process involves two stages.
First: the data is preprocess to improve the classification ability and the efficiency of the classification process.
Second: by using concept of sample itemset matching, the hybrid system of data post-analysis is proposed.
The hybrid system includes data pruning, data clustering and data summarization to support effective analysis and interpretation of the discovered rules.
In data pruning the frequent and redundant itemsets are prune and data clustering using to grouping those itemsets results from data pruning into similar clusters.
Finally, data summarization using to prune itemsets in each clusters those results from data clustering.
In the Hybrid Strategy approach, we use the composite criteria of minimum support and minimum confidence as the rule weight to indicate the significance of the rule.
Through our study, we find it is important to find a good combination of these two rule interestingness threshold values. By using datasets from UCI Repository, we compared the Hybrid Strategy results with some well-known associative classification algorithms such as CMAR, CPAR, TFPC and Classification by Bagged Consistent Itemset Rules.
The results that achieved by Hybrid Strategy not only give better classification accuracy but also have better results of rules number.
Hybrid Strategy generates a much smaller set of high-quality predictive rules with high classification accuracy compare with these algorithms.
التخصصات الرئيسية
تكنولوجيا المعلومات وعلم الحاسوب
الموضوعات
عدد الصفحات
72
قائمة المحتويات
Table of contents.
Abstract.
Chapter One : introduction.
Chapter Two : associative classification.s
Chapter Three : the proposed strategy.
Chapter Four : implementation and experiment results.
Chapter Five : conclusions and future work.
References.
نمط استشهاد جمعية علماء النفس الأمريكية (APA)
Ali, Sayf Muhammad. (2010). A hybrid strategy for minimizing association rules. (Master's theses Theses and Dissertations Master). Al albayt University, Jordan
https://search.emarefa.net/detail/BIM-307564
نمط استشهاد الجمعية الأمريكية للغات الحديثة (MLA)
Ali, Sayf Muhammad. A hybrid strategy for minimizing association rules. (Master's theses Theses and Dissertations Master). Al albayt University. (2010).
https://search.emarefa.net/detail/BIM-307564
نمط استشهاد الجمعية الطبية الأمريكية (AMA)
Ali, Sayf Muhammad. (2010). A hybrid strategy for minimizing association rules. (Master's theses Theses and Dissertations Master). Al albayt University, Jordan
https://search.emarefa.net/detail/BIM-307564
لغة النص
الإنجليزية
نوع البيانات
رسائل جامعية
رقم السجل
BIM-307564
قاعدة معامل التأثير والاستشهادات المرجعية العربي "ارسيف Arcif"
أضخم قاعدة بيانات عربية للاستشهادات المرجعية للمجلات العلمية المحكمة الصادرة في العالم العربي
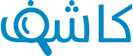
تقوم هذه الخدمة بالتحقق من التشابه أو الانتحال في الأبحاث والمقالات العلمية والأطروحات الجامعية والكتب والأبحاث باللغة العربية، وتحديد درجة التشابه أو أصالة الأعمال البحثية وحماية ملكيتها الفكرية. تعرف اكثر
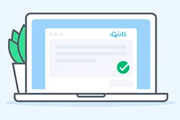