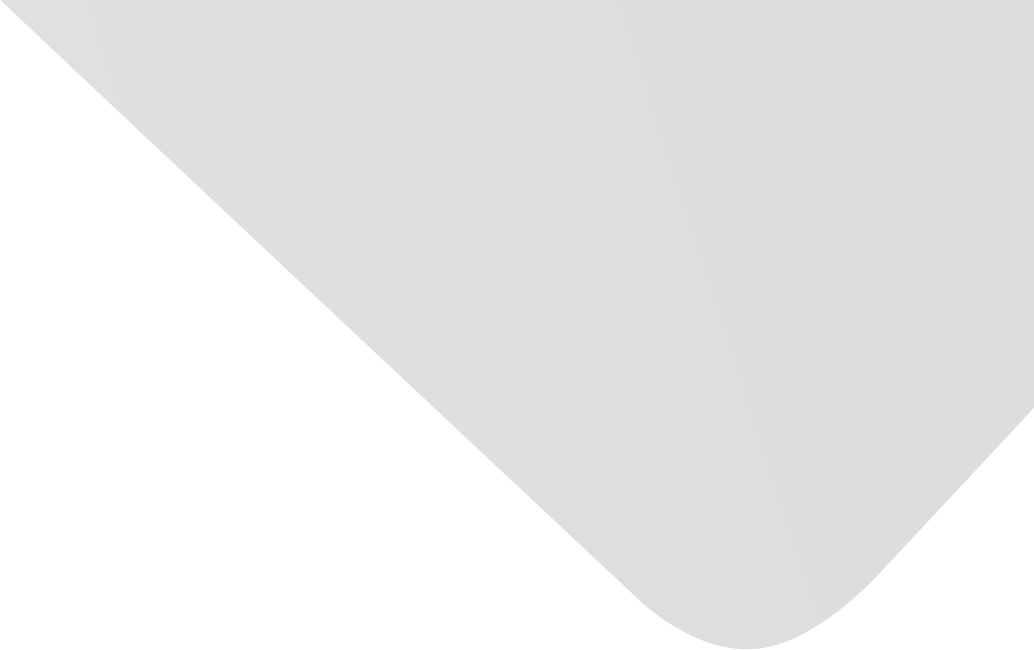
Improving Causality Induction with Category Learning
Joint Authors
Shao, Zhiqing
Guo, Yi
Wang, Zhihong
Source
Issue
Vol. 2014, Issue 2014 (31 Dec. 2014), pp.1-8, 8 p.
Publisher
Hindawi Publishing Corporation
Publication Date
2014-04-30
Country of Publication
Egypt
No. of Pages
8
Main Subjects
Medicine
Information Technology and Computer Science
Abstract EN
Causal relations are of fundamental importance for human perception and reasoning.
According to the nature of causality, causality has explicit and implicit forms.
In the case of explicit form, causal-effect relations exist at either clausal or discourse levels.
The implicit causal-effect relations heavily rely on empirical analysis and evidence accumulation.
This paper proposes a comprehensive causality extraction system (CL-CIS) integrated with the means of category-learning.
CL-CIS considers cause-effect relations in both explicit and implicit forms and especially practices the relation between category and causality in computation.
In elaborately designed experiments, CL-CIS is evaluated together with general causality analysis system (GCAS) and general causality analysis system with learning (GCAS-L), and it testified to its own capability and performance in construction of cause-effect relations.
This paper confirms the expectation that the precision and coverage of causality induction can be remarkably improved by means of causal and category learning.
American Psychological Association (APA)
Guo, Yi& Wang, Zhihong& Shao, Zhiqing. 2014. Improving Causality Induction with Category Learning. The Scientific World Journal،Vol. 2014, no. 2014, pp.1-8.
https://search.emarefa.net/detail/BIM-1050506
Modern Language Association (MLA)
Guo, Yi…[et al.]. Improving Causality Induction with Category Learning. The Scientific World Journal No. 2014 (2014), pp.1-8.
https://search.emarefa.net/detail/BIM-1050506
American Medical Association (AMA)
Guo, Yi& Wang, Zhihong& Shao, Zhiqing. Improving Causality Induction with Category Learning. The Scientific World Journal. 2014. Vol. 2014, no. 2014, pp.1-8.
https://search.emarefa.net/detail/BIM-1050506
Data Type
Journal Articles
Language
English
Notes
Includes bibliographical references
Record ID
BIM-1050506