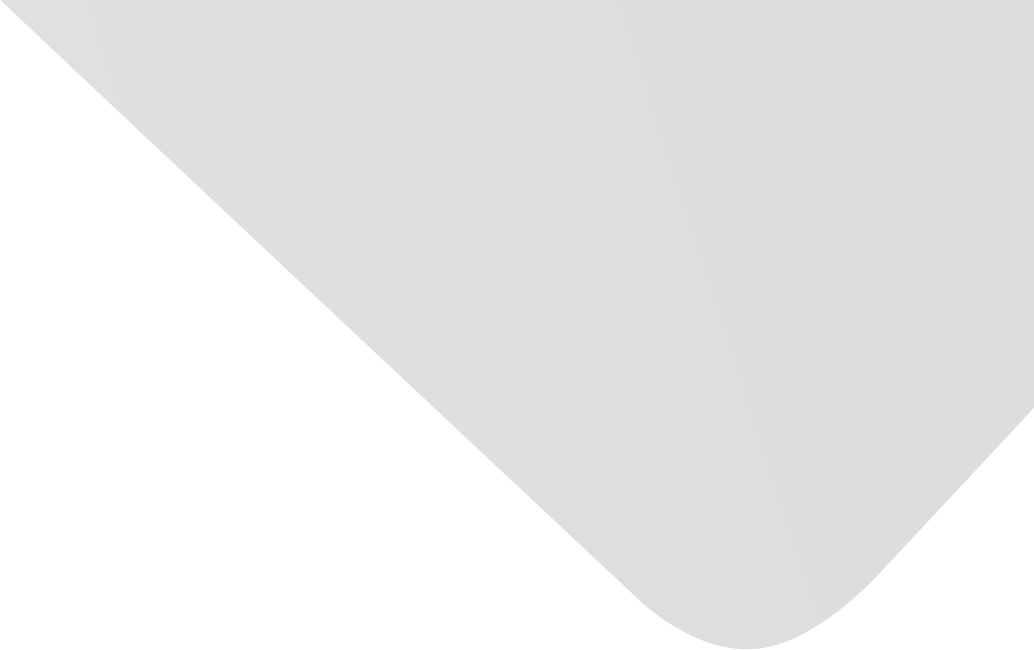
New genetic algorithm-neural networks-based methodologies to derive optimal fuzzy systems
المؤلفون المشاركون
Bin Muhammad, M.
Boudihir, M. al-arbi
Rahmun, A.
المصدر
The Arabian Journal for Science and Engineering. Section B, Engineering
العدد
المجلد 25، العدد 2B (31 أكتوبر/تشرين الأول 2000)، ص ص. 105-122، 18ص.
الناشر
جامعة الملك فهد للبترول و المعادن
تاريخ النشر
2000-10-31
دولة النشر
السعودية
عدد الصفحات
18
التخصصات الرئيسية
العلوم الهندسية والتكنولوجية (متداخلة التخصصات)
الموضوعات
الملخص AR
تعتبر نماذج الاستدلال الغامض أدوات تقدير لأنظمة الواقية، مما ينيل من أهميتها في تطبيقا ت التحكم- و يتوقف أداء هذه الأنظمة على عدة عوامل : مثل نظام قواعد الاستلزام، و صفات المجموعات الجزئية الغامضة، و عامل الاستلزام الغامض، و طريقة الانتقال من الغامض إلى النظام العلوم.
و في معظم الحالات, في مثل هذه العوامل فإنها تقرر من طرف الإنسان المختص وفقا لتجربته.
نحاول في هذا البحث تحسبن فعالية الأنظمة الغامضة باستعمال آليات التعلم التي تتركز على النظام الجيني.
و نقترح في هذا الموضوع منهجين يعتمد أن على طريقة التجويد ذات مستويين.
في كلا المنهجين فإن عقدة التكرار الجينية تستعمل لتجويد اختيار البارا مترات، و عملية التصنيف (قواعد الاستلزام) التي تعتمد على شبكة الخلايا العصبية الاصطناعية.
أما المنهج الأول فهو يستعمل نظام التعلم الموجه لضبط دقيق للتركيب، بينما المنهج الثاني يستعمل عقدة تكرار داخلية لإنشاء أفضل نموذج للاستدلال الغامض.
الملخص EN
Fuzzy reasoning models are model-free estimators of controi systems; this makes them very powerful tools in control applications.
Their performance depends on several factors such as the completeness of the rule base, the subjective definitions of the fuzzy subsets parameters or fuzzy partitioning, the fuzzy implication operator, and the defuzzification method.
In most cases, these factors are decided upon subjective experience of the human expert.
In this paper, we attempt to improve the fuzzy systems performance by means of genetic-based learning mechanisms.
We propose two methodologies.
These are two-step optimization methods.
In both methodologies, a Genetic Algorithm (GA) loop is used for parameter optimization and selection, and competitive clustering to generate clusters.
One methodology uses a supervised training algorithm for fine tuning the fuzzy structure (GA-ST), while the other uses an inner GA-loop to derive optimal Fuzzy Reasoning Model (FRM) parameters (GA2).
نمط استشهاد جمعية علماء النفس الأمريكية (APA)
Rahmun, A.& Bin Muhammad, M.& Boudihir, M. al-arbi. 2000. New genetic algorithm-neural networks-based methodologies to derive optimal fuzzy systems. The Arabian Journal for Science and Engineering. Section B, Engineering،Vol. 25, no. 2B, pp.105-122.
https://search.emarefa.net/detail/BIM-389591
نمط استشهاد الجمعية الأمريكية للغات الحديثة (MLA)
Bin Muhammad, M.…[et al.]. New genetic algorithm-neural networks-based methodologies to derive optimal fuzzy systems. The Arabian Journal for Science and Engineering. Section B, Engineering Vol. 25, no. 2B (Oct. 2000), pp.105-122.
https://search.emarefa.net/detail/BIM-389591
نمط استشهاد الجمعية الطبية الأمريكية (AMA)
Rahmun, A.& Bin Muhammad, M.& Boudihir, M. al-arbi. New genetic algorithm-neural networks-based methodologies to derive optimal fuzzy systems. The Arabian Journal for Science and Engineering. Section B, Engineering. 2000. Vol. 25, no. 2B, pp.105-122.
https://search.emarefa.net/detail/BIM-389591
نوع البيانات
مقالات
لغة النص
الإنجليزية
الملاحظات
Includes bibliographical references : p. 121-122
رقم السجل
BIM-389591
قاعدة معامل التأثير والاستشهادات المرجعية العربي "ارسيف Arcif"
أضخم قاعدة بيانات عربية للاستشهادات المرجعية للمجلات العلمية المحكمة الصادرة في العالم العربي
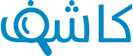
تقوم هذه الخدمة بالتحقق من التشابه أو الانتحال في الأبحاث والمقالات العلمية والأطروحات الجامعية والكتب والأبحاث باللغة العربية، وتحديد درجة التشابه أو أصالة الأعمال البحثية وحماية ملكيتها الفكرية. تعرف اكثر
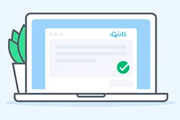