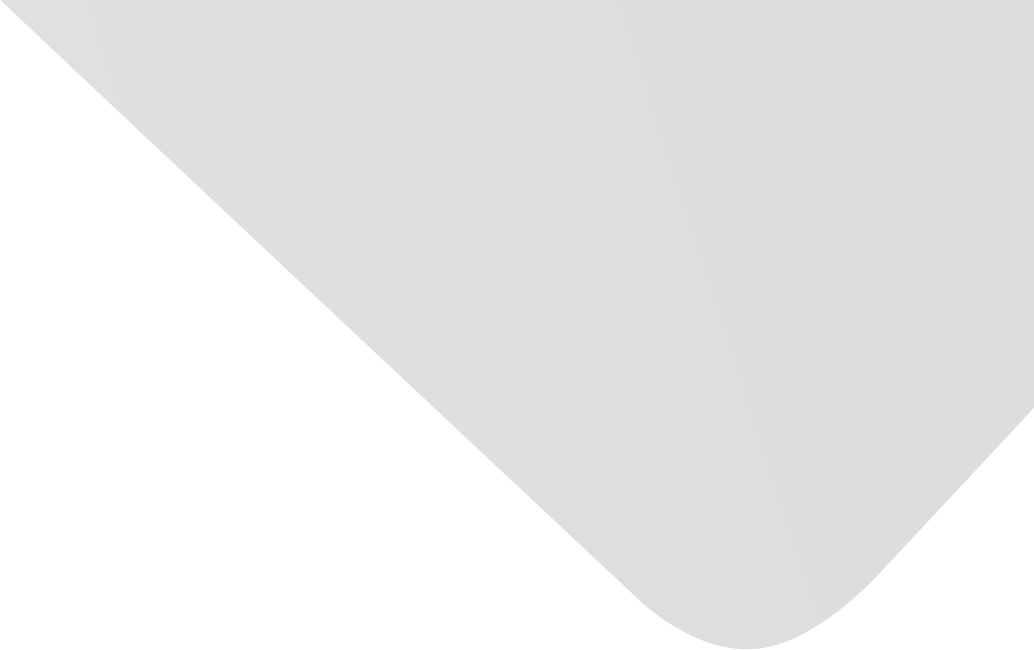
Instance Transfer Learning with Multisource Dynamic TrAdaBoost
Joint Authors
Zhang, Qian
Li, Haigang
Zhang, Yong
Li, Ming
Source
Issue
Vol. 2014, Issue 2014 (31 Dec. 2014), pp.1-8, 8 p.
Publisher
Hindawi Publishing Corporation
Publication Date
2014-07-24
Country of Publication
Egypt
No. of Pages
8
Main Subjects
Medicine
Information Technology and Computer Science
Abstract EN
Since the transfer learning can employ knowledge in relative domains to help the learning tasks in current target domain, compared with the traditional learning it shows the advantages of reducing the learning cost and improving the learning efficiency.
Focused on the situation that sample data from the transfer source domain and the target domain have similar distribution, an instance transfer learning method based on multisource dynamic TrAdaBoost is proposed in this paper.
In this method, knowledge from multiple source domains is used well to avoid negative transfer; furthermore, the information that is conducive to target task learning is obtained to train candidate classifiers.
The theoretical analysis suggests that the proposed algorithm improves the capability that weight entropy drifts from source to target instances by means of adding the dynamic factor, and the classification effectiveness is better than single source transfer.
Finally, experimental results show that the proposed algorithm has higher classification accuracy.
American Psychological Association (APA)
Zhang, Qian& Li, Haigang& Zhang, Yong& Li, Ming. 2014. Instance Transfer Learning with Multisource Dynamic TrAdaBoost. The Scientific World Journal،Vol. 2014, no. 2014, pp.1-8.
https://search.emarefa.net/detail/BIM-1049062
Modern Language Association (MLA)
Zhang, Qian…[et al.]. Instance Transfer Learning with Multisource Dynamic TrAdaBoost. The Scientific World Journal No. 2014 (2014), pp.1-8.
https://search.emarefa.net/detail/BIM-1049062
American Medical Association (AMA)
Zhang, Qian& Li, Haigang& Zhang, Yong& Li, Ming. Instance Transfer Learning with Multisource Dynamic TrAdaBoost. The Scientific World Journal. 2014. Vol. 2014, no. 2014, pp.1-8.
https://search.emarefa.net/detail/BIM-1049062
Data Type
Journal Articles
Language
English
Notes
Includes bibliographical references
Record ID
BIM-1049062