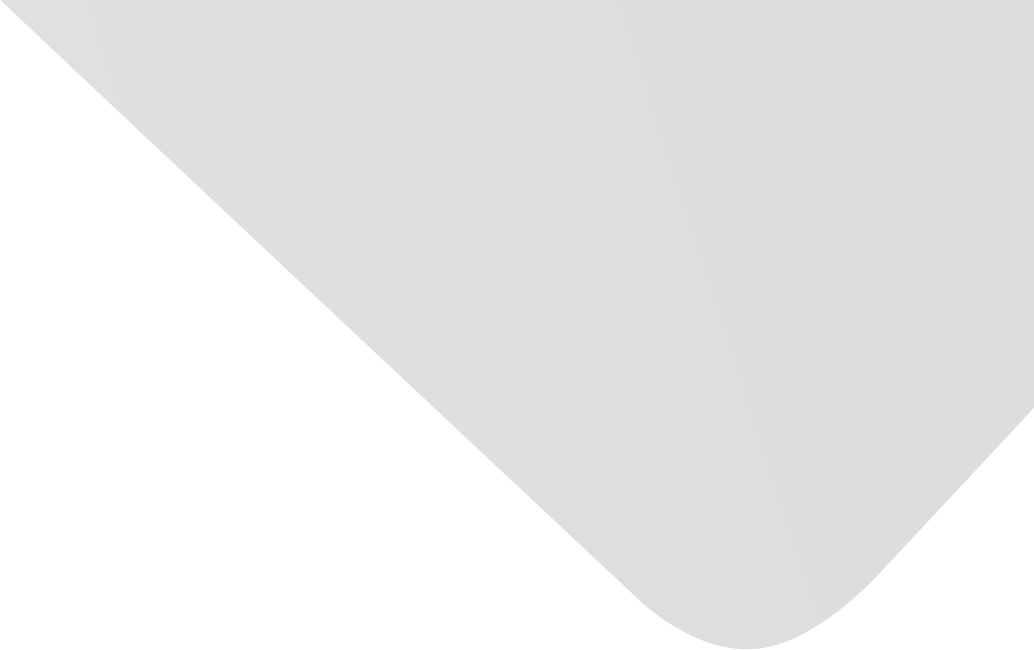
Robust Framework to Combine Diverse Classifiers Assigning Distributed Confidence to Individual Classifiers at Class Level
Joint Authors
Jabbar, Sohail
Arshad, Sannia
Rho, Seungmin
Khalid, Shehzad
Source
Issue
Vol. 2014, Issue 2014 (31 Dec. 2014), pp.1-14, 14 p.
Publisher
Hindawi Publishing Corporation
Publication Date
2014-09-08
Country of Publication
Egypt
No. of Pages
14
Main Subjects
Medicine
Information Technology and Computer Science
Abstract EN
We have presented a classification framework that combines multiple heterogeneous classifiers in the presence of class label noise.
An extension of m-Mediods based modeling is presented that generates model of various classes whilst identifying and filtering noisy training data.
This noise free data is further used to learn model for other classifiers such as GMM and SVM.
A weight learning method is then introduced to learn weights on each class for different classifiers to construct an ensemble.
For this purpose, we applied genetic algorithm to search for an optimal weight vector on which classifier ensemble is expected to give the best accuracy.
The proposed approach is evaluated on variety of real life datasets.
It is also compared with existing standard ensemble techniques such as Adaboost, Bagging, and Random Subspace Methods.
Experimental results show the superiority of proposed ensemble method as compared to its competitors, especially in the presence of class label noise and imbalance classes.
American Psychological Association (APA)
Khalid, Shehzad& Arshad, Sannia& Jabbar, Sohail& Rho, Seungmin. 2014. Robust Framework to Combine Diverse Classifiers Assigning Distributed Confidence to Individual Classifiers at Class Level. The Scientific World Journal،Vol. 2014, no. 2014, pp.1-14.
https://search.emarefa.net/detail/BIM-1049825
Modern Language Association (MLA)
Khalid, Shehzad…[et al.]. Robust Framework to Combine Diverse Classifiers Assigning Distributed Confidence to Individual Classifiers at Class Level. The Scientific World Journal No. 2014 (2014), pp.1-14.
https://search.emarefa.net/detail/BIM-1049825
American Medical Association (AMA)
Khalid, Shehzad& Arshad, Sannia& Jabbar, Sohail& Rho, Seungmin. Robust Framework to Combine Diverse Classifiers Assigning Distributed Confidence to Individual Classifiers at Class Level. The Scientific World Journal. 2014. Vol. 2014, no. 2014, pp.1-14.
https://search.emarefa.net/detail/BIM-1049825
Data Type
Journal Articles
Language
English
Notes
Includes bibliographical references
Record ID
BIM-1049825