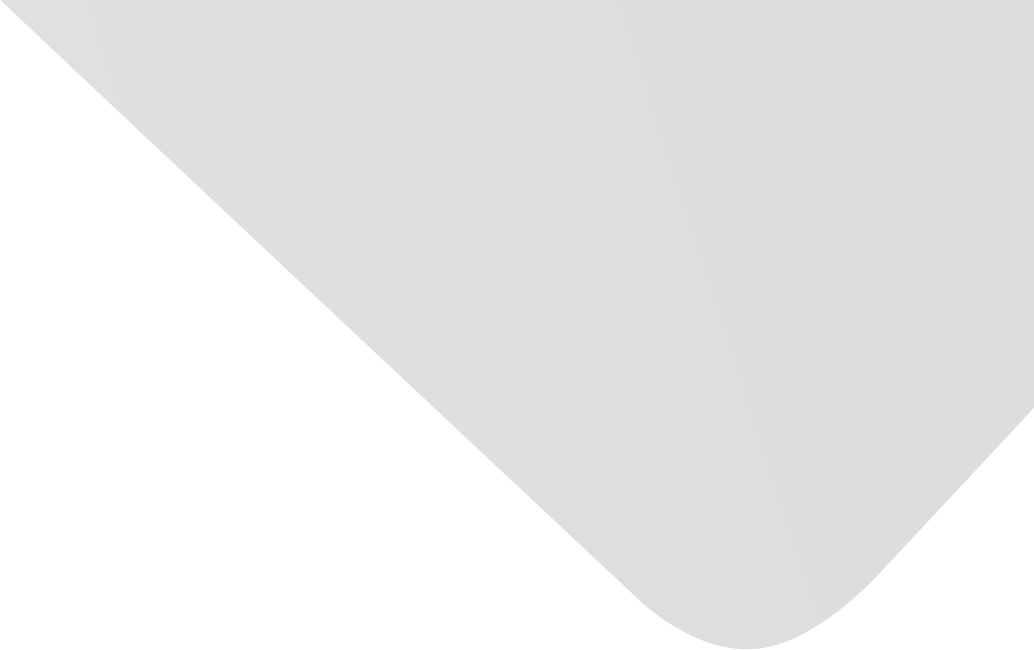
A Stochastic Restricted Principal Components Regression Estimator in the Linear Model
Joint Authors
Source
Issue
Vol. 2014, Issue 2014 (31 Dec. 2014), pp.1-6, 6 p.
Publisher
Hindawi Publishing Corporation
Publication Date
2014-01-23
Country of Publication
Egypt
No. of Pages
6
Main Subjects
Medicine
Information Technology and Computer Science
Abstract EN
We propose a new estimator to combat the multicollinearity in the linear model when there are stochastic linear restrictions on the regression coefficients.
The new estimator is constructed by combining the ordinary mixed estimator (OME) and the principal components regression (PCR) estimator, which is called the stochastic restricted principal components (SRPC) regression estimator.
Necessary and sufficient conditions for the superiority of the SRPC estimator over the OME and the PCR estimator are derived in the sense of the mean squared error matrix criterion.
Finally, we give a numerical example and a Monte Carlo study to illustrate the performance of the proposed estimator.
American Psychological Association (APA)
He, Daojiang& Wu, Yan. 2014. A Stochastic Restricted Principal Components Regression Estimator in the Linear Model. The Scientific World Journal،Vol. 2014, no. 2014, pp.1-6.
https://search.emarefa.net/detail/BIM-1048807
Modern Language Association (MLA)
He, Daojiang& Wu, Yan. A Stochastic Restricted Principal Components Regression Estimator in the Linear Model. The Scientific World Journal No. 2014 (2014), pp.1-6.
https://search.emarefa.net/detail/BIM-1048807
American Medical Association (AMA)
He, Daojiang& Wu, Yan. A Stochastic Restricted Principal Components Regression Estimator in the Linear Model. The Scientific World Journal. 2014. Vol. 2014, no. 2014, pp.1-6.
https://search.emarefa.net/detail/BIM-1048807
Data Type
Journal Articles
Language
English
Notes
Includes bibliographical references
Record ID
BIM-1048807