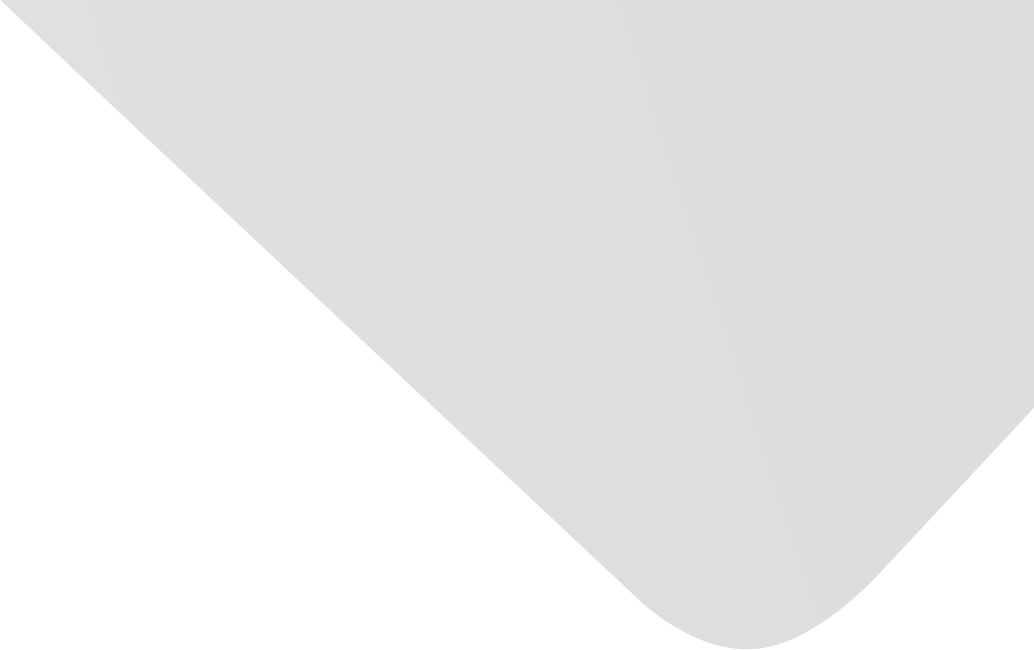
Data classification using quantum neural network
العناوين الأخرى
تصنيف البيانات باستخدام الشبكة العصبية الكمية
المؤلفون المشاركون
Abd al-Majid, Ghassan Hamid
al-Isa, Zaynab Tawfiq Baqir
Naji, Hasan Sad Allah
المصدر
العدد
المجلد 20، العدد 4 (30 إبريل/نيسان 2014)، ص ص. 36-50، 15ص.
الناشر
تاريخ النشر
2014-04-30
دولة النشر
العراق
عدد الصفحات
15
التخصصات الرئيسية
تكنولوجيا المعلومات وعلم الحاسوب
الموضوعات
الملخص AR
يتطرق البحث إلى الشبكة العصبية الكمية كإحدى أصناف الشبكات العصبية الاصطناعية و التي تتم من خلال دمج مجالين مهمين و هما مجال الاحتساب الكمي مع مجال الشبكة العصبية الاصطناعية.
تستخدم هذه الدائرة في مجال تقنية تصنيف البيانات, إن بيانات أزهار السوسن (Iris flower database) قد تم اعتمادها هنا كإشارات تصنيف.
و لهذا الغرض تم اعتماد تقنية تحليل المركبة الأساس (ICA) كتقنية استخلاص الخصائص من إشارات التصنيف بعد مرحلة المعالجة الأولية للبيانات لغرض تهيئة البيانات, إن الطبقة الوسطية المعروفة بالطبقة الخفية تتألف من عدد من الخلايا العصبية التي تكون متعددة المستويات لغرض معالجة بيانات التدريب و الاختبار لتعطي بذالك مستويات متعددة من التأكيد.
النتائج التجريبية تشير بأن الشبكة العصبية الكمية لها قدرة تمييز البيانات أفضل مقارنة بالشبكة العصبية الصناعية ولنفس الغرض, بالإضافة لذلك النتائج تشير بأن الشبكة العصبية الكمية تعطي نتائج تمييز (تصنيف) أسرع و أكثر واقعية أي إن دوائر QNN تعتبر الأفضل (بدقة تصل 97.778%) مقارنة بالشبكة العصبية الاصطناعية (التي تصل دقتها %93.334).
الملخص EN
In this paper, integrated quantum neural network (QNN), which is a class of feedforward neural networks (FFNN’s), is performed through emerging quantum computing (QC) with artificial neural network(ANN) classifier.
It is used in data classification technique, and here iris flower data is used as a classification signals.
For this purpose independent component analysis (ICA) is used as a feature extraction technique after normalization of these signals, the architecture of (QNN’s) has inherently built in fuzzy, hidden units of these networks (QNN’s) to develop quantized representations of sample information provided by the training data set in various graded levels of certainty.
Experimental results presented here show that (QNN’s) are capable of recognizing structures in data, a property that conventional (FFNN’s) with sigmoidal hidden units lack.
In addition, (QNN) gave a kind of fast and realistic results compared with the (FFNN).
Simulation results indicate that QNN is superior (with total accuracy of 97.778%) than ANN (with total accuracy of 93.334 %).
نمط استشهاد جمعية علماء النفس الأمريكية (APA)
Abd al-Majid, Ghassan Hamid& al-Isa, Zaynab Tawfiq Baqir& Naji, Hasan Sad Allah. 2014. Data classification using quantum neural network. Journal of Engineering،Vol. 20, no. 4, pp.36-50.
https://search.emarefa.net/detail/BIM-376996
نمط استشهاد الجمعية الأمريكية للغات الحديثة (MLA)
al-Isa, Zaynab Tawfiq Baqir…[et al.]. Data classification using quantum neural network. Journal of Engineering Vol. 20, no. 4 (Apr. 2014), pp.36-50.
https://search.emarefa.net/detail/BIM-376996
نمط استشهاد الجمعية الطبية الأمريكية (AMA)
Abd al-Majid, Ghassan Hamid& al-Isa, Zaynab Tawfiq Baqir& Naji, Hasan Sad Allah. Data classification using quantum neural network. Journal of Engineering. 2014. Vol. 20, no. 4, pp.36-50.
https://search.emarefa.net/detail/BIM-376996
نوع البيانات
مقالات
لغة النص
الإنجليزية
الملاحظات
Includes appendices : p. 45-50
رقم السجل
BIM-376996
قاعدة معامل التأثير والاستشهادات المرجعية العربي "ارسيف Arcif"
أضخم قاعدة بيانات عربية للاستشهادات المرجعية للمجلات العلمية المحكمة الصادرة في العالم العربي

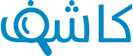
تقوم هذه الخدمة بالتحقق من التشابه أو الانتحال في الأبحاث والمقالات العلمية والأطروحات الجامعية والكتب والأبحاث باللغة العربية، وتحديد درجة التشابه أو أصالة الأعمال البحثية وحماية ملكيتها الفكرية. تعرف اكثر
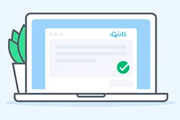