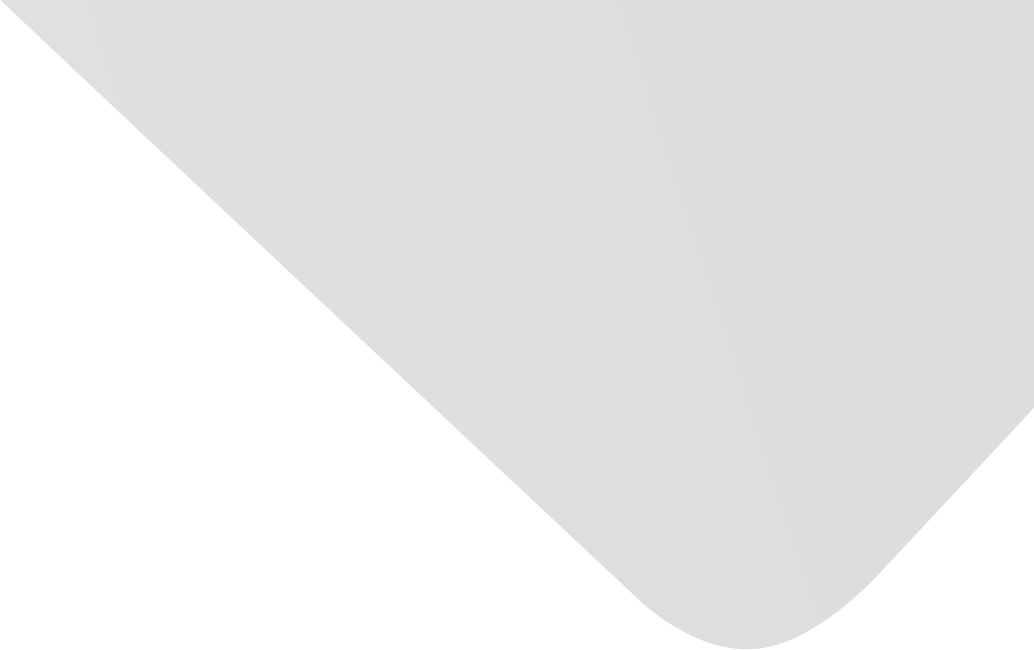
Semisupervised Kernel Marginal Fisher Analysis for Face Recognition
Joint Authors
Wang, Ziqiang
Sun, Xia
Sun, Lijun
Huang, Yuchun
Source
Issue
Vol. 2013, Issue 2013 (31 Dec. 2013), pp.1-13, 13 p.
Publisher
Hindawi Publishing Corporation
Publication Date
2013-09-12
Country of Publication
Egypt
No. of Pages
13
Main Subjects
Medicine
Information Technology and Computer Science
Abstract EN
Dimensionality reduction is a key problem in face recognition due to the high-dimensionality of face image.
To effectively cope with this problem, a novel dimensionality reduction algorithm called semisupervised kernel marginal Fisher analysis (SKMFA) for face recognition is proposed in this paper.
SKMFA can make use of both labelled and unlabeled samples to learn the projection matrix for nonlinear dimensionality reduction.
Meanwhile, it can successfully avoid the singularity problem by not calculating the matrix inverse.
In addition, in order to make the nonlinear structure captured by the data-dependent kernel consistent with the intrinsic manifold structure, a manifold adaptive nonparameter kernel is incorporated into the learning process of SKMFA.
Experimental results on three face image databases demonstrate the effectiveness of our proposed algorithm.
American Psychological Association (APA)
Wang, Ziqiang& Sun, Xia& Sun, Lijun& Huang, Yuchun. 2013. Semisupervised Kernel Marginal Fisher Analysis for Face Recognition. The Scientific World Journal،Vol. 2013, no. 2013, pp.1-13.
https://search.emarefa.net/detail/BIM-1033516
Modern Language Association (MLA)
Wang, Ziqiang…[et al.]. Semisupervised Kernel Marginal Fisher Analysis for Face Recognition. The Scientific World Journal No. 2013 (2013), pp.1-13.
https://search.emarefa.net/detail/BIM-1033516
American Medical Association (AMA)
Wang, Ziqiang& Sun, Xia& Sun, Lijun& Huang, Yuchun. Semisupervised Kernel Marginal Fisher Analysis for Face Recognition. The Scientific World Journal. 2013. Vol. 2013, no. 2013, pp.1-13.
https://search.emarefa.net/detail/BIM-1033516
Data Type
Journal Articles
Language
English
Notes
Includes bibliographical references
Record ID
BIM-1033516