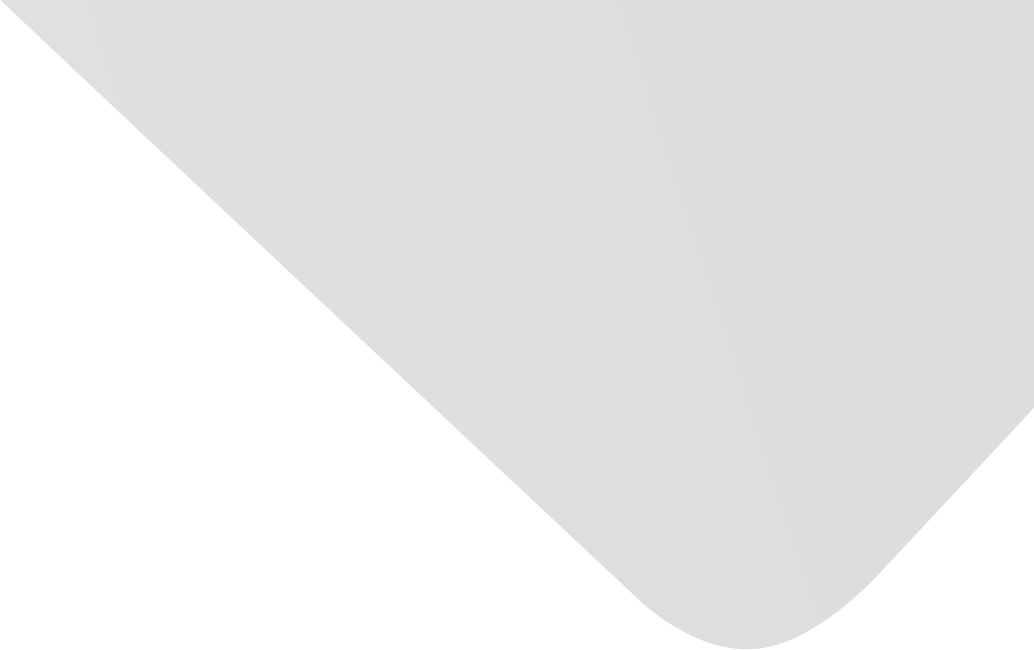
Prediction of Protein-Protein Interaction Strength Using Domain Features with Supervised Regression
Joint Authors
Hayashida, Morihiro
Kamada, Mayumi
Sakuma, Yusuke
Akutsu, Tatsuya
Source
Issue
Vol. 2014, Issue 2014 (31 Dec. 2014), pp.1-7, 7 p.
Publisher
Hindawi Publishing Corporation
Publication Date
2014-06-24
Country of Publication
Egypt
No. of Pages
7
Main Subjects
Medicine
Information Technology and Computer Science
Abstract EN
Proteins in living organisms express various important functions by interacting with other proteins and molecules.
Therefore, many efforts have been made to investigate and predict protein-protein interactions (PPIs).
Analysis of strengths of PPIs is also important because such strengths are involved in functionality of proteins.
In this paper, we propose several feature space mappings from protein pairs using protein domain information to predict strengths of PPIs.
Moreover, we perform computational experiments employing two machine learning methods, support vector regression (SVR) and relevance vector machine (RVM), for dataset obtained from biological experiments.
The prediction results showed that both SVR and RVM with our proposed features outperformed the best existing method.
American Psychological Association (APA)
Kamada, Mayumi& Sakuma, Yusuke& Hayashida, Morihiro& Akutsu, Tatsuya. 2014. Prediction of Protein-Protein Interaction Strength Using Domain Features with Supervised Regression. The Scientific World Journal،Vol. 2014, no. 2014, pp.1-7.
https://search.emarefa.net/detail/BIM-1048854
Modern Language Association (MLA)
Kamada, Mayumi…[et al.]. Prediction of Protein-Protein Interaction Strength Using Domain Features with Supervised Regression. The Scientific World Journal No. 2014 (2014), pp.1-7.
https://search.emarefa.net/detail/BIM-1048854
American Medical Association (AMA)
Kamada, Mayumi& Sakuma, Yusuke& Hayashida, Morihiro& Akutsu, Tatsuya. Prediction of Protein-Protein Interaction Strength Using Domain Features with Supervised Regression. The Scientific World Journal. 2014. Vol. 2014, no. 2014, pp.1-7.
https://search.emarefa.net/detail/BIM-1048854
Data Type
Journal Articles
Language
English
Notes
Includes bibliographical references
Record ID
BIM-1048854