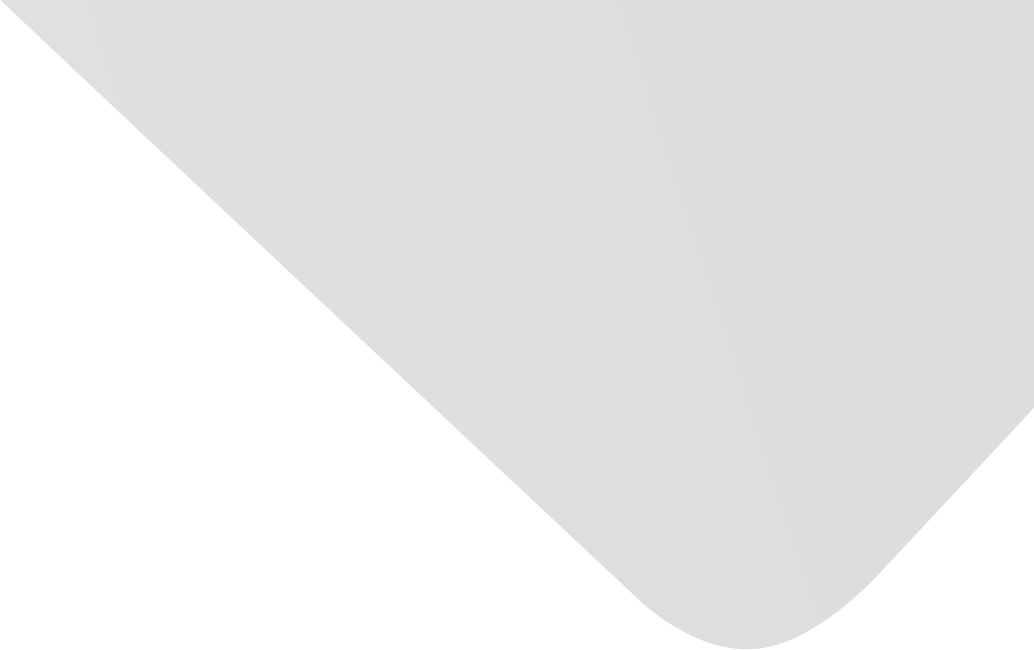
Study on Support Vector Machine-Based Fault Detection in Tennessee Eastman Process
Joint Authors
Yin, Shen
Zhu, Xiangping
Gao, Xin
Karimi, Hamid Reza
Source
Issue
Vol. 2014, Issue 2014 (31 Dec. 2014), pp.1-8, 8 p.
Publisher
Hindawi Publishing Corporation
Publication Date
2014-04-24
Country of Publication
Egypt
No. of Pages
8
Main Subjects
Abstract EN
This paper investigates the proficiency of supportvector machine (SVM) using datasets generated by TennesseeEastman process simulation for fault detection.
Due to its excellentperformance in generalization, the classification performanceof SVM is satisfactory.
SVM algorithm combined with kernelfunction has the nonlinear attribute and can better handle thecase where samples and attributes are massive.
In addition, withforehand optimizing the parameters using the cross-validationtechnique, SVM can produce high accuracy in fault detection.
Therefore, there is no need to deal with original data orrefer to other algorithms, making the classification problemsimple to handle.
In order to further illustrate the efficiency,an industrial benchmark of Tennessee Eastman (TE) process isutilized with the SVM algorithm and PLS algorithm, respectively.
By comparing the indices of detection performance, the SVMtechnique shows superior fault detection ability to the PLSalgorithm.
American Psychological Association (APA)
Yin, Shen& Gao, Xin& Karimi, Hamid Reza& Zhu, Xiangping. 2014. Study on Support Vector Machine-Based Fault Detection in Tennessee Eastman Process. Abstract and Applied Analysis،Vol. 2014, no. 2014, pp.1-8.
https://search.emarefa.net/detail/BIM-1034041
Modern Language Association (MLA)
Yin, Shen…[et al.]. Study on Support Vector Machine-Based Fault Detection in Tennessee Eastman Process. Abstract and Applied Analysis No. 2014 (2014), pp.1-8.
https://search.emarefa.net/detail/BIM-1034041
American Medical Association (AMA)
Yin, Shen& Gao, Xin& Karimi, Hamid Reza& Zhu, Xiangping. Study on Support Vector Machine-Based Fault Detection in Tennessee Eastman Process. Abstract and Applied Analysis. 2014. Vol. 2014, no. 2014, pp.1-8.
https://search.emarefa.net/detail/BIM-1034041
Data Type
Journal Articles
Language
English
Notes
Includes bibliographical references
Record ID
BIM-1034041