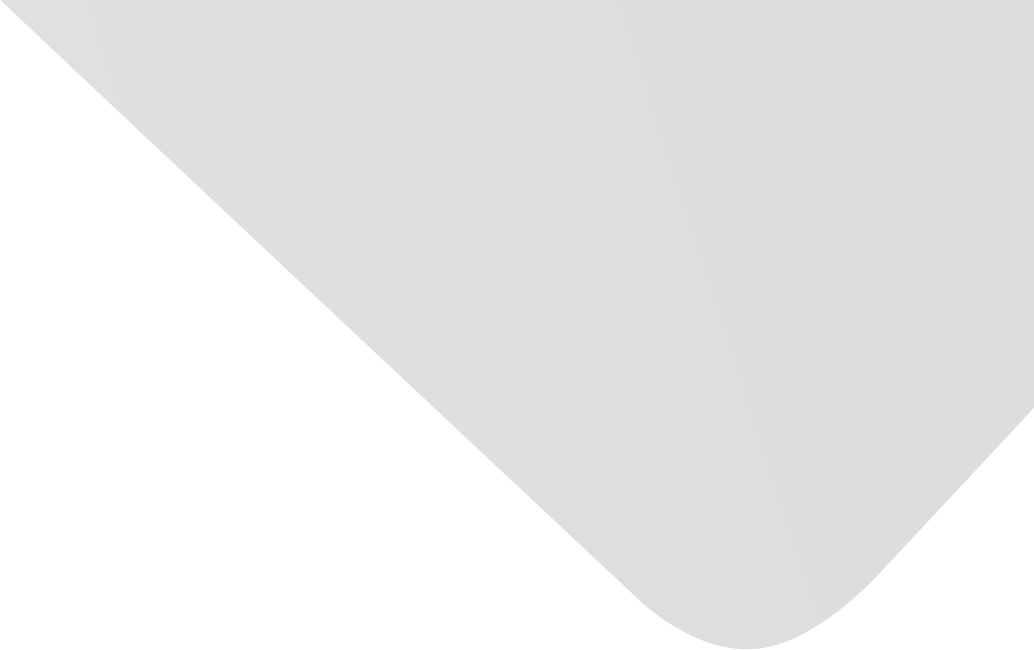
Comparison and Optimization of Neural Networks and Network Ensembles for Gap Filling of Wind Energy Data
Joint Authors
Schmidt, Andres
Suchaneck, Maya
Source
Issue
Vol. 2014, Issue 2014 (31 Dec. 2014), pp.1-15, 15 p.
Publisher
Hindawi Publishing Corporation
Publication Date
2014-05-26
Country of Publication
Egypt
No. of Pages
15
Main Subjects
Abstract EN
Wind turbines play an important role in providing electrical energy for an ever-growing demand.
Due to climate change driven by anthropogenic emissions of greenhouse gases, the exploration and use of sustainable energy sources is essential with wind energy covering a significant portion.
Data of existing wind turbines is needed to reduce the uncertainty of model predictions of future energy yields for planned wind farms.
Due to maintenance routines and technical issues, data gaps of reference wind parks are unavoidable.
Here, we present real-world case studies using multilayer perceptron networks and radial basis function networks to reproduce electrical energy outputs of wind turbines at 3 different locations in Germany covering a range of landscapes with varying topographic complexity.
The results show that the energy output values of the turbines could be modeled with high correlations ranging from 0.90 to 0.99.
In complex terrain, the RBF networks outperformed the MLP networks.
In addition, rare extreme values were better captured by the RBF networks in most cases.
By using wind meteorological variables and operating data recorded by the wind turbines in addition to the daily energy output values, the error could be further reduced to more than 20%.
American Psychological Association (APA)
Schmidt, Andres& Suchaneck, Maya. 2014. Comparison and Optimization of Neural Networks and Network Ensembles for Gap Filling of Wind Energy Data. Journal of Renewable Energy،Vol. 2014, no. 2014, pp.1-15.
https://search.emarefa.net/detail/BIM-1042906
Modern Language Association (MLA)
Schmidt, Andres& Suchaneck, Maya. Comparison and Optimization of Neural Networks and Network Ensembles for Gap Filling of Wind Energy Data. Journal of Renewable Energy No. 2014 (2014), pp.1-15.
https://search.emarefa.net/detail/BIM-1042906
American Medical Association (AMA)
Schmidt, Andres& Suchaneck, Maya. Comparison and Optimization of Neural Networks and Network Ensembles for Gap Filling of Wind Energy Data. Journal of Renewable Energy. 2014. Vol. 2014, no. 2014, pp.1-15.
https://search.emarefa.net/detail/BIM-1042906
Data Type
Journal Articles
Language
English
Notes
Includes bibliographical references
Record ID
BIM-1042906