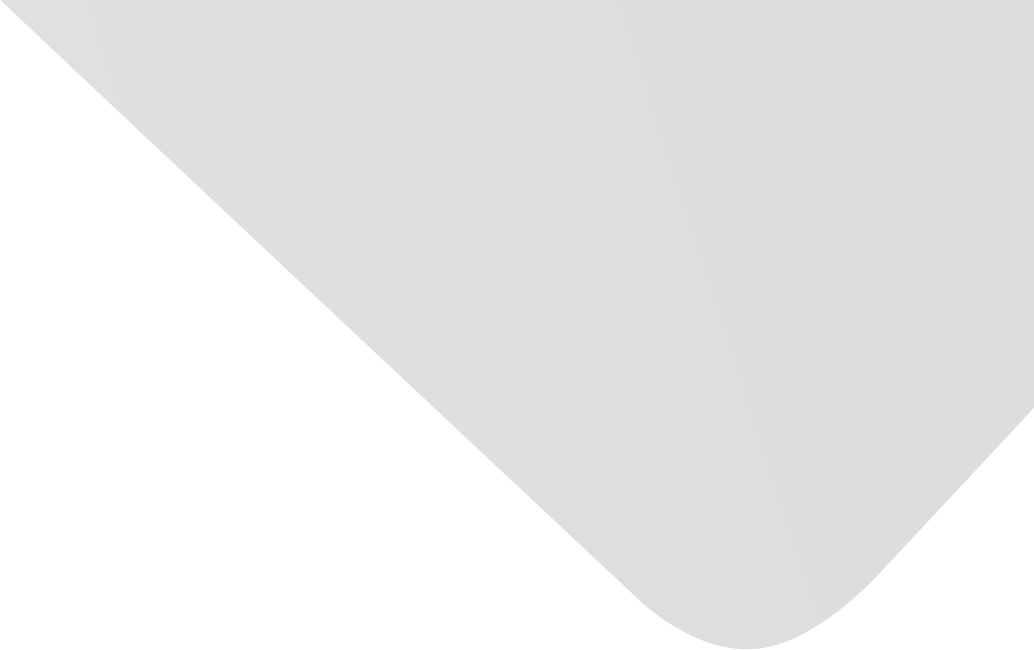
Optimizing linear models via sinusoidal transformation for boosted machine learning in medicine
العناوين الأخرى
تحسين النماذج الخطية عن طريق التحويل الجيبي لتعلم الآلة المعزز في الطب
المؤلف
المصدر
Journal of the Faculty of Medicine Baghdad
العدد
المجلد 61، العدد 3-4 (31 ديسمبر/كانون الأول 2019)، ص ص. 128-136، 9ص.
الناشر
تاريخ النشر
2019-12-31
دولة النشر
العراق
عدد الصفحات
9
التخصصات الرئيسية
الموضوعات
الملخص AR
يعتمد التعلم الآلي على مزيج من التحليلات، بما في ذلك تحليلات الانحدار، و التراجع الخطي.
لا توجد هناك هناك محاولات مسبقة لأستخدام المحولات الجيبية للبيانات لتعزيز نماذج الانحدار الخطي.
الأهداف : نحن نهدف إلى تحسين النماذج الخطية من خلال تطبيق التحويل الجيبي لتقليل العدد الإجمالي للمربعات للبيانات لتعزيز نماذج الانحدار الخطي.
المنهجية : قمنا بتطبيق إحصاءات غيربايزي باستخدام SPSS و MatLab تم استخدام Excel لإنشاء ثلاثين تجربة لنماذج الانحدار الخطي، و لكل منها ألف ملاحظة (عينة).
تم استخدام برنامج SPSS من أجل الانحدار الخطي، و اختبار و يلكوكسون، و إحصائيات كرونباخ ألفا لتقييم أداء نموذج التحسين (التحويل الجيبي).
النتائج : كان التحويل الجيبي ناجحًا عن طريق تقليل إجمالي المربعات و بقيمة P<0.001 بشكل أحصائي ملحوظ.
أكد اختبار كرونباخ ألفا الثبات الداخلي للنموذج المستخدم (معامل كرونباخ ألفا = 0.999) الإستتنتاج : يعد نموذج التحويل الجيبي ذو أهمية في الأبحاث عالية التأثير التي تعتمد على الانحدار و التراجع الخطي.
حيث يمكن أن تقلل من متطلبات المعالجة الحسابية لتحليلات قوية في الوقت الحقيقي و التنبؤات الأحصائية.
الملخص EN
Background : Machine learning relies on a hybrid of analytics, including regression analyses.
There have been no attempts to deploy a sinusoidal transformation of data to enhance linear regression models.
Objectives : We aim to optimize linear models by implementing sinusoidal transformation to minimize the sum of squared error.
Methods : We implemented non-Bayesian statistics using SPSS and MatLab.
We used Excel to generate 30 trials of linear regression models, and each has 1, 000 observations.
We utilized SPSS linear regression, Wilcoxon signed-rank test, and Cronbach's alpha statistics to evaluate the performance of the optimization model.
Results : Thesinusoidal transformation succeeded by significantly reducing the sum of squared errors (P-value < 0.
001).
Inter-item reliability testing confirmed the robust internal consistency of the model (Cronbach's alpha = 0.
999).
Conclusion : Ouroptimization model is valuable for high-impact research based on linear regression.
It can reduce the computational processing demands for powerful real-time and predictive analytics of big data.
نمط استشهاد جمعية علماء النفس الأمريكية (APA)
al-Imam, Ahmad Muhammad Lutfi. 2019. Optimizing linear models via sinusoidal transformation for boosted machine learning in medicine. Journal of the Faculty of Medicine Baghdad،Vol. 61, no. 3-4, pp.128-136.
https://search.emarefa.net/detail/BIM-973985
نمط استشهاد الجمعية الأمريكية للغات الحديثة (MLA)
al-Imam, Ahmad Muhammad Lutfi. Optimizing linear models via sinusoidal transformation for boosted machine learning in medicine. Journal of the Faculty of Medicine Baghdad Vol. 61, no. 3-4 (2019), pp.128-136.
https://search.emarefa.net/detail/BIM-973985
نمط استشهاد الجمعية الطبية الأمريكية (AMA)
al-Imam, Ahmad Muhammad Lutfi. Optimizing linear models via sinusoidal transformation for boosted machine learning in medicine. Journal of the Faculty of Medicine Baghdad. 2019. Vol. 61, no. 3-4, pp.128-136.
https://search.emarefa.net/detail/BIM-973985
نوع البيانات
مقالات
لغة النص
الإنجليزية
الملاحظات
Includes bibliographical references : p. 133-135
رقم السجل
BIM-973985
قاعدة معامل التأثير والاستشهادات المرجعية العربي "ارسيف Arcif"
أضخم قاعدة بيانات عربية للاستشهادات المرجعية للمجلات العلمية المحكمة الصادرة في العالم العربي
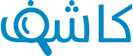
تقوم هذه الخدمة بالتحقق من التشابه أو الانتحال في الأبحاث والمقالات العلمية والأطروحات الجامعية والكتب والأبحاث باللغة العربية، وتحديد درجة التشابه أو أصالة الأعمال البحثية وحماية ملكيتها الفكرية. تعرف اكثر
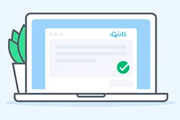