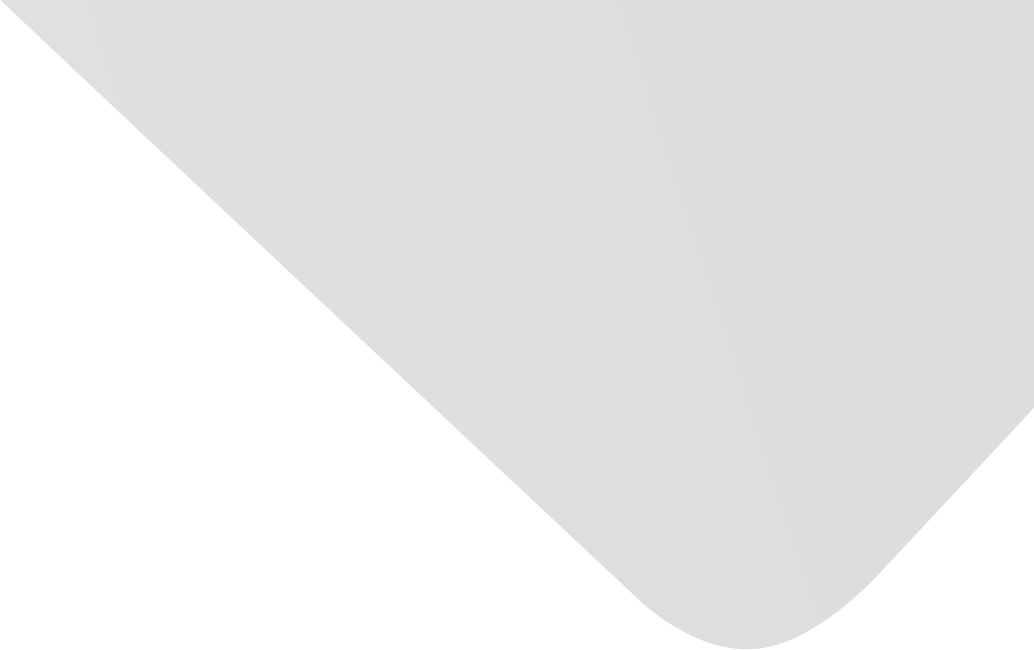
Damage Identification for Large Span Structure Based on Multiscale Inputs to Artificial Neural Networks
Joint Authors
Source
Issue
Vol. 2014, Issue 2014 (31 Dec. 2014), pp.1-12, 12 p.
Publisher
Hindawi Publishing Corporation
Publication Date
2014-05-25
Country of Publication
Egypt
No. of Pages
12
Main Subjects
Medicine
Information Technology and Computer Science
Abstract EN
In structural health monitoring system, little research on the damage identification from different types of sensors applied to large span structure has been done in the field.
In fact, it is significant to estimate the whole structural safety if the multitype sensors or multiscale measurements are used in application of structural health monitoring and the damage identification for large span structure.
A methodology to combine the local and global measurements in noisy environments based on artificial neural network is proposed in this paper.
For a real large span structure, the capacity of the methodology is validated, including the decision on damage placement, the discussions on the number of the sensors, and the optimal parameters for artificial neural networks.
Furthermore, the noisy environments in different levels are simulated to demonstrate the robustness and effectiveness of the proposed approach.
American Psychological Association (APA)
Lu, Wei& Teng, Jun& Cui, Yan. 2014. Damage Identification for Large Span Structure Based on Multiscale Inputs to Artificial Neural Networks. The Scientific World Journal،Vol. 2014, no. 2014, pp.1-12.
https://search.emarefa.net/detail/BIM-1050050
Modern Language Association (MLA)
Lu, Wei…[et al.]. Damage Identification for Large Span Structure Based on Multiscale Inputs to Artificial Neural Networks. The Scientific World Journal No. 2014 (2014), pp.1-12.
https://search.emarefa.net/detail/BIM-1050050
American Medical Association (AMA)
Lu, Wei& Teng, Jun& Cui, Yan. Damage Identification for Large Span Structure Based on Multiscale Inputs to Artificial Neural Networks. The Scientific World Journal. 2014. Vol. 2014, no. 2014, pp.1-12.
https://search.emarefa.net/detail/BIM-1050050
Data Type
Journal Articles
Language
English
Notes
Includes bibliographical references
Record ID
BIM-1050050