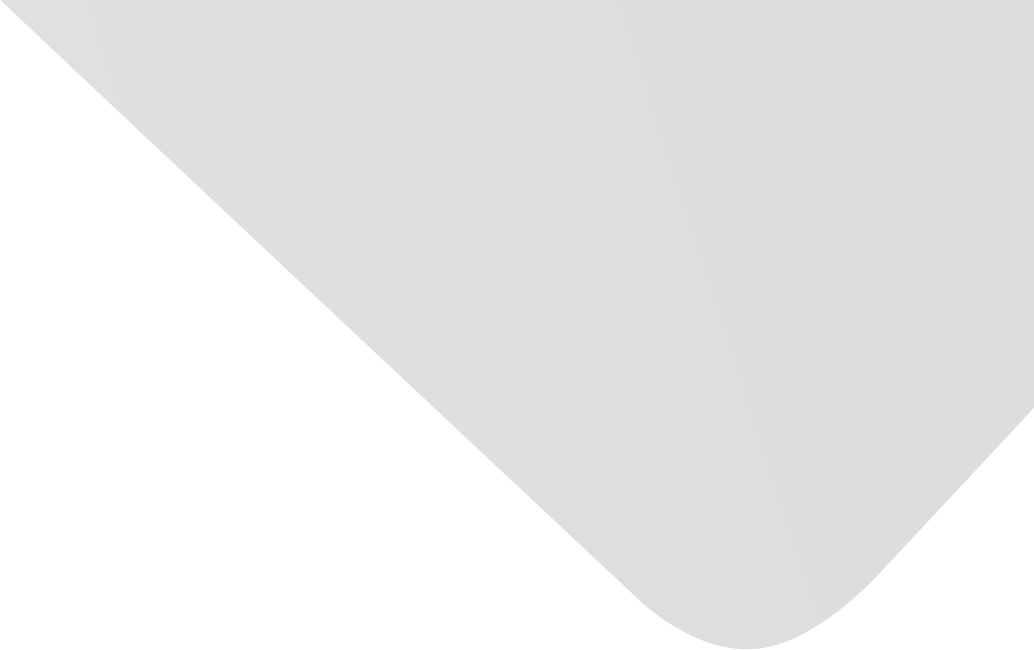
Biometrics continuous authentication for detecting compromised accounts on Facebook
العناوين الأخرى
المصادقة المستمرة باستخدام القياسات الحيوية لكشف الحسابات المخترقة على ال Facebook
مقدم أطروحة جامعية
مشرف أطروحة جامعية
al-Madi, Nailah
al-Qatawinah, Jafar
الجامعة
جامعة الأميرة سمية للتكنولوجيا
الكلية
كلية الملك الحسين لعلوم الحوسبة
القسم الأكاديمي
أمن نظم المعلومات و الجرائم الرقمية
دولة الجامعة
الأردن
الدرجة العلمية
ماجستير
تاريخ الدرجة العلمية
2018
الملخص الإنجليزي
Online Social Networks have become one of the key channels for social communication.
One of the main features that characterize most online social networks is that they are profile based services where a user needs to create an account to start using their services.
Such accounts or profiles are subject to several security and privacy threats.
Account breakthrough represents one of the most critical security threats to users as it violates account owner privacy and an imposter can exploit account information and pre-established connections to use it in a bad and abusive manner.
This thesis addresses the use of behavioral biometrics to detect intrusions on a Facebook account and authenticate users on a continuous manner.
In particular, keystroke dynamics features in addition to social interaction patterns are used to characterize user behavior over Facebook.
The efficiency of keystroke dynamics features are validated by collecting and analyzing real user keystrokes during their normal use of Facebook.
While social behavioral features are validated through collecting and analyzing same users Facebook activity logs.
Four well known machine learning classifiers including (Decision trees, Random Forest, Multi-Layer Perceptron neural networks, and Naïve Bayes) are applied and tested on collected data to evaluate the capability of built models in discriminating legitimate users from impostors.
Experimental performance results for both keystroke dynamics features as well as social behavioral features indicate that built classification models are able to discriminate a legitimate user from an impostor with 90.8% accuracy rate obtained using keystrokes based models and 99% accuracy rate obtained using social behavior based models.
It has been shown through conducting experiments that classification models perform better for detecting impostor than for continuous authentication approach, with precision values that range between 90% and 93% respectively, and recall values that range between 92% and 97% for the keystrokes based models.
The same inference with precision and recall results for the social behavior based models that ranges between 94% and 100% precision, and 93% and 100% recall.
التخصصات الرئيسية
تكنولوجيا المعلومات وعلم الحاسوب
الموضوعات
عدد الصفحات
70
قائمة المحتويات
Table of contents.
Abstract.
Abstract in Arabic.
Chapter One : Introduction.
Chapter Two : Background.
Chapter Three : Related work.
Chapter Four : Proposed methodology.
Chapter Five : Results and discussion.
Chapter Six : Conclusion and future work.
References.
نمط استشهاد جمعية علماء النفس الأمريكية (APA)
al-Qatawinah, Rawan Nashat. (2018). Biometrics continuous authentication for detecting compromised accounts on Facebook. (Master's theses Theses and Dissertations Master). Jordan
https://search.emarefa.net/detail/BIM-833375
نمط استشهاد الجمعية الأمريكية للغات الحديثة (MLA)
al-Qatawinah, Rawan Nashat. Biometrics continuous authentication for detecting compromised accounts on Facebook. (Master's theses Theses and Dissertations Master). (2018).
https://search.emarefa.net/detail/BIM-833375
نمط استشهاد الجمعية الطبية الأمريكية (AMA)
al-Qatawinah, Rawan Nashat. (2018). Biometrics continuous authentication for detecting compromised accounts on Facebook. (Master's theses Theses and Dissertations Master). Jordan
https://search.emarefa.net/detail/BIM-833375
لغة النص
الإنجليزية
نوع البيانات
رسائل جامعية
رقم السجل
BIM-833375
قاعدة معامل التأثير والاستشهادات المرجعية العربي "ارسيف Arcif"
أضخم قاعدة بيانات عربية للاستشهادات المرجعية للمجلات العلمية المحكمة الصادرة في العالم العربي

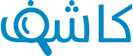
تقوم هذه الخدمة بالتحقق من التشابه أو الانتحال في الأبحاث والمقالات العلمية والأطروحات الجامعية والكتب والأبحاث باللغة العربية، وتحديد درجة التشابه أو أصالة الأعمال البحثية وحماية ملكيتها الفكرية. تعرف اكثر
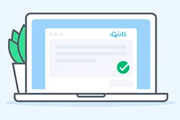