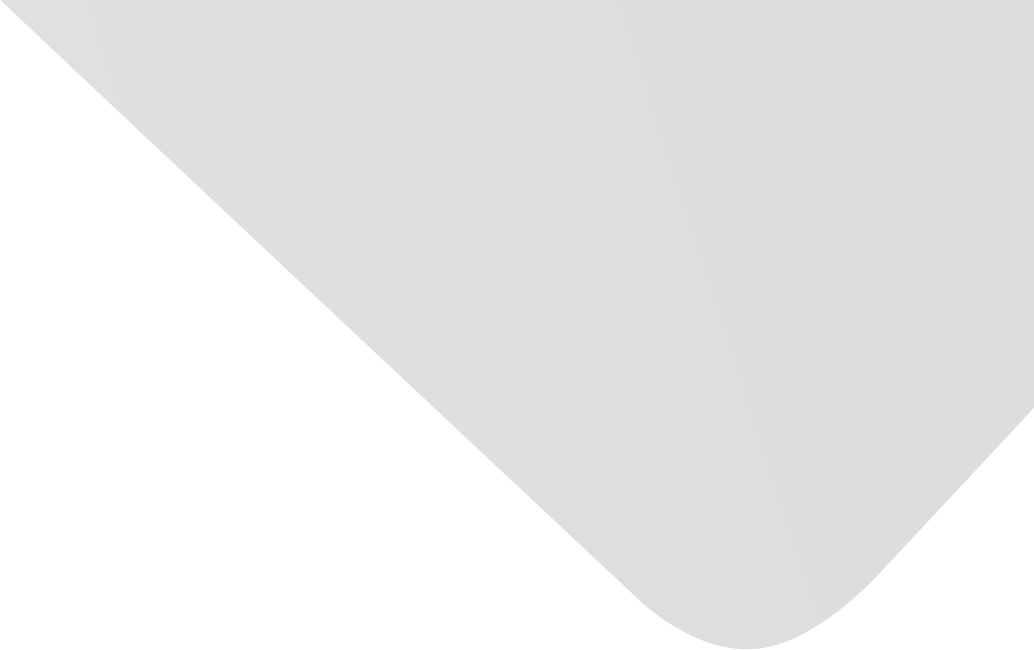
Handwritten recognition using neural networks based on multiple feature extraction algorithms
العناوين الأخرى
تمييز كتابة اليد باستخدام الشبكات العصبية المعتمدة على طرق متعددة لاستخلاص الخصائص
المؤلفون المشاركون
al-Jumah, Basim Abd al-Baqi
al-Shammari, Iyad Ghazi Nasir
Kazim, Maryam Khalaf
المصدر
العدد
المجلد 55، العدد 4A (31 ديسمبر/كانون الأول 2014)، ص ص. 1625-1637، 13ص.
الناشر
تاريخ النشر
2014-12-31
دولة النشر
العراق
عدد الصفحات
13
التخصصات الرئيسية
تكنولوجيا المعلومات وعلم الحاسوب
الموضوعات
الملخص AR
الحروف المكتوبة باليد تكون مبهمة في الغالب كما قد لا تكون الخطوط مستقيمة تماما"، و المنحنيات لا تكون بالضرورة بشكل واضح، و تختلف بذلك عن الحروف المطبوعة.
علاوة على ذلك، الحرف يمكن أن يرسم بحجوم مختلفة.
لذا، هذا البحث اقترحنا استخدام أربعة طرق معتمدة على خوارزميتي استخلاص الخصائص.
إن الخوارزمية الأولى هي تحويg الموجات المتقطعة Discrete wavelet transform و الخوارزمية الثانية هي تحويل رادون Radon transform و من خلال التجارب وجدت أن استخلاص الخصائص باستخدام تحويل رادون Radon transform هو تحويل أكثر دقة من تحويل المويجات المتقطعة Discrete wavelet transform.
التقنية المقترحة حساب تحويل رادون في اتجاهات مختلفة و يلتقط ملامح الاتجاه من الصور الحروف.
كان نسبة التمييز هي 93 % عند تدريب الشبكات العصبية باسخدام تحويل الرادون.
تمت المقارنة بين أربعة طرق لاختيار الأفضل منها.
تم تدريب الشبكات العصبية باستخدام خوارزمية الانتشار العكسي Back-Propagation.
الملخص EN
Handwritten letters are vague in nature as there may not always be sharp perfectly straight lines, and curves not necessarily be clearly, unlike the printed letters.
Furthermore, letter can be drawn in different sizes.
Therefore, the proposed used of four methods based on two algorithms for feature extraction.
The first algorithm discrete wavelet transform and second algorithm is Radon transform, by experiments was found that the feature extraction using radon transform is more precise than wavelet transform.
The proposed technique computes Radon projections in different orientations and captures the directional features of letter images.
The obtain recognition rate was 93 % when used for train artificial neural networks.
Comparative between four methods to choose the best has been done.
Training method is based on Back-Propagation learning algorithm used by feed forward Neural Network.
نمط استشهاد جمعية علماء النفس الأمريكية (APA)
al-Jumah, Basim Abd al-Baqi& al-Shammari, Iyad Ghazi Nasir& Kazim, Maryam Khalaf. 2014. Handwritten recognition using neural networks based on multiple feature extraction algorithms. Iraqi Journal of Science،Vol. 55, no. 4A, pp.1625-1637.
https://search.emarefa.net/detail/BIM-516571
نمط استشهاد الجمعية الأمريكية للغات الحديثة (MLA)
al-Jumah, Basim Abd al-Baqi…[et al.]. Handwritten recognition using neural networks based on multiple feature extraction algorithms. Iraqi Journal of Science Vol. 55, no. 4A (2014), pp.1625-1637.
https://search.emarefa.net/detail/BIM-516571
نمط استشهاد الجمعية الطبية الأمريكية (AMA)
al-Jumah, Basim Abd al-Baqi& al-Shammari, Iyad Ghazi Nasir& Kazim, Maryam Khalaf. Handwritten recognition using neural networks based on multiple feature extraction algorithms. Iraqi Journal of Science. 2014. Vol. 55, no. 4A, pp.1625-1637.
https://search.emarefa.net/detail/BIM-516571
نوع البيانات
مقالات
لغة النص
الإنجليزية
الملاحظات
Text in English ; abstracts in English and Arabic.
رقم السجل
BIM-516571
قاعدة معامل التأثير والاستشهادات المرجعية العربي "ارسيف Arcif"
أضخم قاعدة بيانات عربية للاستشهادات المرجعية للمجلات العلمية المحكمة الصادرة في العالم العربي
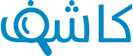
تقوم هذه الخدمة بالتحقق من التشابه أو الانتحال في الأبحاث والمقالات العلمية والأطروحات الجامعية والكتب والأبحاث باللغة العربية، وتحديد درجة التشابه أو أصالة الأعمال البحثية وحماية ملكيتها الفكرية. تعرف اكثر
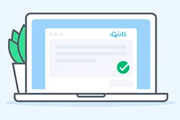