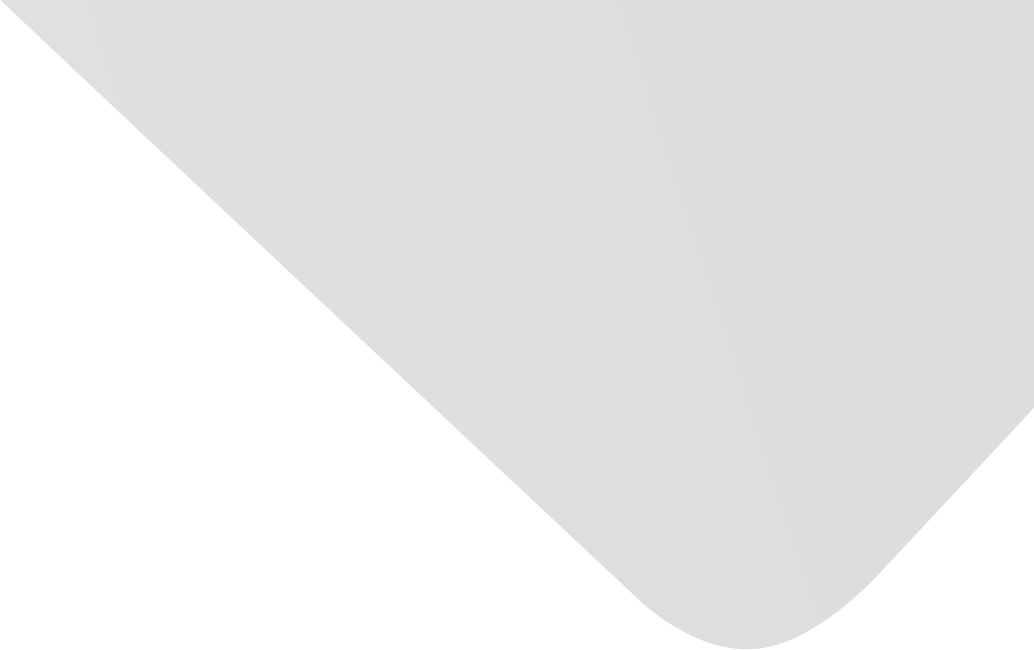
Adaptive Control Using Fully Online Sequential-Extreme Learning Machine and a Case Study on Engine Air-Fuel Ratio Regulation
المؤلفون المشاركون
Wong, Ka In
Wong, Pak-kin
Vong, Chi Man
Gao, Xiang Hui
المصدر
Mathematical Problems in Engineering
العدد
المجلد 2014، العدد 2014 (31 ديسمبر/كانون الأول 2014)، ص ص. 1-11، 11ص.
الناشر
Hindawi Publishing Corporation
تاريخ النشر
2014-04-07
دولة النشر
مصر
عدد الصفحات
11
التخصصات الرئيسية
الملخص EN
Most adaptive neural control schemes are based on stochastic gradient-descent backpropagation (SGBP), which suffers from local minima problem.
Although the recently proposed regularized online sequential-extreme learning machine (ReOS-ELM) can overcome this issue, it requires a batch of representative initial training data to construct a base model before online learning.
The initial data is usually difficult to collect in adaptive control applications.
Therefore, this paper proposes an improved version of ReOS-ELM, entitled fully online sequential-extreme learning machine (FOS-ELM).
While retaining the advantages of ReOS-ELM, FOS-ELM discards the initial training phase, and hence becomes suitable for adaptive control applications.
To demonstrate its effectiveness, FOS-ELM was applied to the adaptive control of engine air-fuel ratio based on a simulated engine model.
Besides, controller parameters were also analyzed, in which it is found that large hidden node number with small regularization parameter leads to the best performance.
A comparison among FOS-ELM and SGBP was also conducted.
The result indicates that FOS-ELM achieves better tracking and convergence performance than SGBP, since FOS-ELM tends to learn the unknown engine model globally whereas SGBP tends to “forget” what it has learnt.
This implies that FOS-ELM is more preferable for adaptive control applications.
نمط استشهاد جمعية علماء النفس الأمريكية (APA)
Wong, Pak-kin& Vong, Chi Man& Gao, Xiang Hui& Wong, Ka In. 2014. Adaptive Control Using Fully Online Sequential-Extreme Learning Machine and a Case Study on Engine Air-Fuel Ratio Regulation. Mathematical Problems in Engineering،Vol. 2014, no. 2014, pp.1-11.
https://search.emarefa.net/detail/BIM-457048
نمط استشهاد الجمعية الأمريكية للغات الحديثة (MLA)
Wong, Pak-kin…[et al.]. Adaptive Control Using Fully Online Sequential-Extreme Learning Machine and a Case Study on Engine Air-Fuel Ratio Regulation. Mathematical Problems in Engineering No. 2014 (2014), pp.1-11.
https://search.emarefa.net/detail/BIM-457048
نمط استشهاد الجمعية الطبية الأمريكية (AMA)
Wong, Pak-kin& Vong, Chi Man& Gao, Xiang Hui& Wong, Ka In. Adaptive Control Using Fully Online Sequential-Extreme Learning Machine and a Case Study on Engine Air-Fuel Ratio Regulation. Mathematical Problems in Engineering. 2014. Vol. 2014, no. 2014, pp.1-11.
https://search.emarefa.net/detail/BIM-457048
نوع البيانات
مقالات
لغة النص
الإنجليزية
الملاحظات
Includes bibliographical references
رقم السجل
BIM-457048
قاعدة معامل التأثير والاستشهادات المرجعية العربي "ارسيف Arcif"
أضخم قاعدة بيانات عربية للاستشهادات المرجعية للمجلات العلمية المحكمة الصادرة في العالم العربي
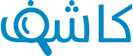
تقوم هذه الخدمة بالتحقق من التشابه أو الانتحال في الأبحاث والمقالات العلمية والأطروحات الجامعية والكتب والأبحاث باللغة العربية، وتحديد درجة التشابه أو أصالة الأعمال البحثية وحماية ملكيتها الفكرية. تعرف اكثر
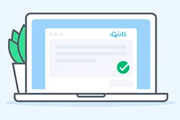