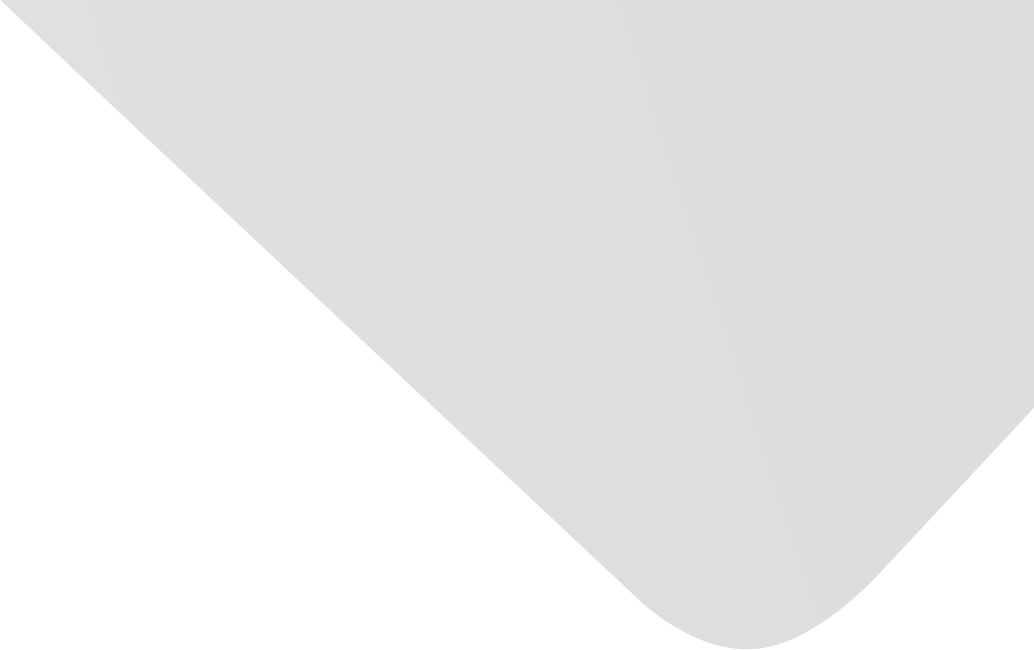
Neural networks of engine fault diagnosis based on exhaust gas analysis
المؤلفون المشاركون
Abd al-Wahhab, Qusayy T.
Laftah, Rafil Mahmud
Hamzah, Jafar M.
المصدر
Thi-Qar University Journal for Engineering Sciences
العدد
المجلد 4، العدد 1 (31 مارس/آذار 2013)، ص ص. 58-73، 16ص.
الناشر
تاريخ النشر
2013-03-31
دولة النشر
العراق
عدد الصفحات
16
التخصصات الرئيسية
الموضوعات
الملخص AR
يستخدم هذا العمل الشبكات العصبية الاصطناعية لتشخيص أعطال مولد كهربائي ذو محرك بأسطوانة واحدة، رباعي الأشواط، لقد تم اختبار أربعة عشر نوع من الأعطال بالإضافة إلى الحالة الطبيعية عمليا لغرض إنتاج مجموعة من البيانات الشاملة و الواقعية، استعملت هذه البيانات لتدريب و تحقيق الشبكة.
كانت البيانات الناتجة على شكل غازات العادم و سرعة المحرك المسجلة لكل حالة على حدة في ظل ظروف تحميل مختلفة.
بعد انتهاء عملية التعلم، تكون الشبكة قادرة على التشخيص عند استخدام بيانات جديدة.
البيانات المدخلة إلى الشبكة العصبية الصناعية تتضمن مجموعة من أعطال المحرك التي نفذت بشكل تجريبي لهذا الغرض.
و من هذه الأعطال، خلل في نظام المكربنة، نظام ترشيح الهواء، شمعة القدح، الصمامات، و حلقات المكبس ...
الخ.
أظهرت النتائج أن خوارزمية تدريب الرجوع العكسي هي كافية في تشخيص أعطال المحرك تحت ظروف التحميل المختلفة.
وجد أن قيم معامل الارتباط هي 0.999 و 1 لبيانات الاختبار و التدريب، على التوالي.
و أظهرت النتائج في هذا التحقيق أن نظام تشخيص الأخطاء المستند على الشبكات العصبية قادر على اكتشاف الخطأ و التشخيص مع موثوقية عالية.
الملخص EN
This work uses the Artificial Neural Networks (ANNs) for fault diagnosis of a single cylinder four stroke gasoline generator type (Astra Korea AST11700).
One normal and fourteen faulty conditions are examined experimentally to produce a realistic data set, which is to be used for the training and validation of the ANNs.
The resulted data was in the form of exhaust gases and engine speed records for each case separately under different loading conditions.
After the learning process is completed, the ANN becomes able to make a diagnosis about the gasoline engine condition when new data is presented.
The data presented to the ANN system include a subset of engine faults which were selected and executed experimentally for this topic.
These include, faults in carburetor, air filter, spark plug, valves, piston rings, etc.
The results showed that the multi-layer training algorithm is sufficient enough in diagnose engine faults under different loading conditions.
It was found that the correlation coefficient values are 0.999 and 1 for the testing and training data, respectively.
The results obtained in this investigation showed that the ANN-based fault diagnosis system is capable of fault diagnosis with high reliability.
نمط استشهاد جمعية علماء النفس الأمريكية (APA)
Laftah, Rafil Mahmud& Abd al-Wahhab, Qusayy T.& Hamzah, Jafar M.. 2013. Neural networks of engine fault diagnosis based on exhaust gas analysis. Thi-Qar University Journal for Engineering Sciences،Vol. 4, no. 1, pp.58-73.
https://search.emarefa.net/detail/BIM-343171
نمط استشهاد الجمعية الأمريكية للغات الحديثة (MLA)
Hamzah, Jafar M.…[et al.]. Neural networks of engine fault diagnosis based on exhaust gas analysis. Thi-Qar University Journal for Engineering Sciences Vol. 4, no. 1 (2013), pp.58-73.
https://search.emarefa.net/detail/BIM-343171
نمط استشهاد الجمعية الطبية الأمريكية (AMA)
Laftah, Rafil Mahmud& Abd al-Wahhab, Qusayy T.& Hamzah, Jafar M.. Neural networks of engine fault diagnosis based on exhaust gas analysis. Thi-Qar University Journal for Engineering Sciences. 2013. Vol. 4, no. 1, pp.58-73.
https://search.emarefa.net/detail/BIM-343171
نوع البيانات
مقالات
لغة النص
الإنجليزية
الملاحظات
Includes appendicx : p. 73
رقم السجل
BIM-343171
قاعدة معامل التأثير والاستشهادات المرجعية العربي "ارسيف Arcif"
أضخم قاعدة بيانات عربية للاستشهادات المرجعية للمجلات العلمية المحكمة الصادرة في العالم العربي

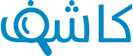
تقوم هذه الخدمة بالتحقق من التشابه أو الانتحال في الأبحاث والمقالات العلمية والأطروحات الجامعية والكتب والأبحاث باللغة العربية، وتحديد درجة التشابه أو أصالة الأعمال البحثية وحماية ملكيتها الفكرية. تعرف اكثر
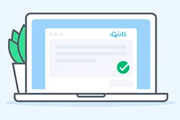