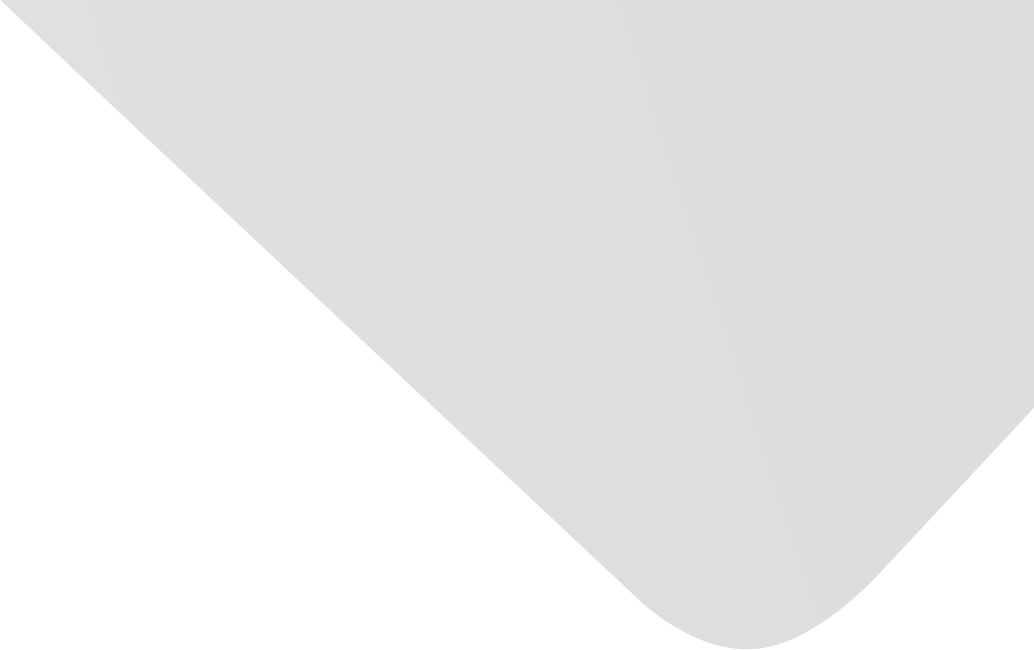
Neuro-snake pattern recognition and classification using gradiant vector flow (Gvf and Hnn)
العناوين الأخرى
طريقة الثعبان العصبي للتميز والتصنيف باستخدام مجري المنحدر الاتجاهي
المؤلف
المصدر
Engineering and Technology Journal
العدد
المجلد 27، العدد 05 (30 إبريل/نيسان 2009)، ص ص. 973-982، 10ص.
الناشر
تاريخ النشر
2009-04-30
دولة النشر
العراق
عدد الصفحات
10
التخصصات الرئيسية
الموضوعات
الملخص AR
أهم تطبيقات الشبكات العصبية و كمثال شبكة ال (Hopfield) هي تمييز الأشكال و تصنيفها (pattern recognition and classification).
و لكن هناك بعض التحديدات المهمة في عمل هذا النوع من المصنف و هي مشكلة التذبذب (oscillation) أي عدم القدرة على تمييز الأشكال المتقاربة جدا.
في هذا البحث تم ابتكار طريقة تجمع بين الناقلات الفعالة (active contour) أو ما تسمى بال (snake) و الشبكات العصبية الاصطناعية (ANN) لتعمل كمميز للأشكال و تصنيفها.
البحث يستخدم تقنية ناقلات التدفق التدريجي (GVF) في عمل المصنف حيث تقوم بتحديد دقيق للإطار الخاص بالشكل (الصورة) ثم تمريره إلى مصنف مبني على أساس الشبكة العصبية الاصطناعية (HNN) ليتم تصنيفه نسبة إلى احد الأشكال المخزونة سلفا في المصنف.
الثعبان (snake) لها القدرة العالية للالتفاف على حدود الشكل المطلوب بدقة عالية لتأخذ شكل المقطع المطلوب مع حذف الإضافات الغير مطلوبة من الشكل (noise) و هذا سيساعد على تقليل نسبة الخطأ النقطي (bit error rate) إلى دون 0.215 نسبة إلى أكثر من 0.5 في المصنف الاعتيادي و تجنب حالة التذبذب الموجودة في المصنف الخاص بالشبكات العصبية الاصطناعية تم استخدام برنامج 7 mat lab لعمل المحاكاة للناقلات الفعالة (GVF) و عملية التصنيف النهائية للأشكال.
الملخص EN
The most popular applications of Hopfield neural network algorithm (HNN) are pattern recognition and classification.
But the HNN has some limitation like the local minima (oscillation) problem.
In this paper a novel method of combining an active contour (snake) and an artificial neural network to behave together as pattern recognition and classification is presented.
The approach used the technique of the gradient vector flow (GVF) that locate the boundary of target pattern (image) then pass it to a classifier built by Hopfield algorithm to classify it according to one of the storage pattern.
The snakes can find the boundaries of objects so it is very accurate to take the shape of the object wanted, that will eliminate the noise from the original image and reduce the bit error rate of the Hopfield network to 0.215 and overcome the oscillation state in recognition of the entered pattern.
MATLAB 7 program have been used for the simulation of the active contour and the pattern classification.
نمط استشهاد جمعية علماء النفس الأمريكية (APA)
Ali, Wissam Hasan. 2009. Neuro-snake pattern recognition and classification using gradiant vector flow (Gvf and Hnn). Engineering and Technology Journal،Vol. 27, no. 05, pp.973-982.
https://search.emarefa.net/detail/BIM-49622
نمط استشهاد الجمعية الأمريكية للغات الحديثة (MLA)
Ali, Wissam Hasan. Neuro-snake pattern recognition and classification using gradiant vector flow (Gvf and Hnn). Engineering and Technology Journal Vol. 27, no. 05 (2009), pp.973-982.
https://search.emarefa.net/detail/BIM-49622
نمط استشهاد الجمعية الطبية الأمريكية (AMA)
Ali, Wissam Hasan. Neuro-snake pattern recognition and classification using gradiant vector flow (Gvf and Hnn). Engineering and Technology Journal. 2009. Vol. 27, no. 05, pp.973-982.
https://search.emarefa.net/detail/BIM-49622
نوع البيانات
مقالات
لغة النص
الإنجليزية
الملاحظات
Includes bibliographical references : p. 976-977
رقم السجل
BIM-49622
قاعدة معامل التأثير والاستشهادات المرجعية العربي "ارسيف Arcif"
أضخم قاعدة بيانات عربية للاستشهادات المرجعية للمجلات العلمية المحكمة الصادرة في العالم العربي
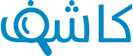
تقوم هذه الخدمة بالتحقق من التشابه أو الانتحال في الأبحاث والمقالات العلمية والأطروحات الجامعية والكتب والأبحاث باللغة العربية، وتحديد درجة التشابه أو أصالة الأعمال البحثية وحماية ملكيتها الفكرية. تعرف اكثر
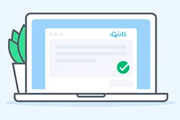