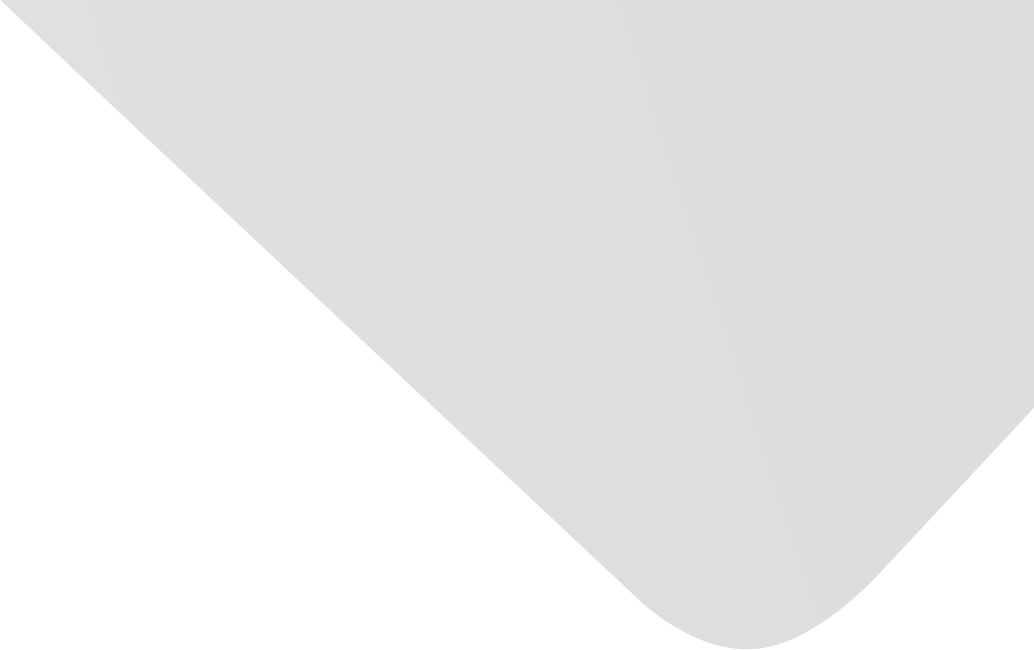
Diabetes risk level predictionusing data mining techniques
مقدم أطروحة جامعية
مشرف أطروحة جامعية
الجامعة
جامعة الإسراء
الكلية
كلية تكنولوجيا المعلومات
القسم الأكاديمي
قسم هندسة البرمجيات
دولة الجامعة
الأردن
الدرجة العلمية
ماجستير
تاريخ الدرجة العلمية
2019
الملخص الإنجليزي
Big data faces many challenges in various aspects that appear through characteristicssuch As: volume, velocity, and variety; big data processes and analyzis challenges acquiring quality information to support accurate decision-making values.
Health care produces large amount of data by follow up the patients.
This data can be used for diagnosing, detecting abnormal behavior and decision-making.
Nevertheless, in critical fields that are directly related to human health care, the data must be treated in manner to overcome unwanted medical actions related to Big Data.
Diabetics Big Data is rich in medical details, due to the frequency of updating case, and rich in gaps and unwanted data as well.
Therefore, precise work on big data makes the diagnoses prediction of diabetics in terms of risk level possible.
This prediction helps the doctor to overcome the ambiguousproblem of the case in future and predict the optimal treatment at early stage of the case.
In this work, an approach is proposed to pre-process the benchmark dataset UCI and select the correlated features based on target attribute.
Fuzzy .C-Means is used to values clustering and Support.
Vector Machine (S.VM) is used for.
classification as well.
Clustering and classification techniques are used to increase the clarity of data to enrich the rules that will be generated from dataset.
Risk Matrix was proposed to represent rules of three levels of diabetes (low, high,medium), and use Risk Matrix to train deep learning and build an expert system that can predict the risk level automatically.
The approach is tested in the fourth layer using the evaluation Metrics of machine learning algorithms.
The approach experiments use Diabetes patient data and symptom in rapidminer tool.
This approach Achieved 97.8% accuracy to automatically predict the level of risk and can be applied at the field of health care to target diabetic patients at variant levels of risks and provide customized care to reduce the re-admission rate.
التخصصات الرئيسية
تكنولوجيا المعلومات وعلم الحاسوب
الموضوعات
عدد الصفحات
59
قائمة المحتويات
Table of contents.
Abstract.
Chapter One : Introduction.
Chapter Two : Background and wrevious works.
Chapter Three : Proposed approach.
Chapter Four : Experience and discussions.
Chapter Five : Conclusion and future works.
References.
نمط استشهاد جمعية علماء النفس الأمريكية (APA)
Mahdi, Shajan Muhammad. (2019). Diabetes risk level predictionusing data mining techniques. (Master's theses Theses and Dissertations Master). Isra University, Jordan
https://search.emarefa.net/detail/BIM-896669
نمط استشهاد الجمعية الأمريكية للغات الحديثة (MLA)
Mahdi, Shajan Muhammad. Diabetes risk level predictionusing data mining techniques. (Master's theses Theses and Dissertations Master). Isra University. (2019).
https://search.emarefa.net/detail/BIM-896669
نمط استشهاد الجمعية الطبية الأمريكية (AMA)
Mahdi, Shajan Muhammad. (2019). Diabetes risk level predictionusing data mining techniques. (Master's theses Theses and Dissertations Master). Isra University, Jordan
https://search.emarefa.net/detail/BIM-896669
لغة النص
الإنجليزية
نوع البيانات
رسائل جامعية
رقم السجل
BIM-896669
قاعدة معامل التأثير والاستشهادات المرجعية العربي "ارسيف Arcif"
أضخم قاعدة بيانات عربية للاستشهادات المرجعية للمجلات العلمية المحكمة الصادرة في العالم العربي
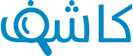
تقوم هذه الخدمة بالتحقق من التشابه أو الانتحال في الأبحاث والمقالات العلمية والأطروحات الجامعية والكتب والأبحاث باللغة العربية، وتحديد درجة التشابه أو أصالة الأعمال البحثية وحماية ملكيتها الفكرية. تعرف اكثر
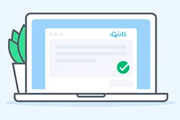