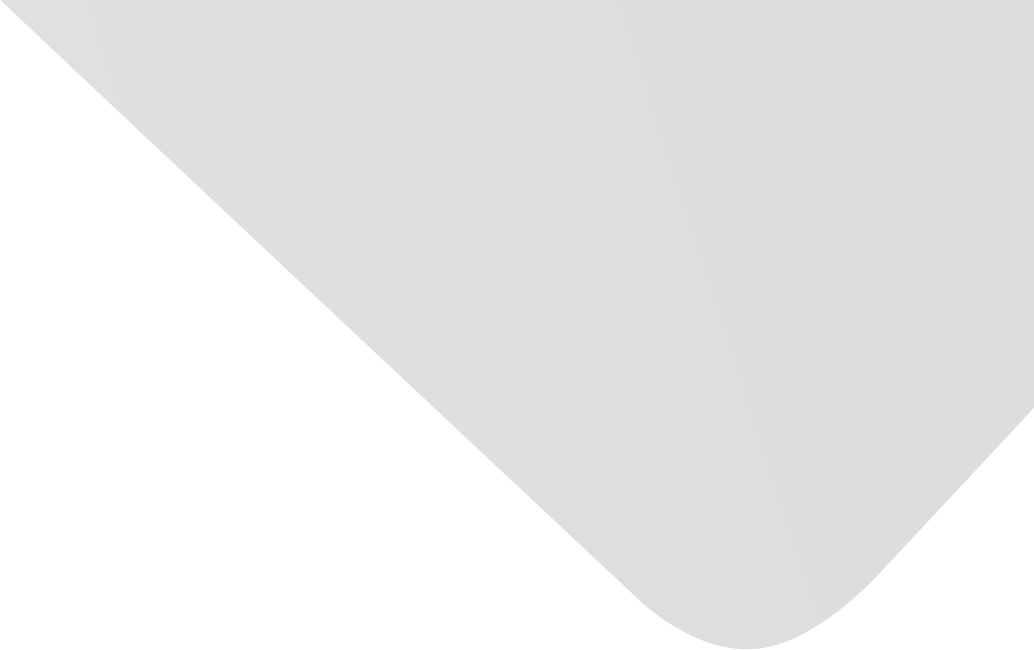
A New Hybrid Method Logistic Regression and Feedforward Neural Network for Lung Cancer Data
Author
Source
Mathematical Problems in Engineering
Issue
Vol. 2012, Issue 2012 (31 Dec. 2012), pp.1-10, 10 p.
Publisher
Hindawi Publishing Corporation
Publication Date
2012-12-05
Country of Publication
Egypt
No. of Pages
10
Main Subjects
Abstract EN
Logistic regression (LR) is a conventional statistical technique used for data classification problem.
Logistic regression is a model-based method, and it uses nonlinear model structure.
Another technique used for classification is feedforward artificial neural networks.
Feedforward artificial neural network is a data-based method which can model nonlinear models through its activation function.
In this study, a hybrid approach of model-based logistic regression technique and data-based artificial neural network was proposed for classification purposes.
The proposed approach was applied to lung cancer data, and obtained results were compared.
It was seen that the proposed hybrid approach was superior to logistic regression and feedforward artificial neural networks with respect to many criteria.
American Psychological Association (APA)
Tunç, Taner. 2012. A New Hybrid Method Logistic Regression and Feedforward Neural Network for Lung Cancer Data. Mathematical Problems in Engineering،Vol. 2012, no. 2012, pp.1-10.
https://search.emarefa.net/detail/BIM-1001428
Modern Language Association (MLA)
Tunç, Taner. A New Hybrid Method Logistic Regression and Feedforward Neural Network for Lung Cancer Data. Mathematical Problems in Engineering No. 2012 (2012), pp.1-10.
https://search.emarefa.net/detail/BIM-1001428
American Medical Association (AMA)
Tunç, Taner. A New Hybrid Method Logistic Regression and Feedforward Neural Network for Lung Cancer Data. Mathematical Problems in Engineering. 2012. Vol. 2012, no. 2012, pp.1-10.
https://search.emarefa.net/detail/BIM-1001428
Data Type
Journal Articles
Language
English
Notes
Includes bibliographical references
Record ID
BIM-1001428