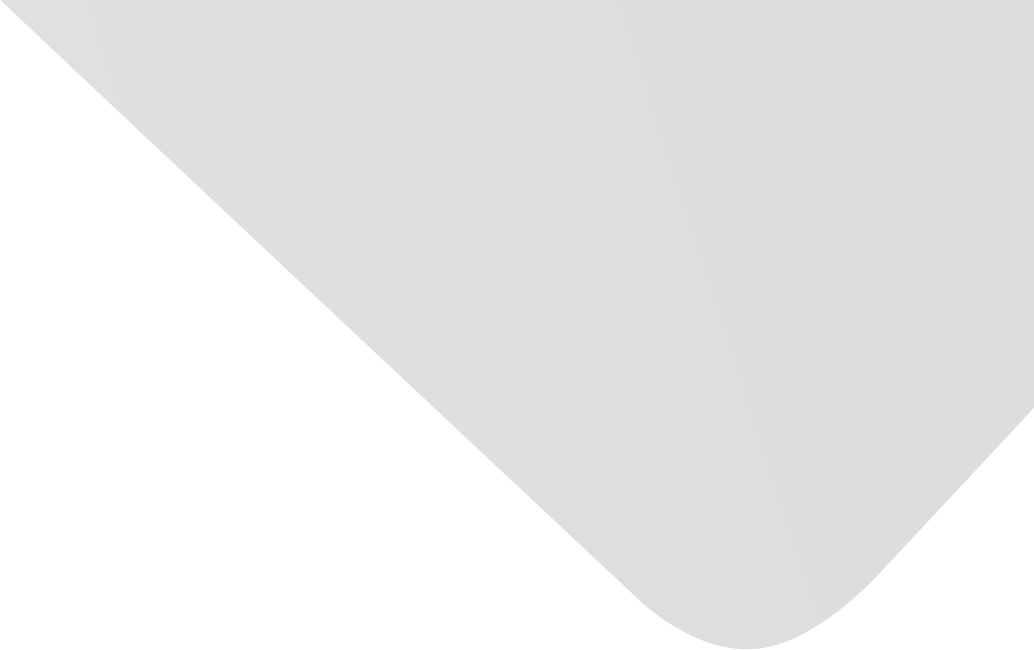
Investigating approaches to enhance document clustering by exploiting background knowledge in word net and Wikipedia
العناوين الأخرى
التحقيق في طرق لتحسين تصنيف الملفات عن طريق استغلال المعرفة الخلفية في وردنت و ويكيبيديا
مقدم أطروحة جامعية
مشرف أطروحة جامعية
الجامعة
الجامعة الإسلامية
الكلية
كلية تكنولوجيا المعلومات
القسم الأكاديمي
قسم نظم تكنولوجيا المعلومات
دولة الجامعة
فلسطين (قطاع غزة)
الدرجة العلمية
ماجستير
تاريخ الدرجة العلمية
2015
الملخص الإنجليزي
Clustering is one of the main data analysis techniques.
Document clustering generates clusters from the whole document collection automatically and it is used in numerous applications, including market research, pattern recognition, data analysis, and image processing.
Traditional techniques of document clustering do not consider the semantic relationships between words when assigning documents to clusters.
For instance, if two documents talking about the same topic but by using different words (which may be synonyms or semantically associated), these techniques may assign documents to different clusters.
Previous research has approached this problem by enriching the document representation with the background knowledge from an ontology or a controlled vocabulary such as Wordnet.
This research builds on previous efforts and provides a thorough investigation on the use of controlled vocabularies such as WordNet and knowledge resources such as Wikipedia to enhance document clustering.
The contribution of this research is twofold: First, it provides a thorough investigation on the value of using WordNet to enhance document clustering: previous researches which explored the use of WordNet for document clustering often showed conflicting results: some efforts claim that WordNet has the potential to improve the performance of the clustering by helping to identify synonyms and semantically related words in the document collection.
Other researches claim that WordNet provides little or no enhancement on the clustering results.
In this research, we will try to experimentally resolve this conflict between the two teams, and explain why WordNet could be useful in some cases while not in others, and what factors can influence the value v of the WordNet.
