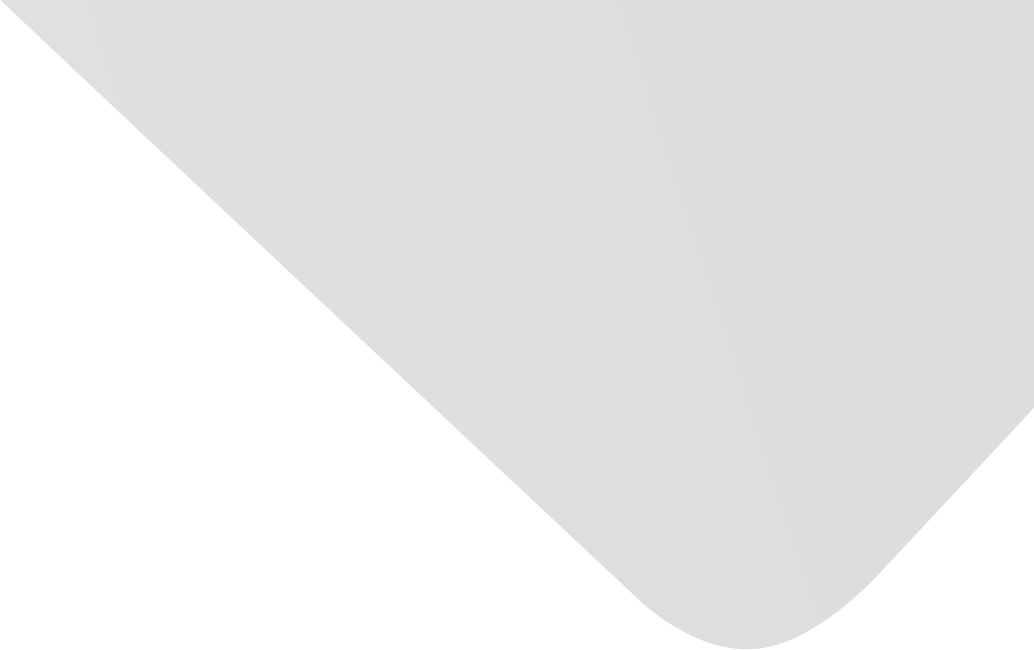
A Mahalanobis Hyperellipsoidal Learning Machine Class Incremental Learning Algorithm
Joint Authors
Qin, Yuping
Li, Dan
Lun, Shuxian
Zhang, Aihua
Karimi, Hamid Reza
Source
Issue
Vol. 2014, Issue 2014 (31 Dec. 2014), pp.1-5, 5 p.
Publisher
Hindawi Publishing Corporation
Publication Date
2014-02-11
Country of Publication
Egypt
No. of Pages
5
Main Subjects
Abstract EN
A Mahalanobis hyperellipsoidal learning machine class incremental learning algorithm is proposed.
To each class sample, the hyperellipsoidal that encloses as many as possible and pushes the outlier samples away is trained in the feature space.
In the process of incremental learning, only one subclassifier is trained with the new class samples.
The old models of the classifier are not influenced and can be reused.
In the process of classification, considering the information of sample’s distribution in the feature space, the Mahalanobis distances from the sample mapping to the center of each hyperellipsoidal are used to decide the classified sample class.
The experimental results show that the proposed method has higher classification precision and classification speed.
American Psychological Association (APA)
Qin, Yuping& Karimi, Hamid Reza& Li, Dan& Lun, Shuxian& Zhang, Aihua. 2014. A Mahalanobis Hyperellipsoidal Learning Machine Class Incremental Learning Algorithm. Abstract and Applied Analysis،Vol. 2014, no. 2014, pp.1-5.
https://search.emarefa.net/detail/BIM-1034074
Modern Language Association (MLA)
Qin, Yuping…[et al.]. A Mahalanobis Hyperellipsoidal Learning Machine Class Incremental Learning Algorithm. Abstract and Applied Analysis No. 2014 (2014), pp.1-5.
https://search.emarefa.net/detail/BIM-1034074
American Medical Association (AMA)
Qin, Yuping& Karimi, Hamid Reza& Li, Dan& Lun, Shuxian& Zhang, Aihua. A Mahalanobis Hyperellipsoidal Learning Machine Class Incremental Learning Algorithm. Abstract and Applied Analysis. 2014. Vol. 2014, no. 2014, pp.1-5.
https://search.emarefa.net/detail/BIM-1034074
Data Type
Journal Articles
Language
English
Notes
Includes bibliographical references
Record ID
BIM-1034074