We have conducted several experiments in which we tested the use of WordNet for document clustering over different testing conditions such as different data sets, different similarity measures and different settings for the clustering algorithm.
Results have shown that different experimental settings will result in different results, and that the influence of WordNet on the clustering results varies based on the used settings.
The importance of these results is that they can inform the designers of experiments, who are willing to use WordNet for document clustering, of the best settings they should use in order to obtain the ultimate benefit from WordNet, For instance, using the Reuters dataset, the clustering with synonyms gave the best results (F-score =0.77 and purity =0.64 ), followed by the clustering with similarity scores (F-score=0.70, Purity=0.59), followed by the clustering without any semantics (F-score=0.64, Purity=0.57).
Second, this thesis presents a novel approach to enhance document clustering by exploiting the semantic knowledge contained in Wikipedia.
It uses the link structure of Wikipedia to measure the semantic relatedness between terms and use the similarity scores to enhance the document’s representation vector.
The proposed approach differs from related efforts which also used Wikipedia for document clustering in two aspects: first, it uses a similarity measure that is modelled after the Normalized Google Distance which is a well-known and low-cost method of measuring term similarity.
Second, it is more time efficient as it applies an algorithm for phrase extraction from documents prior to mapping terms to Wikipedia.
Our approach was evaluated by being compared with different methods from the state of the art using two vi different datasets.
Empirical results showed that our approach improved the clustering results as compared to other similar approaches, According to the F-score measure, for the Reuters dataset, our method (Wikipedia) and Hotho et al’s method (WordNet) achieve 31% and 9% respectively, for the OHSUMed dataset, our method and Hotho et al’s method achieve 27% and 4% respectively.
التخصصات الرئيسية
تكنولوجيا المعلومات وعلم الحاسوب
الموضوعات
عدد الصفحات
72
قائمة المحتويات
Table of contents.
Abstract.
Abstract in Arabic.
Chapter One : Introduction.
Chapter Two : Literature review.
Chapter Three : Related work.
Chapter Four : Investigating the influence of word net on document clustering.
Chapter Five : An efficient approach for semantically-enhanced document clustering by using Wikipedia link structure.
Chapter Six : Conclusions and future work.
References.
نمط استشهاد جمعية علماء النفس الأمريكية (APA)
Nafi, Rami. (2015). Investigating approaches to enhance document clustering by exploiting background knowledge in word net and Wikipedia. (Master's theses Theses and Dissertations Master). Islamic University, Palestine (Gaza Strip)
https://search.emarefa.net/detail/BIM-615616
نمط استشهاد الجمعية الأمريكية للغات الحديثة (MLA)
Nafi, Rami. Investigating approaches to enhance document clustering by exploiting background knowledge in word net and Wikipedia. (Master's theses Theses and Dissertations Master). Islamic University. (2015).
https://search.emarefa.net/detail/BIM-615616
نمط استشهاد الجمعية الطبية الأمريكية (AMA)
Nafi, Rami. (2015). Investigating approaches to enhance document clustering by exploiting background knowledge in word net and Wikipedia. (Master's theses Theses and Dissertations Master). Islamic University, Palestine (Gaza Strip)
https://search.emarefa.net/detail/BIM-615616
لغة النص
الإنجليزية
نوع البيانات
رسائل جامعية
رقم السجل
BIM-615616
قاعدة معامل التأثير والاستشهادات المرجعية العربي "ارسيف Arcif"
أضخم قاعدة بيانات عربية للاستشهادات المرجعية للمجلات العلمية المحكمة الصادرة في العالم العربي
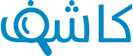
تقوم هذه الخدمة بالتحقق من التشابه أو الانتحال في الأبحاث والمقالات العلمية والأطروحات الجامعية والكتب والأبحاث باللغة العربية، وتحديد درجة التشابه أو أصالة الأعمال البحثية وحماية ملكيتها الفكرية. تعرف اكثر
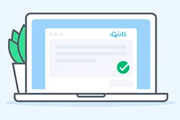